Bayesian-optimized random forest prediction of key properties of micro-/nanofibrillated cellulose from different woody and non-woody feedstocks
Industrial Crops and Products(2023)
摘要
The scale-up of nanocellulose production, a still challenging task, could benefit greatly from machine learning. In this paper, random forest regressors were applied to predict two important parameters for nanocellulose processing: nanofibrillation yield and aspect ratio. The dataset comprised diverse pulp sources, ranging from non-woody and woody feedstock, and two different types of pulp pre-treatments, namely mechanical refining and enzymatic hydrolysis. The obtained models were evaluated in terms of number of features and optimized with regard to some important hyperparameters, using the Bayesian optimization function from the SkOpt library. Considering the heterogeneity of the data, the prediction of both aspect ratio and yield of nanofibrillation was deemed satisfactory. For the former, R-2 scores of 0.986, 0.902 and 0.932 were obtained for the training, validation and testing subsets, respectively. Regarding the latter, R-2 scores of 0.994, 0.947 and 0.877 were achieved for the same types of subsets. Other important remarks are that enzyme dosage was the most important feature for predicting aspect ratio, while it did not play an important role for yield modelling. For this output, the transmittance at 600 nm accounted for almost 90% of the total feature importance.
更多查看译文
关键词
Aspect ratio,Machine learning,Nanocellulose fibers,Random forest,Yield of nanofibrillation
AI 理解论文
溯源树
样例
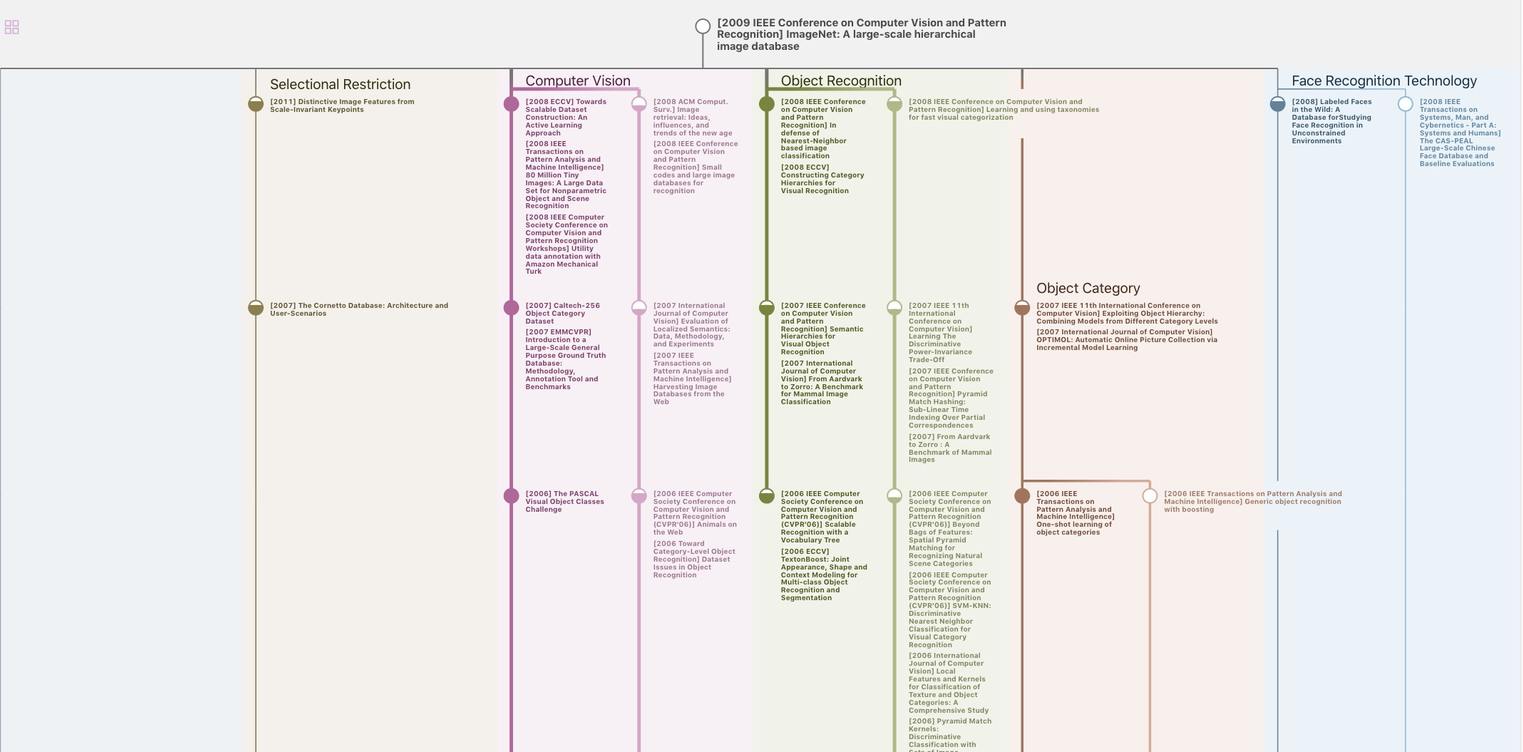
生成溯源树,研究论文发展脉络
Chat Paper
正在生成论文摘要