RIS Subarray Optimization With Reinforcement Learning for Green Symbiotic Communications in Internet of Things
IEEE INTERNET OF THINGS JOURNAL(2023)
摘要
Symbiotic communications have been deemed as a critical technology for Internet of Things (IoT) communications owing to its high spectrum and energy efficiency. Reconfigurable intelligent surface (RIS), which can tune wireless transmission channels by manipulating incident waves through the corresponding electromagnetic elements, is a promising enabler of various symbiotic communications scenarios in IoT. However, when the full electromagnetic elements of RIS are activated, system capacity will be improved and energy efficiency will be reduced inevitably, also with undesirable power consumption. To address this issue, an intelligent dynamic subarray RIS framework based on deep reinforcement learning (DRL) has been proposed. The key idea is to divide RIS electromagnetic elements into several groups and optimize power amplifier factor, independent phase shifts to improve the system energy efficiency under the premise of user's basic requirements. In particular, we formulate a hybrid optimization problem of RIS subarray partition and beamforming to maximize system energy efficiency. It can be proved that this hybrid optimization is a mixed nonconvex integer programming problem. To solve this issue, we proposed a comprehensive DRL framework, including two parts, i.e., 1) a Markov decision process (MDP) to model the subarray partition design, amplitude, and phase shifts of RIS and 2) an active RIS subarray optimization scheme based on deep deterministic policy gradient. Numerical results have demonstrated that, compared with the conventional fully-connected RIS, the system energy efficiency can be significantly improved.
更多查看译文
关键词
Deep reinforcement learning (DRL),energy efficiency,parameters optimization,reconfigurable intelligent surfaces (RISs),subarray partition
AI 理解论文
溯源树
样例
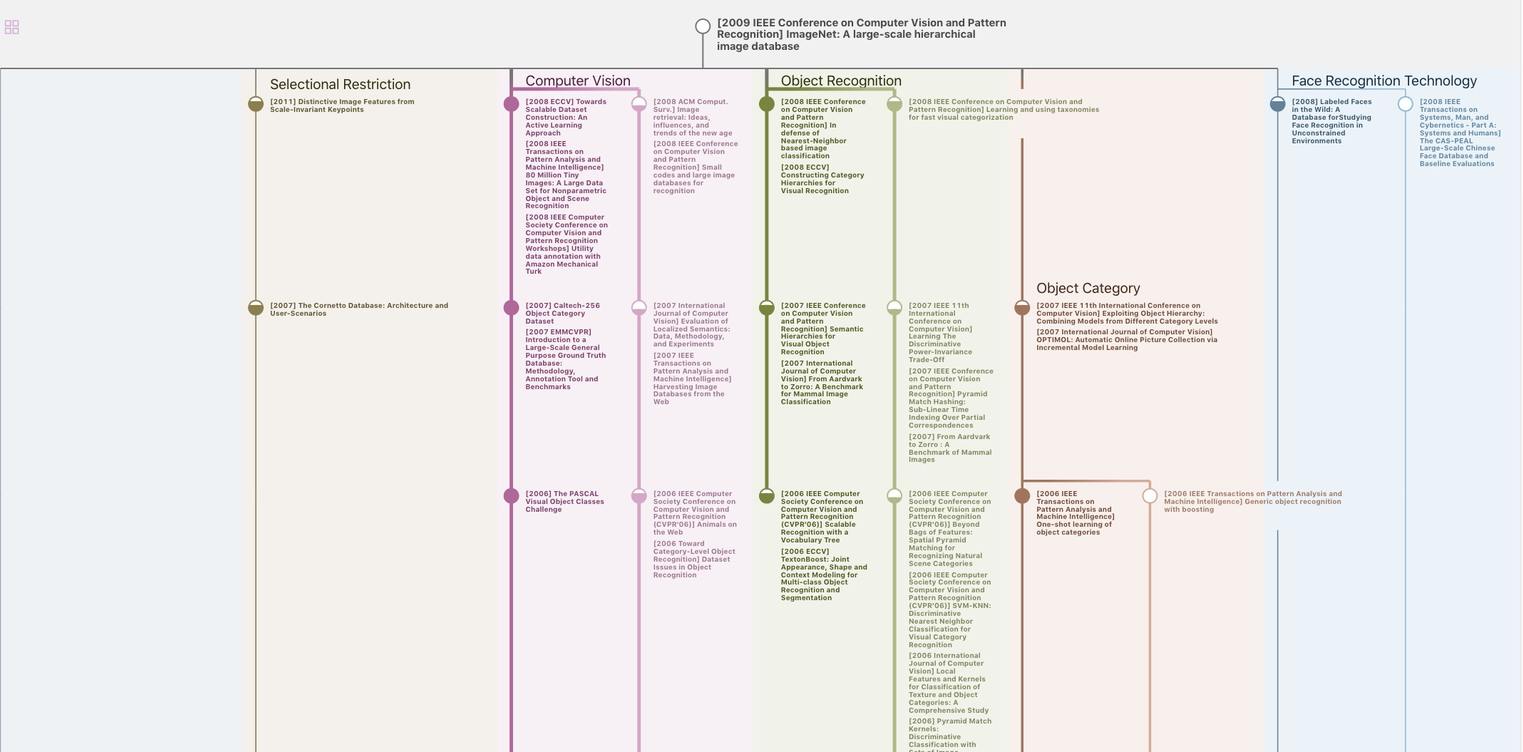
生成溯源树,研究论文发展脉络
Chat Paper
正在生成论文摘要