Probabilistic study of Induced Ordered Linear Fusion Operators for time series forecasting
INFORMATION FUSION(2024)
摘要
The aggregation of several predictors in time series forecasting has been used intensely in the last decade in order to construct a better resulting model. Some of the most used alternatives are the ones related to the Induced Ordered Weighted Averaging (IOWA), in which the prediction values are ordered using a secondary vector, often related to the accuracy of the prediction model in the last prediction. Although the time series study has been historically a subject related to statistics and stochastic processes, the random behaviour of the aggregation process is typically not considered. In addition, extensions of aggregation functions with a weaker notion of monotonicity, pre-aggregation functions, are appearing as better alternative for some topics such us classification. In this paper, a pre-aggregation extension of the IOWA operator, the Induced Ordered Linear Fusion (IOLF), is defined as a way to aggregate time series model predictions and its behaviour is studied from a probabilistic point of view. The IOLF operator over random vectors is defined, its properties studied and the relation between some averaging aggregation functions established. The expressions of the optimal weights according to statistical criteria are derived. The advantages and consequences of the use of the IOLF operator are studied, and its behaviour is compared to the usual procedures. Numerical results illustrate its performance on a practical example.
更多查看译文
关键词
Time series forecasting,Predictions fusion,Induced Ordered Weighted Averaging,Pre-aggregation functions
AI 理解论文
溯源树
样例
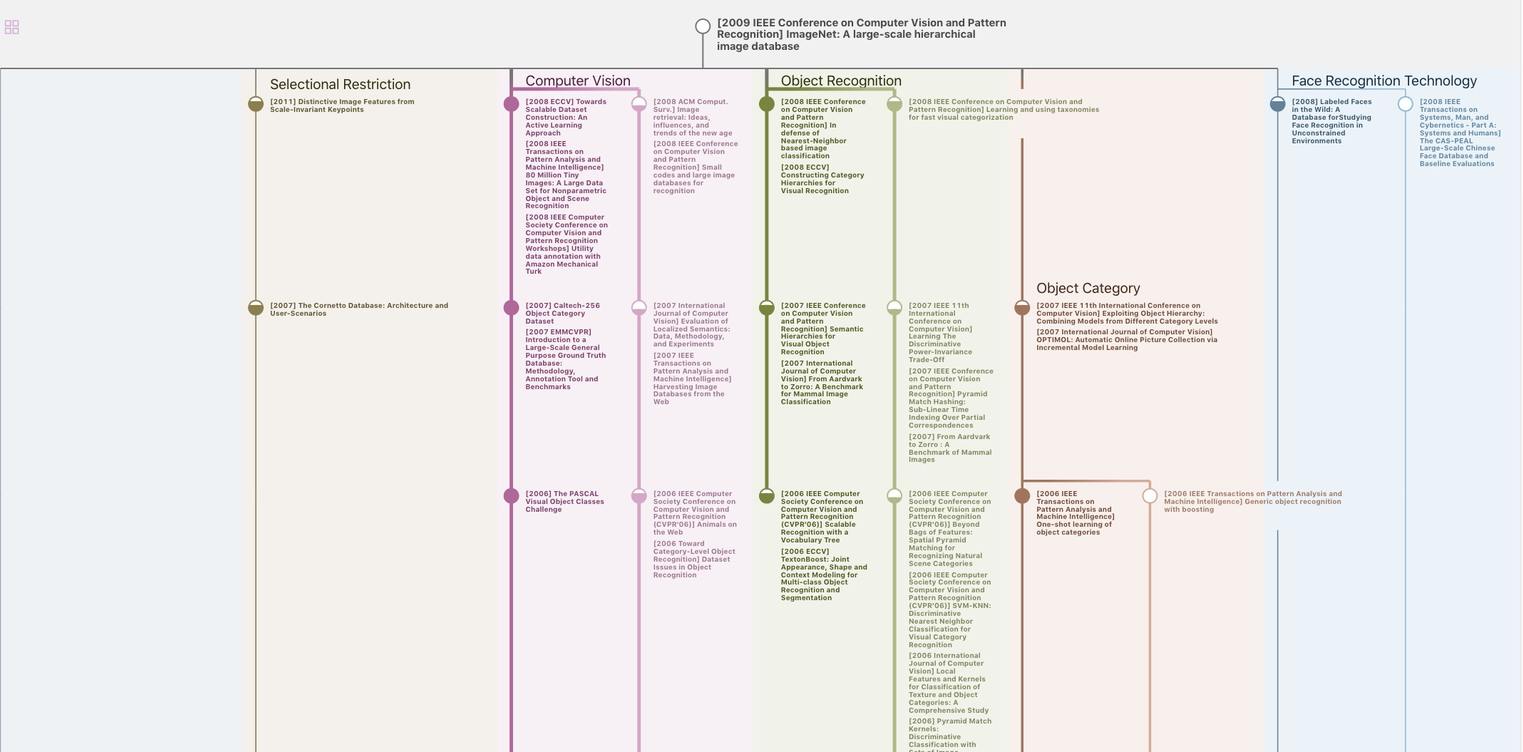
生成溯源树,研究论文发展脉络
Chat Paper
正在生成论文摘要