Unveiling the Limits of Learned Local Search Heuristics: Are You the Mightiest of the Meek?
CoRR(2023)
摘要
In recent years, combining neural networks with local search heuristics has become popular in the field of combinatorial optimization. Despite its considerable computational demands, this approach has exhibited promising outcomes with minimal manual engineering. However, we have identified three critical limitations in the empirical evaluation of these integration attempts. Firstly, instances with moderate complexity and weak baselines pose a challenge in accurately evaluating the effectiveness of learning-based approaches. Secondly, the absence of an ablation study makes it difficult to quantify and attribute improvements accurately to the deep learning architecture. Lastly, the generalization of learned heuristics across diverse distributions remains underexplored. In this study, we conduct a comprehensive investigation into these identified limitations. Surprisingly, we demonstrate that a simple learned heuristic based on Tabu Search surpasses state-of-the-art (SOTA) learned heuristics in terms of performance and generalizability. Our findings challenge prevailing assumptions and open up exciting avenues for future research and innovation in combinatorial optimization.
更多查看译文
关键词
learned local search heuristics,meek,local search
AI 理解论文
溯源树
样例
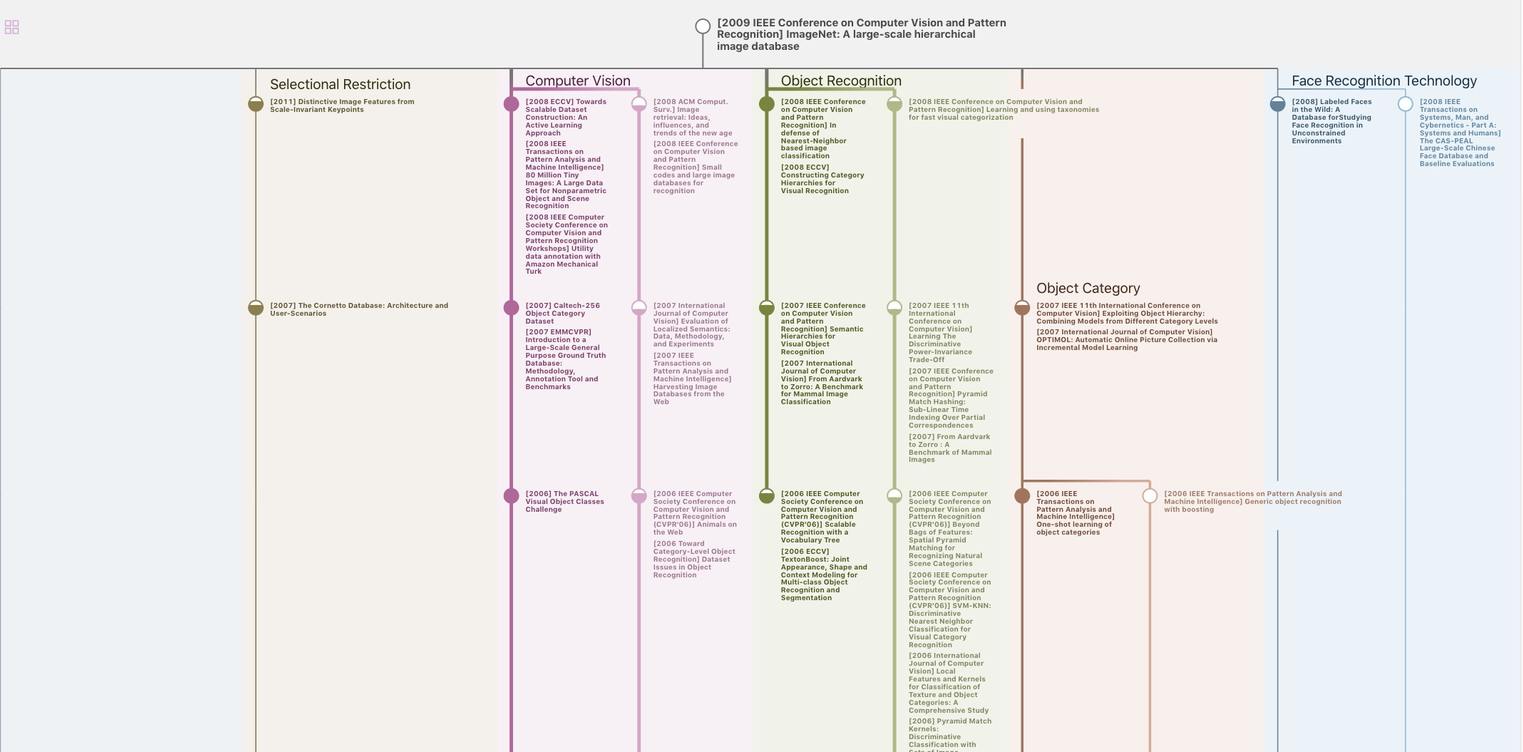
生成溯源树,研究论文发展脉络
Chat Paper
正在生成论文摘要