scDILT: a model-based and constrained deep learning framework for single-cell Data Integration, Label Transferring, and clustering
bioRxiv (Cold Spring Harbor Laboratory)(2023)
摘要
The scRNA-seq technology enables high-resolution profiling and analysis of individual cells. The increasing availability of datasets and advancements in technology have prompted researchers to integrate existing annotated datasets with newly sequenced datasets for a more comprehensive analysis. It is important to ensure that the integration of new datasets does not alter the cell clusters defined in the old/reference datasets. Although several methods have been developed for scRNA-seq data integration, there is currently a lack of tools that can simultaneously achieve the aforementioned objectives. Therefore, in this study, we have introduced a novel tool called scDILT, which leverages a conditional autoencoder and deep embedding clustering to effectively remove batch effects among different datasets. Moreover, scDILT utilizes homogeneous constraints to preserve the cell-type/clustering patterns observed in the reference datasets, while employing heterogeneous constraints to map cells in the new datasets to the annotated cell clusters in the reference datasets. We have conducted extensive experiments to demonstrate that scDILT outperforms other methods in terms of data integration, as confirmed by evaluations on simulated and real datasets. Furthermore, we have shown that scDILT can be successfully applied to integrate multi-omics single-cell datasets. Based on these findings, we conclude that scDILT holds great promise as a tool for integrating single-cell datasets derived from different batches, experiments, times, or interventions.
### Competing Interest Statement
The authors have declared no competing interest.
更多查看译文
关键词
label transferring,deep learning framework,deep learning,model-based,single-cell
AI 理解论文
溯源树
样例
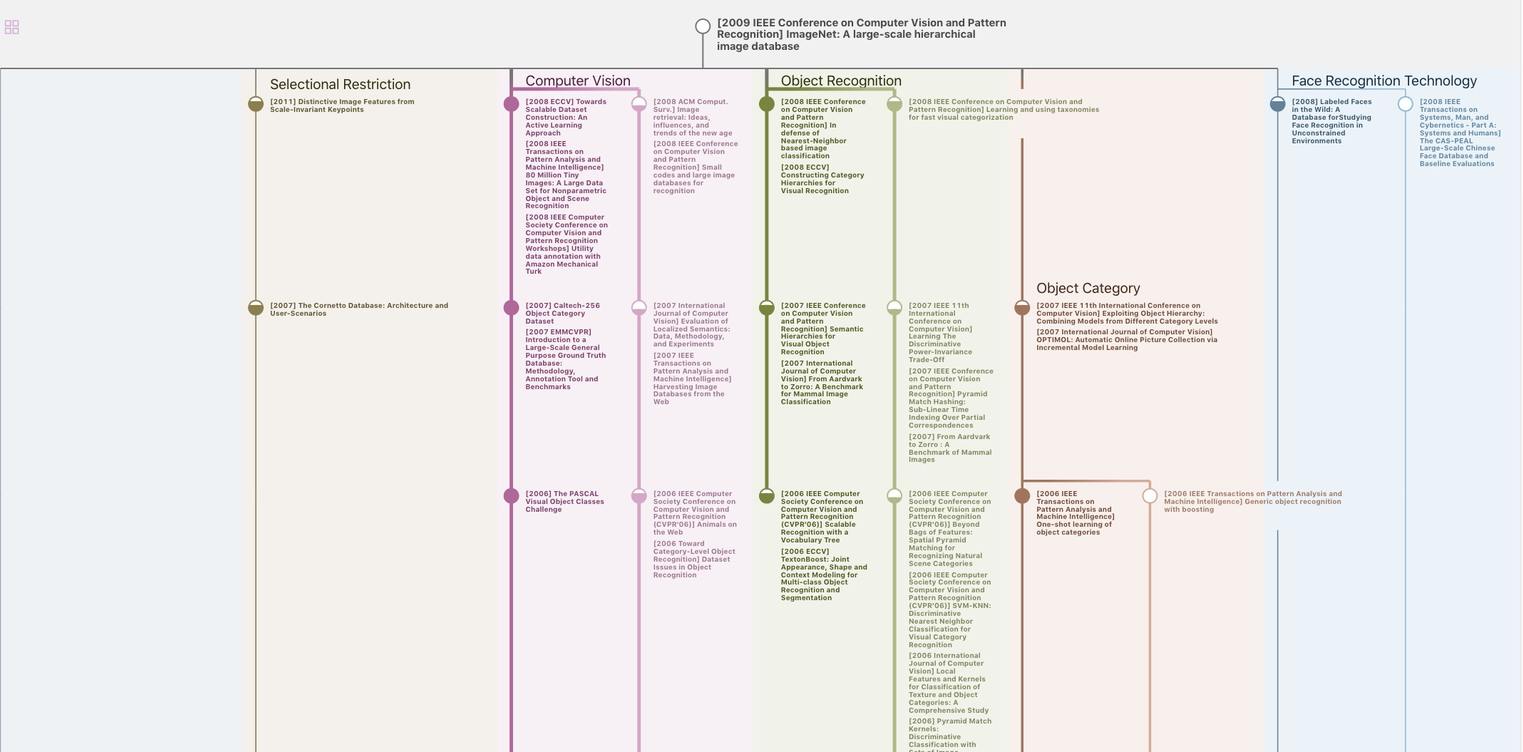
生成溯源树,研究论文发展脉络
Chat Paper
正在生成论文摘要