Generative Neural Fields by Mixtures of Neural Implicit Functions
NeurIPS(2023)
摘要
We propose a novel approach to learning the generative neural fields
represented by linear combinations of implicit basis networks. Our algorithm
learns basis networks in the form of implicit neural representations and their
coefficients in a latent space by either conducting meta-learning or adopting
auto-decoding paradigms. The proposed method easily enlarges the capacity of
generative neural fields by increasing the number of basis networks while
maintaining the size of a network for inference to be small through their
weighted model averaging. Consequently, sampling instances using the model is
efficient in terms of latency and memory footprint. Moreover, we customize
denoising diffusion probabilistic model for a target task to sample latent
mixture coefficients, which allows our final model to generate unseen data
effectively. Experiments show that our approach achieves competitive generation
performance on diverse benchmarks for images, voxel data, and NeRF scenes
without sophisticated designs for specific modalities and domains.
更多查看译文
关键词
neural implicit functions,generative,fields,mixtures
AI 理解论文
溯源树
样例
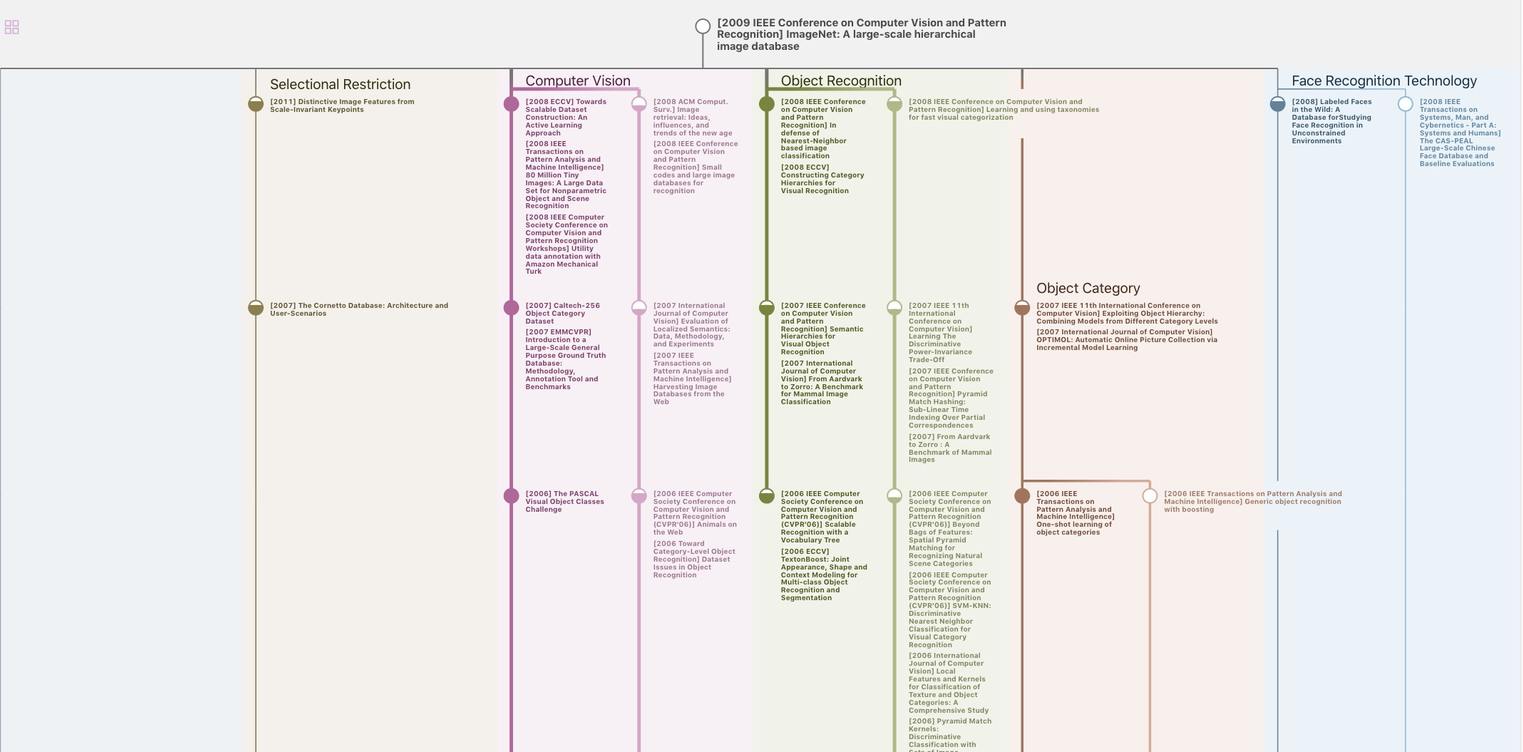
生成溯源树,研究论文发展脉络
Chat Paper
正在生成论文摘要