Efficient IoT Inference via Context-Awareness
CoRR(2023)
摘要
While existing strategies for optimizing deep learning-based classification
models on low-power platforms assume the models are trained on all classes of
interest, this paper posits that adopting context-awareness i.e. focusing
solely on the likely classes in the current context, can substantially enhance
performance in resource-constrained environments. We propose a new paradigm,
CACTUS, for scalable and efficient context-aware classification where a
micro-classifier recognizes a small set of classes relevant to the current
context and, when context change happens, rapidly switches to another suitable
micro-classifier. CACTUS has several innovations including optimizing the
training cost of context-aware classifiers, enabling on-the-fly context-aware
switching between classifiers, and selecting the best context-aware classifiers
given limited resources. We show that CACTUS achieves significant benefits in
accuracy, latency, and compute budget across a range of datasets and IoT
platforms.
更多查看译文
关键词
efficient iot inference,context-awareness
AI 理解论文
溯源树
样例
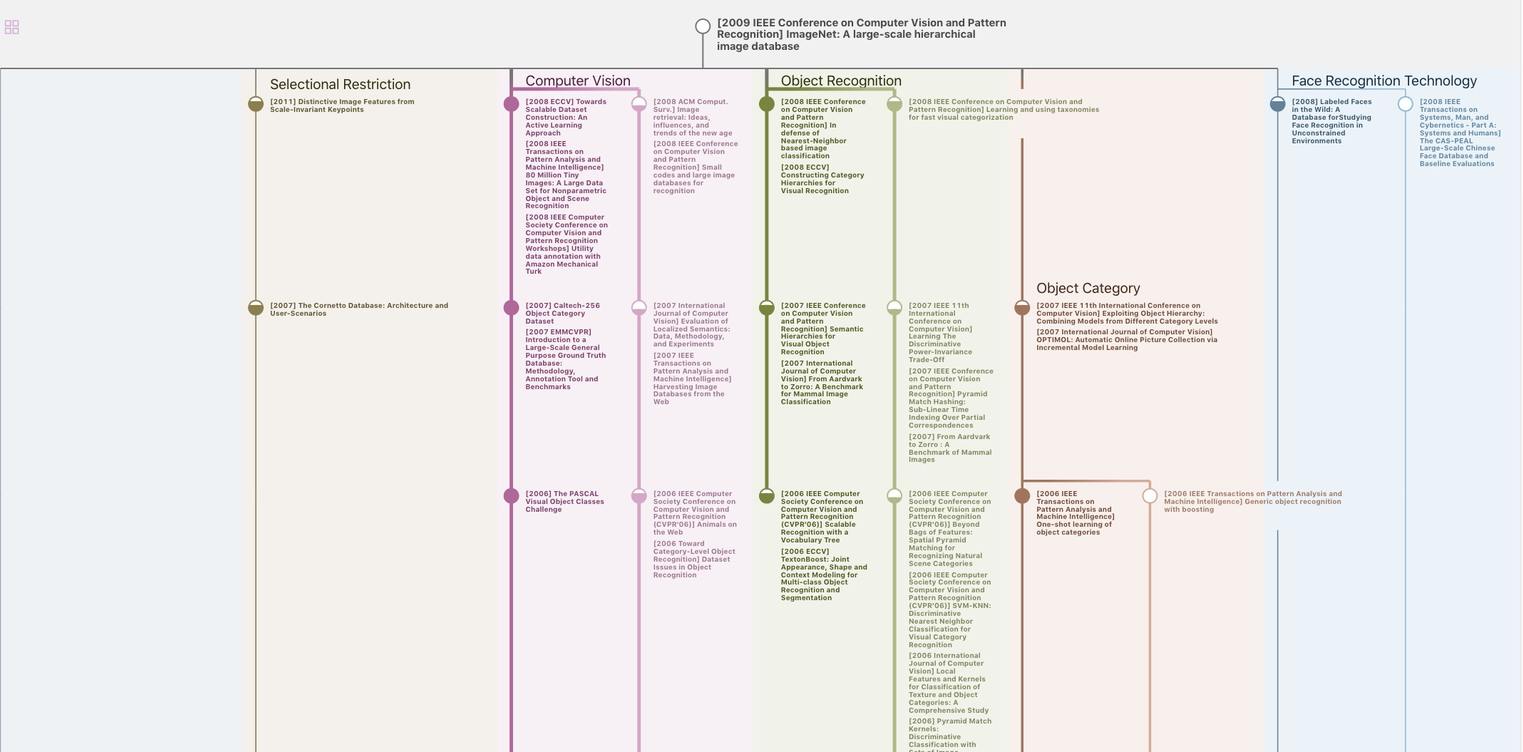
生成溯源树,研究论文发展脉络
Chat Paper
正在生成论文摘要