Enhancing inter-sentence attention for Semantic Textual Similarity
INFORMATION PROCESSING & MANAGEMENT(2024)
摘要
Semantic Textual Similarity (STS) is a fundamental task that aims to measure semantic equiva-lence between two sentences. The pre-trained language models based on Transformer have had great success on the STS task, which is characterized by multi-head self-attention. However, inter-sentence attention referring to attention between a pair of sentences has not been extensively explored or studied, despite its great value to the STS task. To guide models to focus more "attention"on inter-sentence information, such as synonyms, hyponyms, meronyms and antonyms between sentences, we propose a novel multi-head self-attention architecture, namely Enhanced Inter-sentence Attention (EIA). Specifically, EIA combines the enhanced inter-sentence attention with the original attention by a gated fusion module based on Transformer. The architecture effectively integrating the essential information from both attention. Experiments on benchmark datasets verify the effectiveness of our proposed architecture, as the performance of EIA based on RoBERTa reaches 91.22% (Pearson Correlation) and 86.92% (Spearman Correlation) on the SICK dataset, which are 0.52%-2.15% higher than other strong baseline models. Moreover, our EIA can be successfully integrated with other modified transformer-based models to further improve their performance, demonstrating its ability to effectively model matching information between sentences.
更多查看译文
关键词
Semantic textual similarity,Transformer,Multi-head self-attention,Inter-sentence attention
AI 理解论文
溯源树
样例
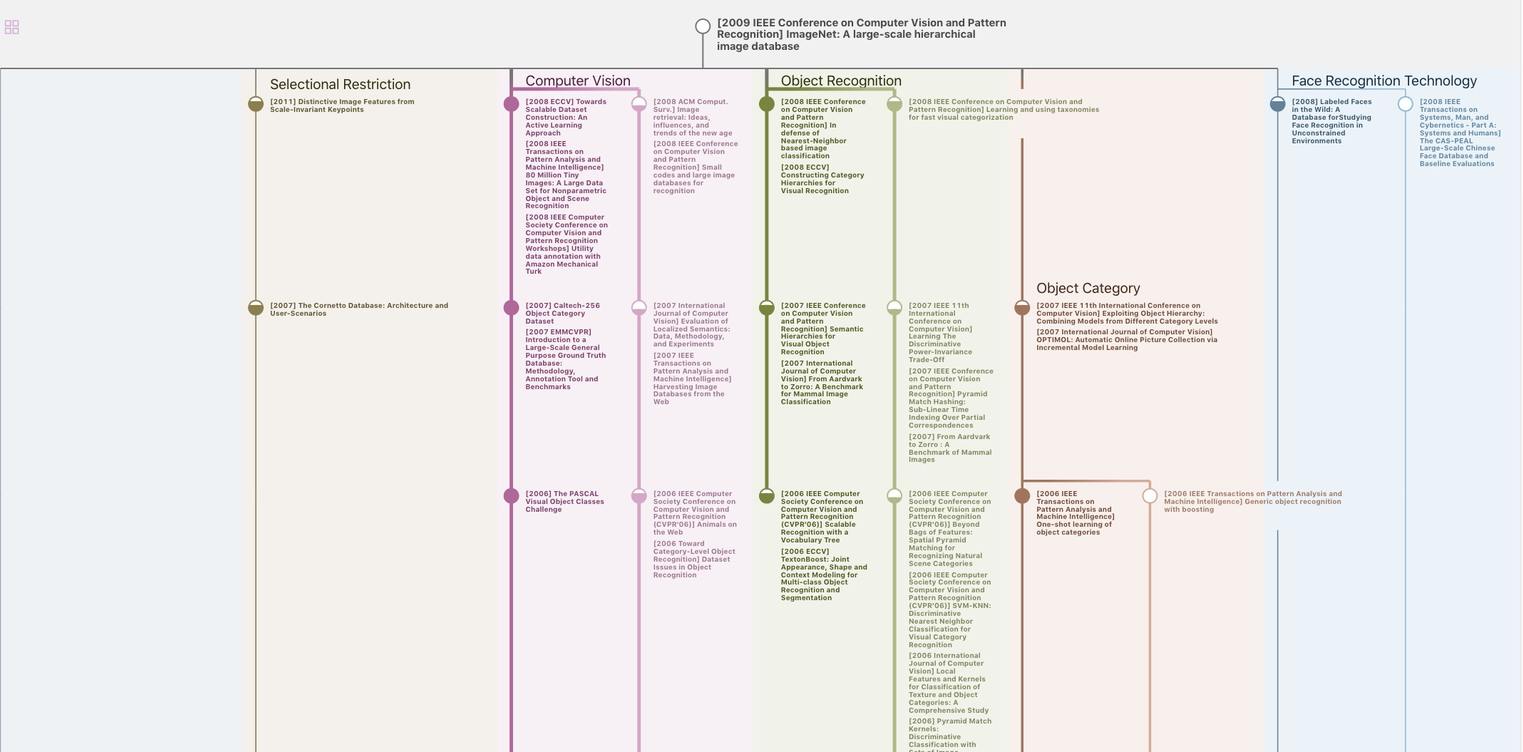
生成溯源树,研究论文发展脉络
Chat Paper
正在生成论文摘要