ViTMatte: Boosting image matting with pre-trained plain vision transformers
INFORMATION FUSION(2024)
摘要
Image matting is an inverse fusion process that separates the foreground and background information by predicting alpha matte for each pixel. Recently, plain vision Transformers (ViT) have shown impressive performance on various computer vision tasks, thanks to their strong modeling capacity and large-scale pre -training. However, they have not yet conquered the problem of image matting. We hypothesize that image matting could also be boosted by ViT and present a new efficient and robust ViT-based matting system, named ViTMatte. Our method utilizes (i) a hybrid attention mechanism combined with a convolution neck to help ViT achieve an excellent performance-computation trade-off in matting tasks. (ii) Additionally, we introduce the detail capture module, which just consists of simple lightweight convolutions to complement the detailed information required by matting. To the best of our knowledge, ViTMatte is the first work to unleash the potential of ViT on image matting with concise adaptation. It inherits many superior properties from ViT to matting, including various pre-training strategies, concise architecture design, and flexible inference strategies. We evaluate ViTMatte on Composition-1k and Distinctions-646, the most commonly used benchmark for image matting, our method achieves state-of-the-art performance and outperforms prior matting works by a large margin. Our codes and models are available at: https://github.com/hustvl/ViTMatte.
更多查看译文
关键词
Image matting,Vision transformer,Pre-training,Deep learning
AI 理解论文
溯源树
样例
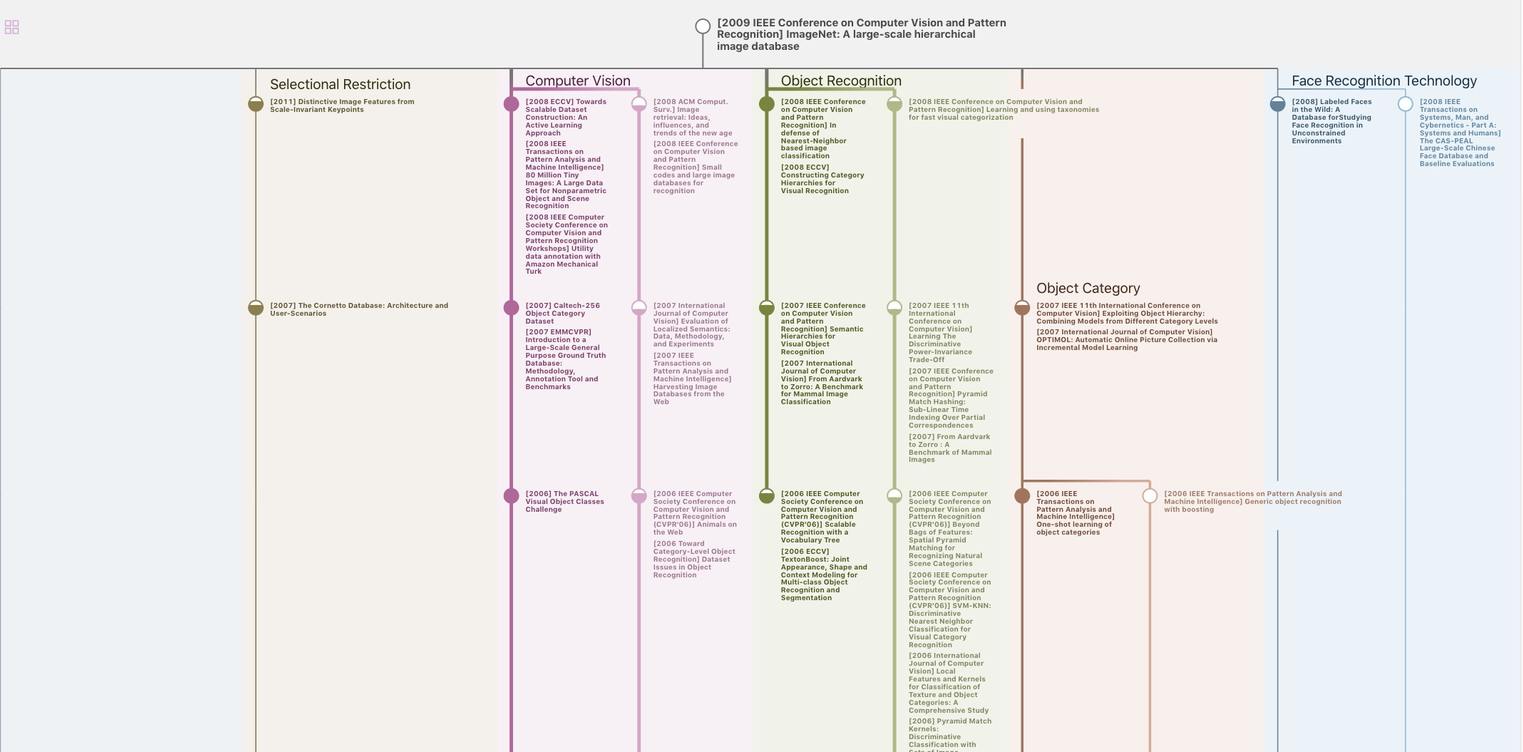
生成溯源树,研究论文发展脉络
Chat Paper
正在生成论文摘要