Online Conversion with Switching Costs: Robust and Learning-Augmented Algorithms
CoRR(2023)
摘要
We introduce and study online conversion with switching costs, a family of
online problems that capture emerging problems at the intersection of energy
and sustainability. In this problem, an online player attempts to purchase
(alternatively, sell) fractional shares of an asset during a fixed time horizon
with length T. At each time step, a cost function (alternatively, price
function) is revealed, and the player must irrevocably decide an amount of
asset to convert. The player also incurs a switching cost whenever their
decision changes in consecutive time steps, i.e., when they increase or
decrease their purchasing amount. We introduce competitive (robust)
threshold-based algorithms for both the minimization and maximization variants
of this problem, and show they are optimal among deterministic online
algorithms. We then propose learning-augmented algorithms that take advantage
of untrusted black-box advice (such as predictions from a machine learning
model) to achieve significantly better average-case performance without
sacrificing worst-case competitive guarantees. Finally, we empirically evaluate
our proposed algorithms using a carbon-aware EV charging case study, showing
that our algorithms substantially improve on baseline methods for this problem.
更多查看译文
关键词
conversion,switching,costs,learning-augmented
AI 理解论文
溯源树
样例
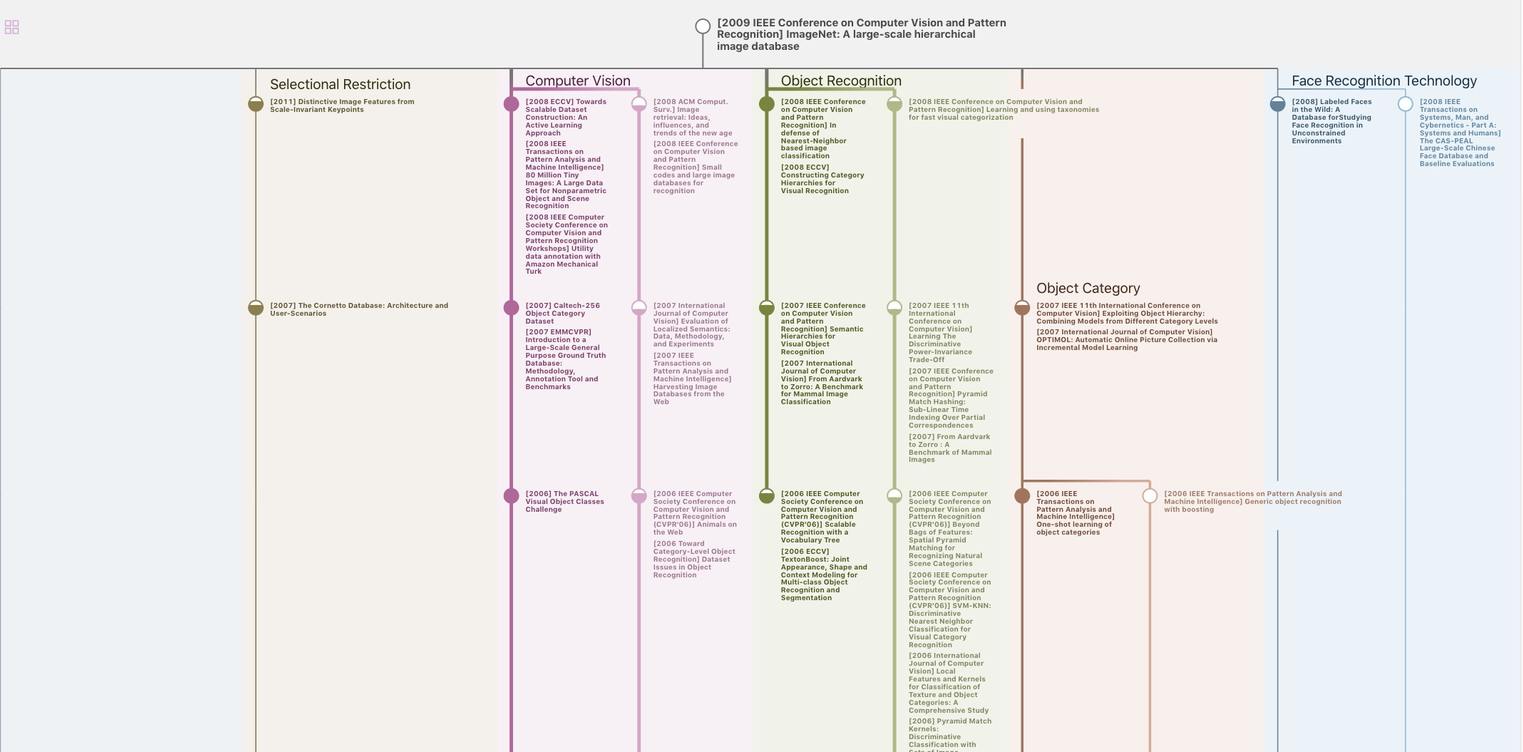
生成溯源树,研究论文发展脉络
Chat Paper
正在生成论文摘要