Exact Recovery and Bregman Hard Clustering of Node-Attributed Stochastic Block Model
arXiv (Cornell University)(2023)
摘要
Network clustering tackles the problem of identifying sets of nodes (communities) that have similar connection patterns. However, in many scenarios, nodes also have attributes that are correlated with the clustering structure. Thus, network information (edges) and node information (attributes) can be jointly leveraged to design high-performance clustering algorithms. Under a general model for the network and node attributes, this work establishes an information-theoretic criterion for the exact recovery of community labels and characterizes a phase transition determined by the Chernoff-Hellinger divergence of the model. The criterion shows how network and attribute information can be exchanged in order to have exact recovery (e.g., more reliable network information requires less reliable attribute information). This work also presents an iterative clustering algorithm that maximizes the joint likelihood, assuming that the probability distribution of network interactions and node attributes belong to exponential families. This covers a broad range of possible interactions (e.g., edges with weights) and attributes (e.g., non-Gaussian models), as well as sparse networks, while also exploring the connection between exponential families and Bregman divergences. Extensive numerical experiments using synthetic data indicate that the proposed algorithm outperforms classic algorithms that leverage only network or only attribute information as well as state-of-the-art algorithms that also leverage both sources of information. The contributions of this work provide insights into the fundamental limits and practical techniques for inferring community labels on node-attributed networks.
更多查看译文
关键词
bregman hard clustering,block model,stochastic
AI 理解论文
溯源树
样例
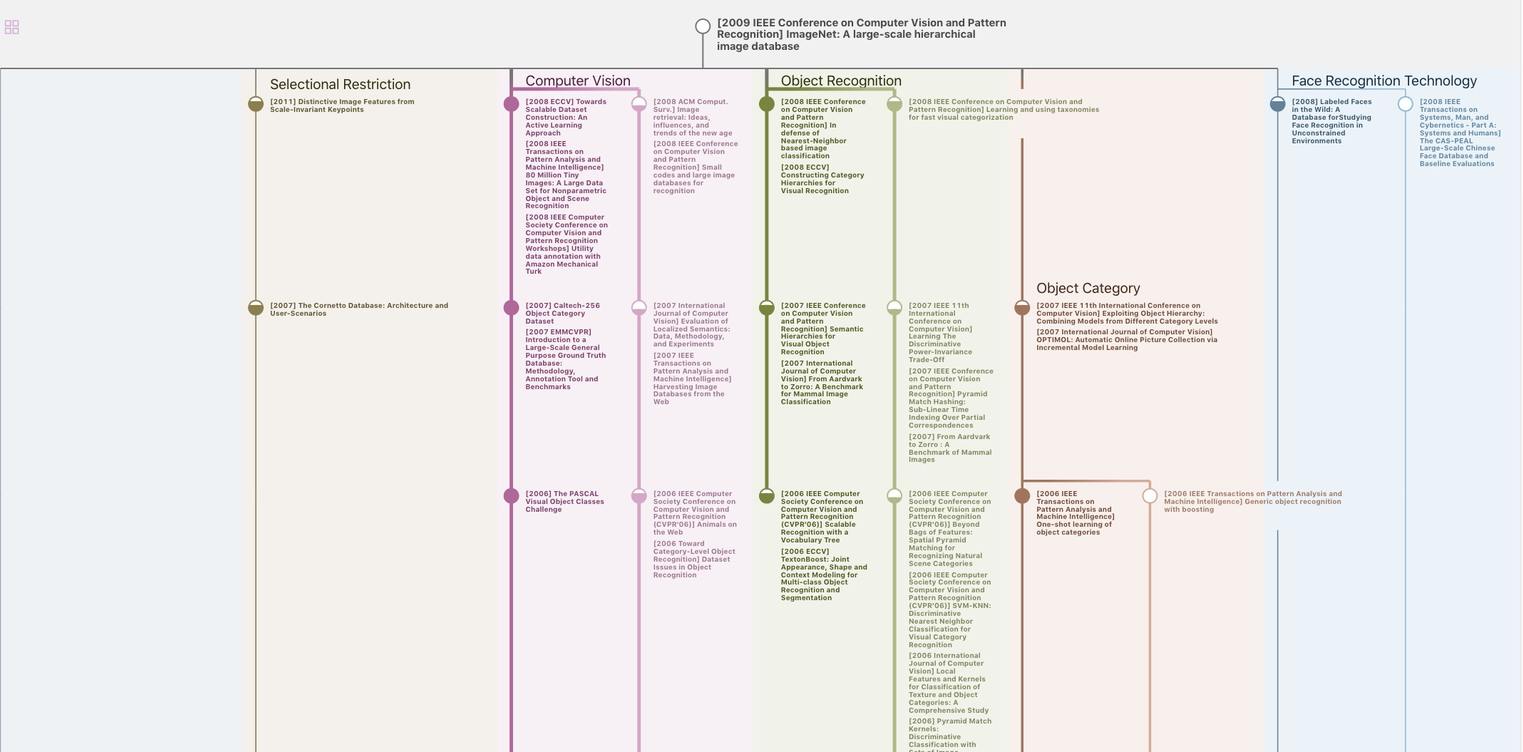
生成溯源树,研究论文发展脉络
Chat Paper
正在生成论文摘要