Analysis the molecular similarity of least common amino acid sites in ACE2 receptor to predict the potential susceptible species for SARS-CoV-2
bioRxiv (Cold Spring Harbor Laboratory)(2023)
摘要
This research offers a bioinformatics approach to forecasting both domestic and wild animals’ likelihood of being susceptible to SARS-CoV-2 infection. Genomic sequencing can resolve phylogenetic relationships between the virus and the susceptible host. The genome sequence of SARS-CoV-2 is highly interactive with the specific sequence region of the ACE2 receptor of the host species. We further evaluate this concept to identify the most important SARS-CoV-2 binding amino acid sites in the ACE2 receptor sequence through the common similarity of the last common amino acid sites (LCAS) in known susceptible host species. Therefore, the SARS-CoV-2 viral genomic interacting key amino acid region in the ACE2 receptor sequence of known susceptible human host was summarized and compared with other reported known SARS-CoV-2 susceptible host species. We identified the 10 most significant amino acid sites for interaction with SARS-CoV-2 infection from the ACE2 receptor sequence region based on the LCAS similarity pattern in known sensitive SARS-CoV-2 hosts. The most significant 10 LCAS were further compared with ACE2 receptor sequences of unknown species to evaluate the similarity of the last common amino acid pattern (LCAP). We predicted the probability of SARS-CoV-2 infection risk in unknown species through the LCAS similarity pattern. This method can be used as a screening tool to assess the risk of SARS-CoV-2 infection in domestic and wild animals to prevent outbreaks of infection.
![Figure][1]
### Competing Interest Statement
The authors have declared no competing interest.
[1]: pending:yes
更多查看译文
关键词
ace2 receptor,molecular similarity,amino,sars-cov
AI 理解论文
溯源树
样例
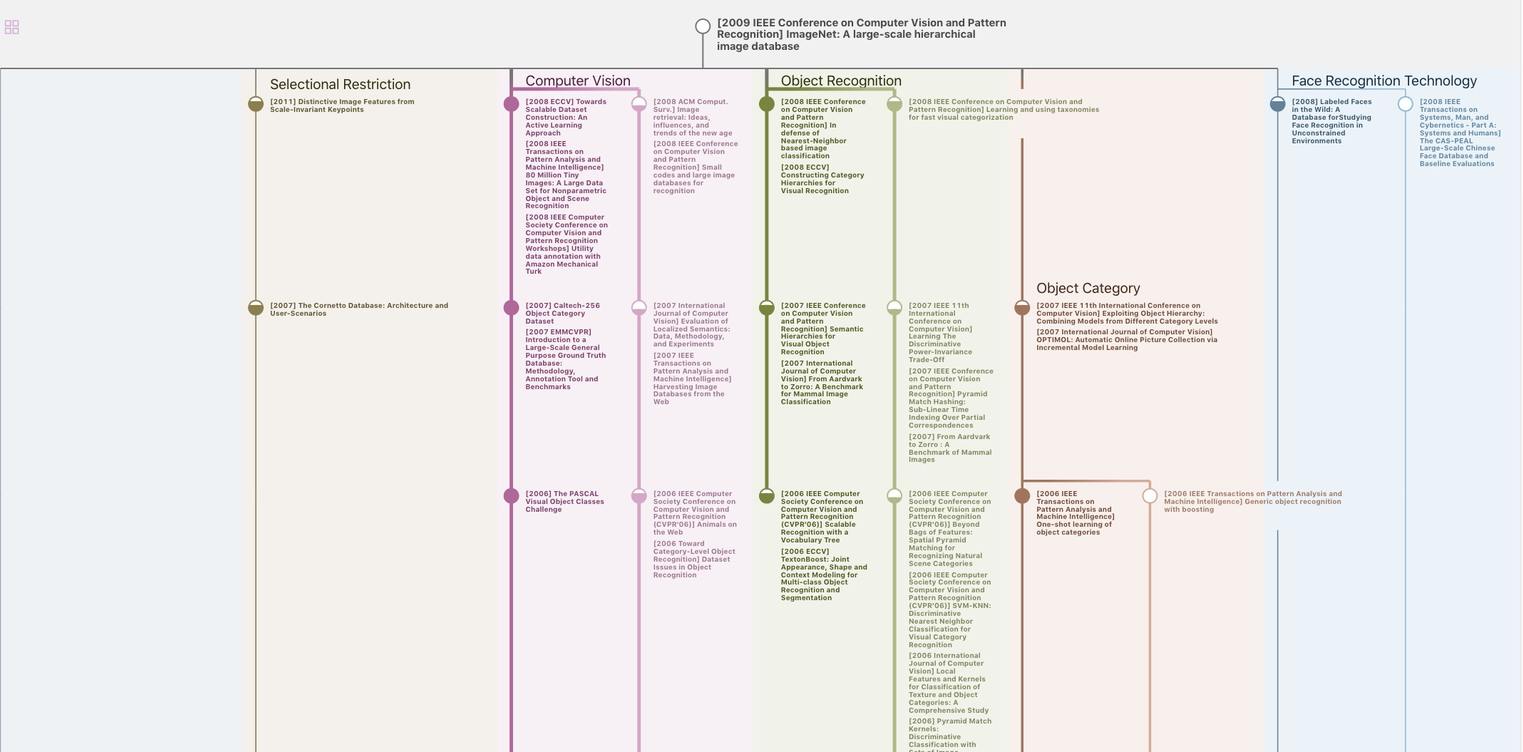
生成溯源树,研究论文发展脉络
Chat Paper
正在生成论文摘要