A data-driven newsvendor problem: A high-dimensional and mixed-frequency method
International Journal of Production Economics(2023)
摘要
In this paper, a data-driven newsvendor problem is studied by mapping high-dimensional and mixed-frequency features of historical data to replenishment decisions. Instead of relying on a known demand distribution, we propose using machine learning algorithms that incorporate demand features into the replenishment decisions to solve single- and multi-product newsvendor problems. In particular, our algorithms simultaneously optimize the demand estimation and replenishment decisions. To extract key features from the historical data, we propose a frequency alignment method to transform high-dimensional mixed-frequency data and historical data into the same frequency. We then propose two variable selection policies based on the empirical risk minimization principle, and employ the regularization method to tackle the parameter proliferation issue. In addition, a feature-based machine learning algorithm is designed to solve the multi-product newsvendor problem. Finally, we numerically justify the performances of proposed machine learning algorithms. We find that (1) data-informed replenishment decisions can effectively leverage the identified key demand features to avoid losing demand information, and (2) the optimal replenishment quantity behaves robustly and shows minimal variation across different cost structures. Our work provides meaningful insights for newsvendors making replenishment decisions under stochastic demand.
更多查看译文
关键词
newsvendor problem,data-driven,high-dimensional,mixed-frequency
AI 理解论文
溯源树
样例
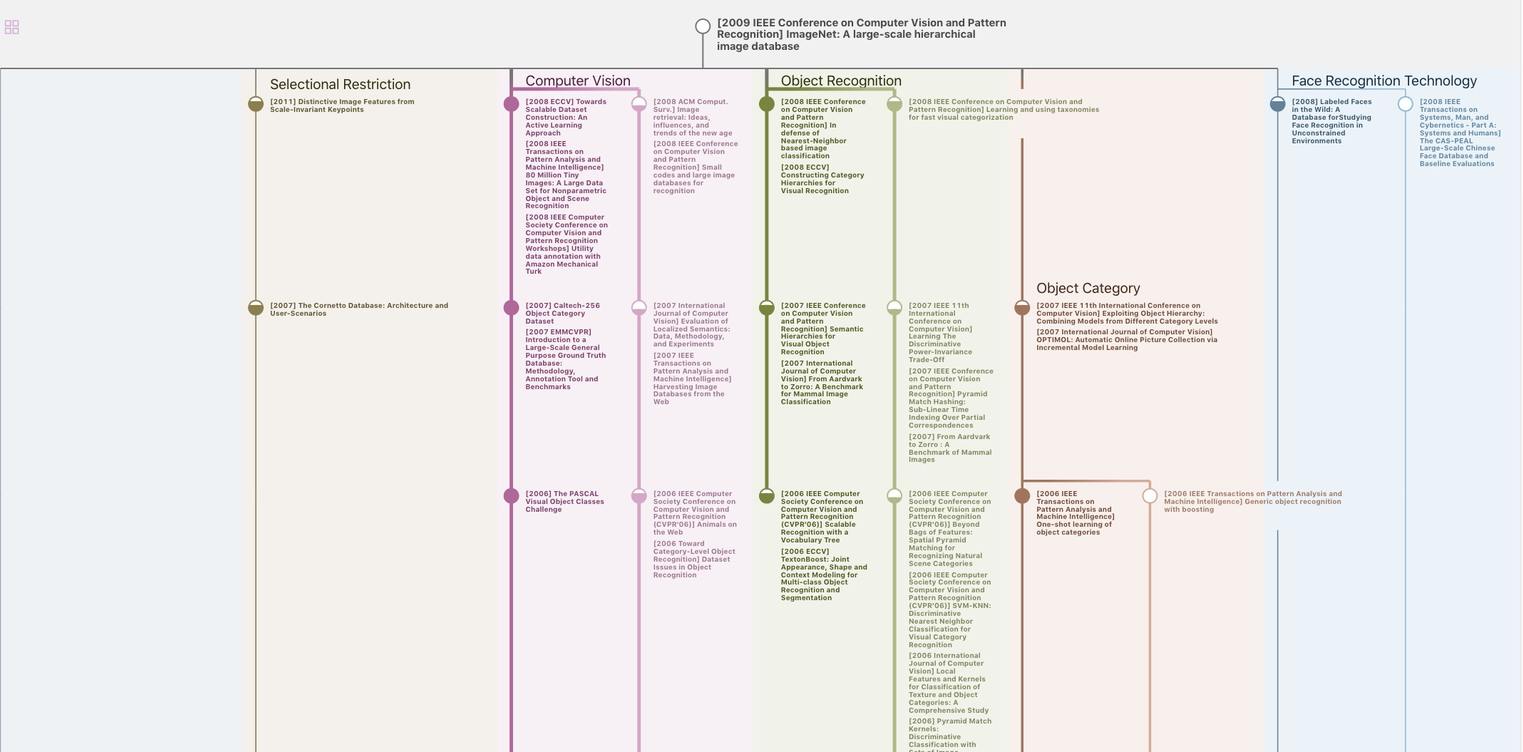
生成溯源树,研究论文发展脉络
Chat Paper
正在生成论文摘要