Improved Bayesian Regret Bounds for Thompson Sampling in Reinforcement Learning
NeurIPS(2023)
摘要
In this paper, we prove the first Bayesian regret bounds for Thompson
Sampling in reinforcement learning in a multitude of settings. We simplify the
learning problem using a discrete set of surrogate environments, and present a
refined analysis of the information ratio using posterior consistency. This
leads to an upper bound of order O(H√(d_l_1T)) in the time
inhomogeneous reinforcement learning problem where H is the episode length
and d_l_1 is the Kolmogorov l_1-dimension of the space of environments.
We then find concrete bounds of d_l_1 in a variety of settings, such as
tabular, linear and finite mixtures, and discuss how how our results are either
the first of their kind or improve the state-of-the-art.
更多查看译文
AI 理解论文
溯源树
样例
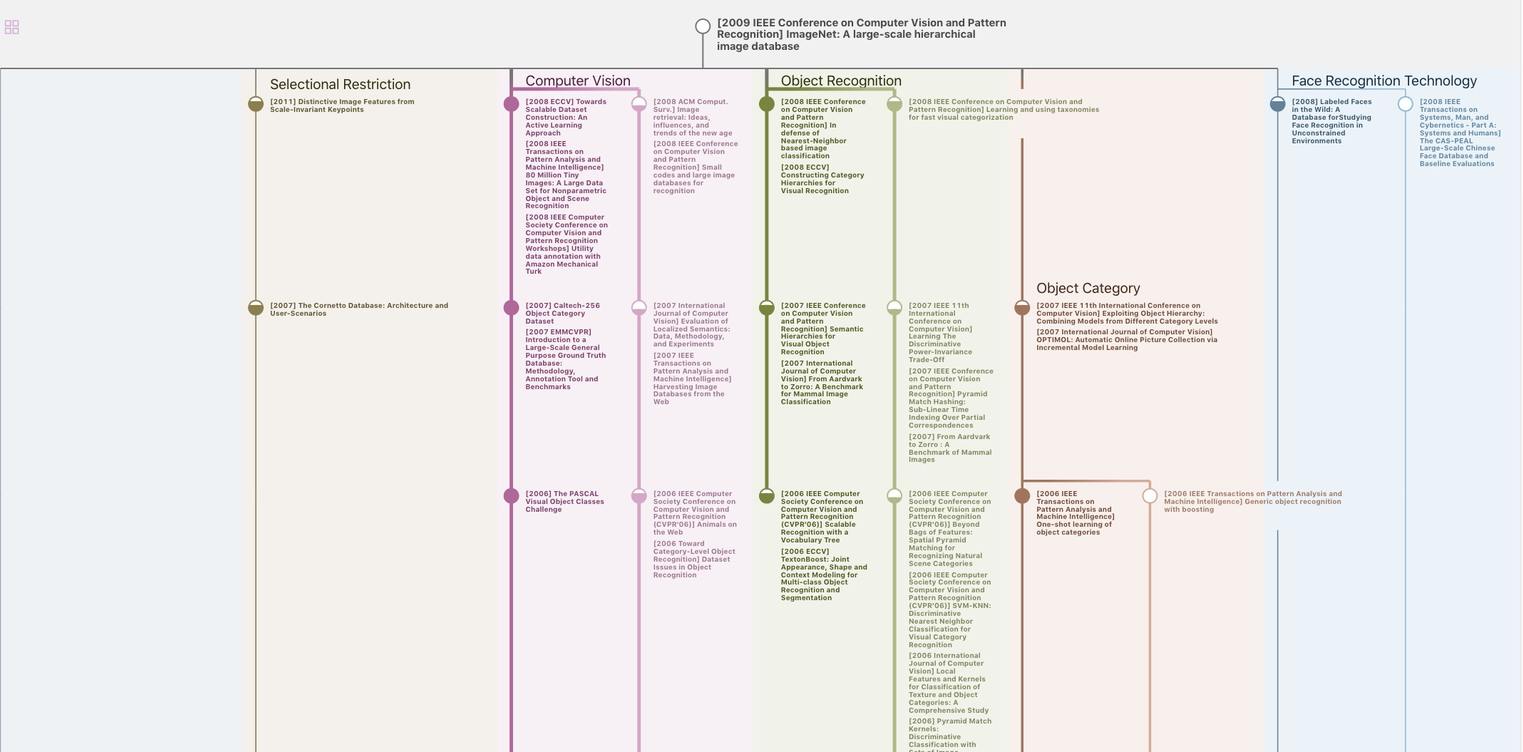
生成溯源树,研究论文发展脉络
Chat Paper
正在生成论文摘要