Optimize Planning Heuristics to Rank, not to Estimate Cost-to-Goal
arXiv (Cornell University)(2023)
摘要
In imitation learning for planning, parameters of heuristic functions are
optimized against a set of solved problem instances. This work revisits the
necessary and sufficient conditions of strictly optimally efficient heuristics
for forward search algorithms, mainly A* and greedy best-first search, which
expand only states on the returned optimal path. It then proposes a family of
loss functions based on ranking tailored for a given variant of the forward
search algorithm. Furthermore, from a learning theory point of view, it
discusses why optimizing cost-to-goal \hstar\ is unnecessarily difficult. The
experimental comparison on a diverse set of problems unequivocally supports the
derived theory.
更多查看译文
关键词
heuristics,planning,rank,cost-to-goal
AI 理解论文
溯源树
样例
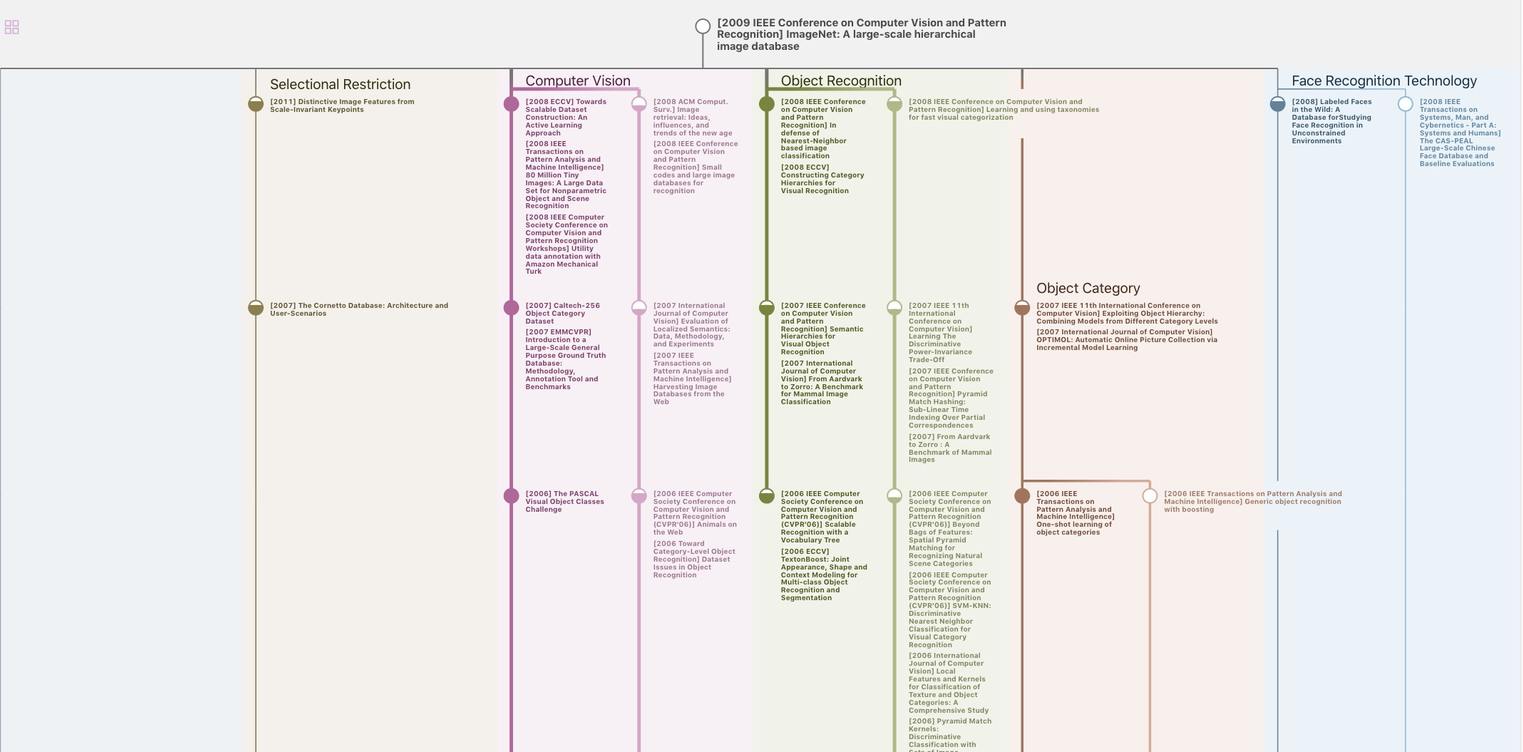
生成溯源树,研究论文发展脉络
Chat Paper
正在生成论文摘要