MRIM: Lightweight saliency-based mixed-resolution imaging for low-power pervasive vision
PERVASIVE AND MOBILE COMPUTING(2023)
摘要
While many pervasive computing applications increasingly utilize real-time context extracted from a vision sensing infrastructure, the high energy overhead of DNN-based vision sensing pipelines remains a challenge for sustainable in-the-wild deployment. One common approach to reducing such energy overheads is the capture and transmission of lower-resolution images to an edge node (where the DNN inferencing task is executed), but this results in an accuracy-vs energy tradeoff, as the DNN inference accuracy typically degrades with a drop in resolution. In this work, we introduce MRIM, a simple but effective framework to tackle this tradeoff. Under MRIM, the vision sensor platform first executes a lightweight preprocessing step to determine the saliency of different sub-regions within a single captured image frame, and then performs a saliency-aware non-uniform downscaling of individual sub-regions to produce a "mixedresolution"image. We describe two novel low-complexity algorithms that the sensor platform can use to quickly compute suitable resolution choices for different regions under different energy/accuracy constraints. Experimental studies, involving object detection tasks evaluated traces from two benchmark urban monitoring datasets as well as a prototype Raspberry Pi-based MRIM implementation, demonstrate MRIM's efficacy: even with an unoptimized embedded platform, MRIM can provide system energy conservation of 35 + % (similar to 80% in high accuracy regimes) or increase task accuracy by 8 + %, over conventional baselines of uniform resolution downscaling or image encoding, while supporting high throughput. On a low power ESP32 vision board, MRIM continues to provide 60+% energy savings over uniform downscaling while maintaining high detection accuracy. We further introduce an automated data-driven technique for determining a close-to-optimal number of MRIM sub-regions (for differential resolution adjustment), across different deployment conditions. We also show the generalized use of MRIM by considering an additional license plate recognition (LPR) task: while alternative approaches suffer 35%-40% loss in accuracy, MRIM suffers only a modest recognition loss of similar to 10% even when the transmission data is reduced by over 50%.
更多查看译文
关键词
Mixed resolution,Pervasive vision tasks,Energy consumption
AI 理解论文
溯源树
样例
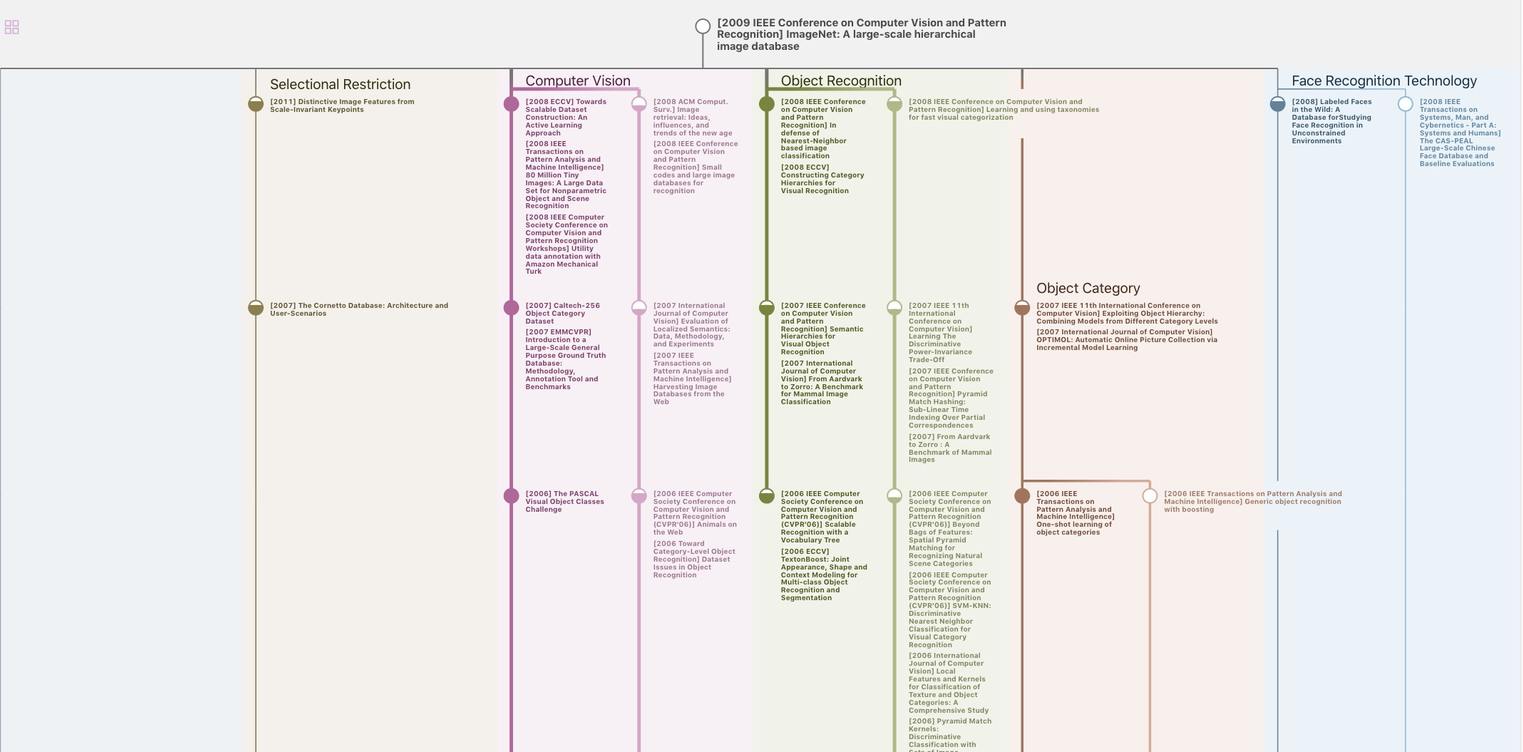
生成溯源树,研究论文发展脉络
Chat Paper
正在生成论文摘要