Generalized Category Discovery with Clustering Assignment Consistency
NEURAL INFORMATION PROCESSING, ICONIP 2023, PT V(2024)
摘要
Generalized category discovery (GCD) is an important openworld task to automatically cluster the unlabeled samples using information transferred from the labeled dataset, given a dataset consisting of labeled and unlabeled instances. A major challenge in GCD is that unlabeled novel class samples and unlabeled known class samples are mixed together in the unlabeled dataset. To conduct GCD without knowing the number of classes in unlabeled dataset, we propose a co-training-based framework that encourages clustering consistency. Specifically, we first introduce weak and strong augmentation transformations to generate two sufficiently different views for the same sample. Then, based on the co-training assumption, we propose a consistency representation learning strategy, which encourages consistency between feature-prototype similarity and clustering assignment. Finally, we use the discriminative embeddings learned from the semi-supervised representation learning process to construct an original sparse network and use a community detection method to obtain the clustering results and the number of categories simultaneously. Extensive experiments show that our method achieves state-of-the-art performance on three generic benchmarks and three fine-grained visual recognition datasets.
更多查看译文
关键词
Generalized category discovery,Open-world problem,Novel category discovery
AI 理解论文
溯源树
样例
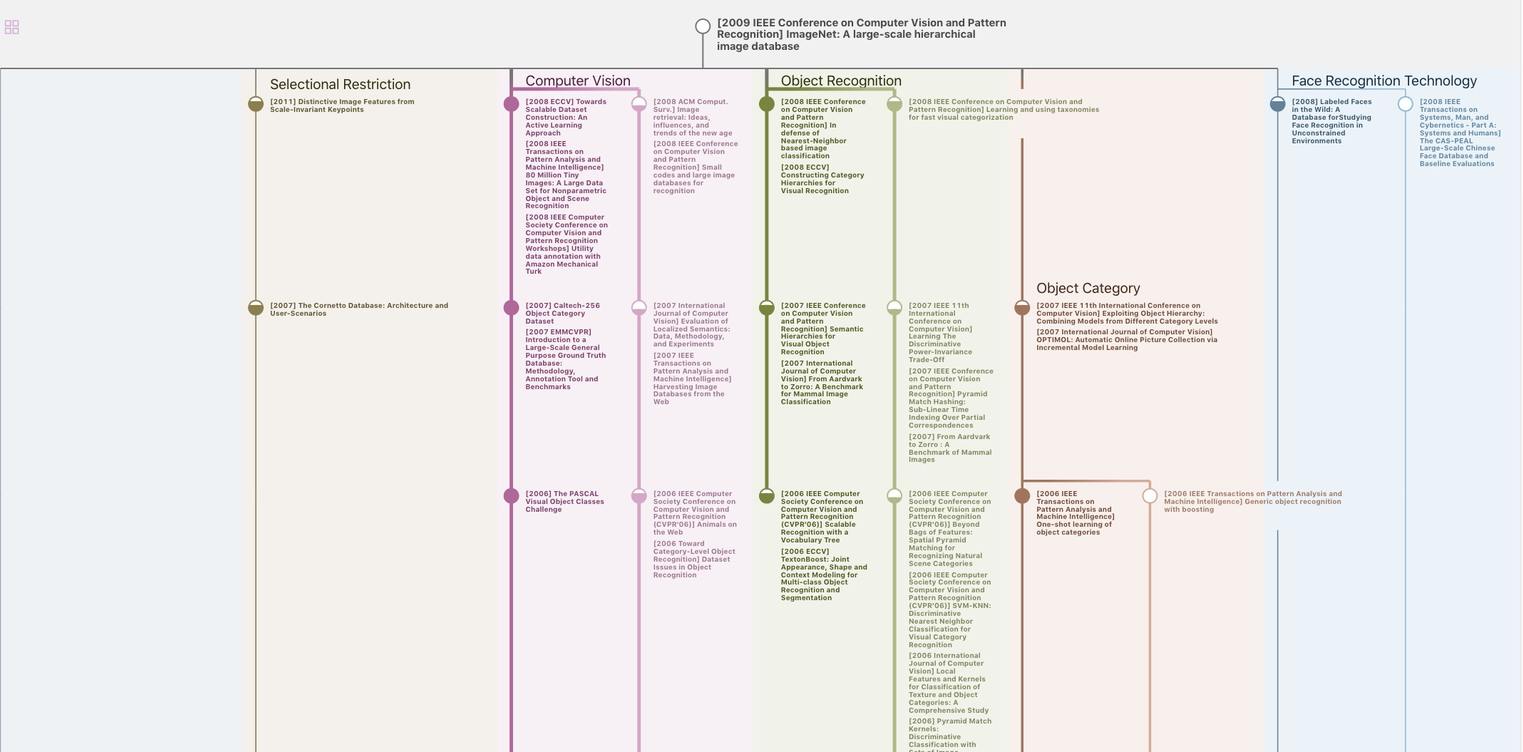
生成溯源树,研究论文发展脉络
Chat Paper
正在生成论文摘要