Learning to Play Chess from Textbooks (LEAP): a Corpus for Evaluating Chess Moves based on Sentiment Analysis
CoRR(2023)
摘要
Learning chess strategies has been investigated widely, with most studies focussing on learning from previous games using search algorithms. Chess textbooks encapsulate grandmaster knowledge, explain playing strategies and require a smaller search space compared to traditional chess agents. This paper examines chess textbooks as a new knowledge source for enabling machines to learn how to play chess -- a resource that has not been explored previously. We developed the LEAP corpus, a first and new heterogeneous dataset with structured (chess move notations and board states) and unstructured data (textual descriptions) collected from a chess textbook containing 1164 sentences discussing strategic moves from 91 games. We firstly labelled the sentences based on their relevance, i.e., whether they are discussing a move. Each relevant sentence was then labelled according to its sentiment towards the described move. We performed empirical experiments that assess the performance of various transformer-based baseline models for sentiment analysis. Our results demonstrate the feasibility of employing transformer-based sentiment analysis models for evaluating chess moves, with the best performing model obtaining a weighted micro F_1 score of 68%. Finally, we synthesised the LEAP corpus to create a larger dataset, which can be used as a solution to the limited textual resource in the chess domain.
更多查看译文
关键词
chess moves,textbooks,corpus,learning
AI 理解论文
溯源树
样例
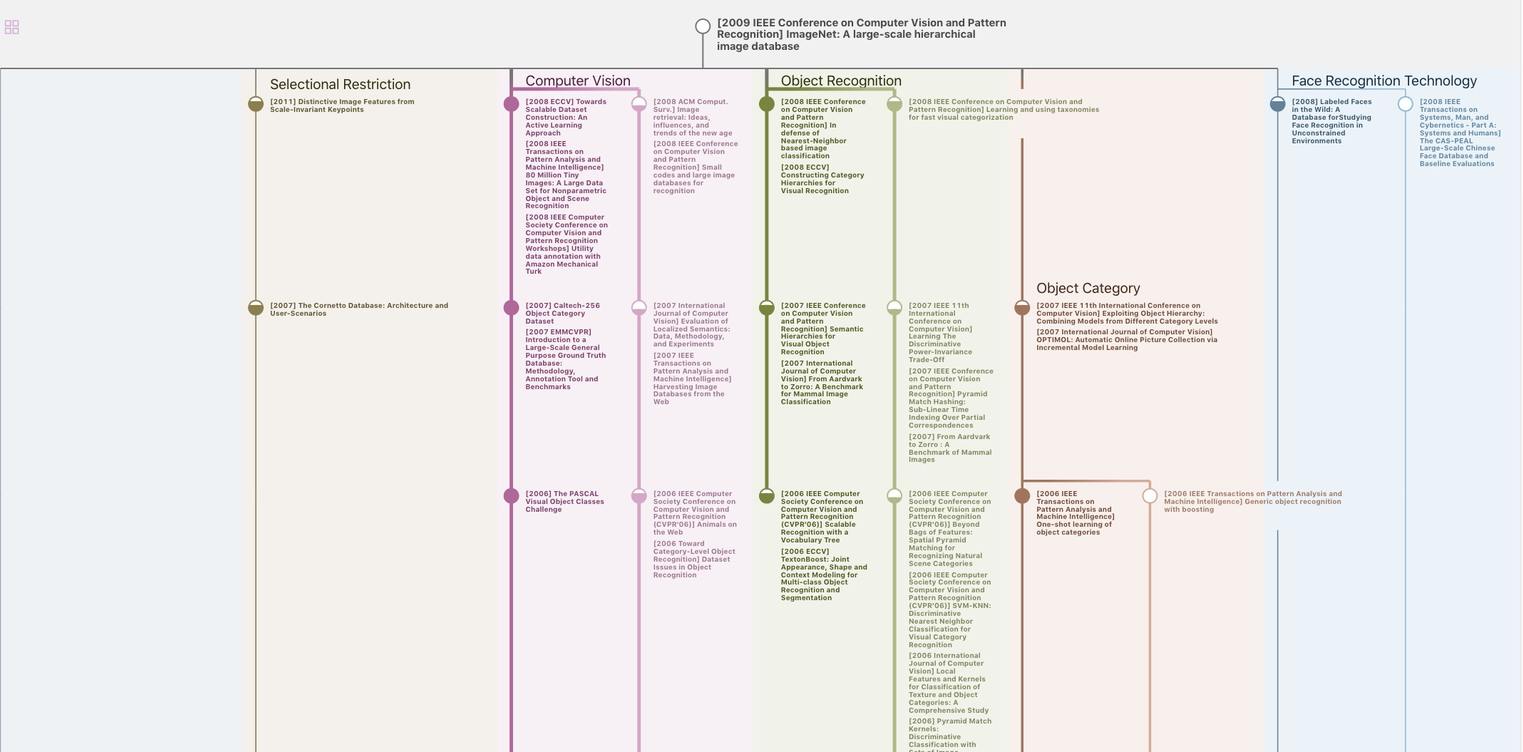
生成溯源树,研究论文发展脉络
Chat Paper
正在生成论文摘要