MIST: Medical Image Segmentation Transformer with Convolutional Attention Mixing (CAM) Decoder
2024 IEEE/CVF Winter Conference on Applications of Computer Vision (WACV)(2023)
摘要
One of the common and promising deep learning approaches used for medical image segmentation is transformers, as they can capture long-range dependencies among the pixels by utilizing self-attention. Despite being successful in medical image segmentation, transformers face limitations in capturing local contexts of pixels in multimodal dimensions. We propose a Medical Image Segmentation Transformer (MIST) incorporating a novel Convolutional Attention Mixing (CAM) decoder to address this issue. MIST has two parts: a pre-trained multi-axis vision transformer (MaxViT) is used as an encoder, and the encoded feature representation is passed through the CAM decoder for segmenting the images. In the CAM decoder, an attention-mixer combining multi-head self-attention, spatial attention, and squeeze and excitation attention modules is introduced to capture long-range dependencies in all spatial dimensions. Moreover, to enhance spatial information gain, deep and shallow convolutions are used for feature extraction and receptive field expansion, respectively. The integration of low-level and high-level features from different network stages is enabled by skip connections, allowing MIST to suppress unnecessary information. The experiments show that our MIST transformer with CAM decoder outperforms the state-of-the-art models specifically designed for medical image segmentation on the ACDC and Synapse datasets. Our results also demonstrate that adding the CAM decoder with a hierarchical transformer improves segmentation performance significantly. Our model with data and code is publicly available on GitHub.
更多查看译文
关键词
Algorithms,Image recognition and understanding,Applications,Biomedical / healthcare / medicine
AI 理解论文
溯源树
样例
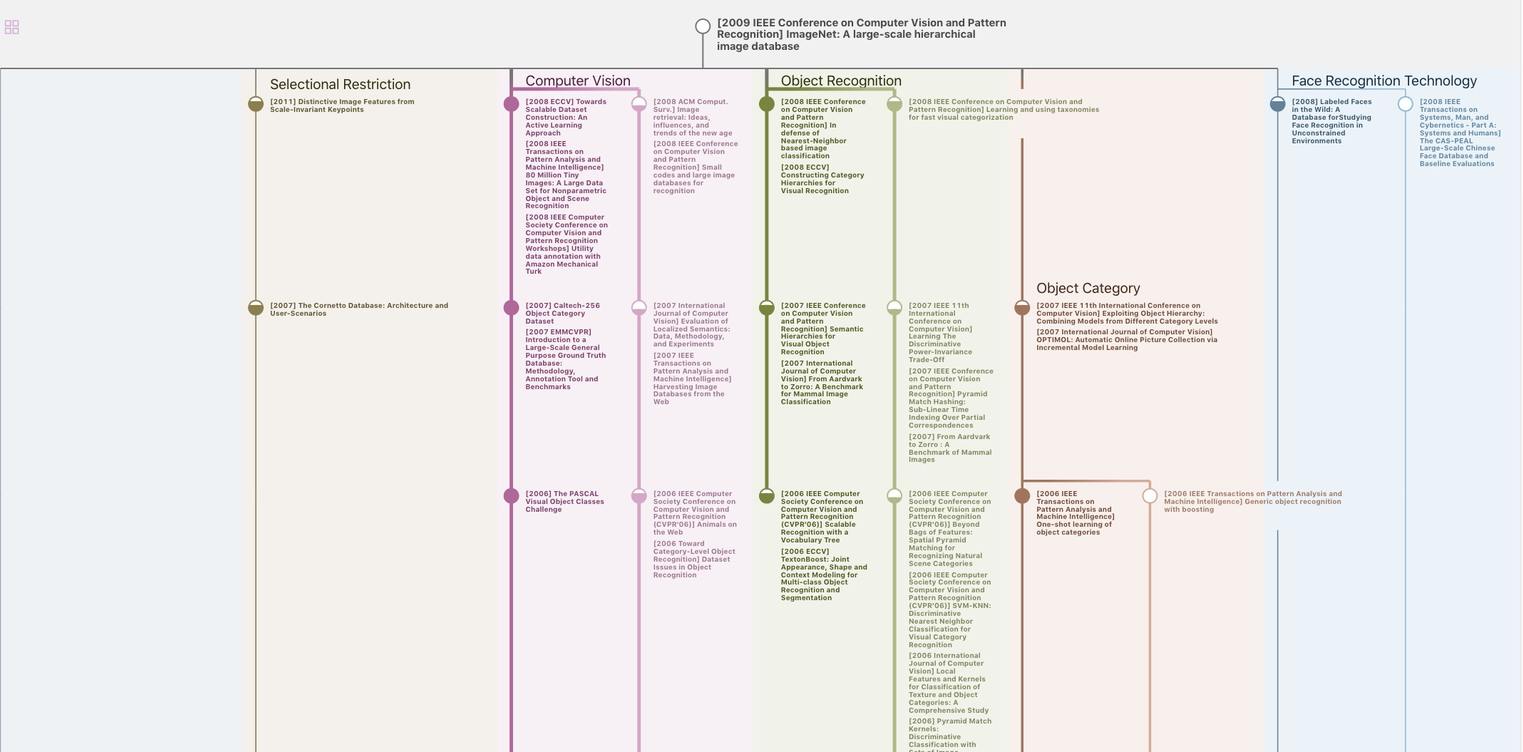
生成溯源树,研究论文发展脉络
Chat Paper
正在生成论文摘要