Systematic improvement of empirical energy functions in the era of machine learning.
Journal of computational chemistry(2024)
摘要
The impact of targeted replacement of individual terms in empirical force fields is quantitatively assessed for pure water, dichloromethane (CH 2 $$ {}_2 $$ Cl 2 $$ {}_2 $$ ), and solvated K + $$ {}^{+} $$ and Cl - $$ {}^{-} $$ ions. For the electrostatic interactions, point charges (PCs) and machine learning (ML)-based minimally distributed charges (MDCM) fitted to the molecular electrostatic potential are evaluated together with electrostatics based on the Coulomb integral. The impact of explicitly including second-order terms is investigated by adding a fragment molecular orbital (FMO)-derived polarization energy to an existing force field, in this case CHARMM. It is demonstrated that anisotropic electrostatics reduce the RMSE for water (by 1.4 kcal/mol), CH 2 $$ {}_2 $$ Cl 2 $$ {}_2 $$ (by 0.8 kcal/mol) and for solvated Cl - $$ {}^{-} $$ clusters (by 0.4 kcal/mol). An additional polarization term can be neglected for CH 2 $$ {}_2 $$ Cl 2 $$ {}_2 $$ but further improves the models for pure water (by ∼ $$ \sim $$ 1.0 kcal/mol) and hydrated Cl - $$ {}^{-} $$ (by 0.4 kcal/mol), and is key for solvated K + $$ {}^{+} $$ , reducing the RMSE by 2.3 kcal/mol. A 12-6 Lennard-Jones functional form performs satisfactorily with PC and MDCM electrostatics, but is not appropriate for descriptions that account for the electrostatic penetration energy. The importance of many-body contributions is assessed by comparing a strictly 2-body approach with self-consistent reference data. Two-body interactions suffice for CH 2 $$ {}_2 $$ Cl 2 $$ {}_2 $$ whereas water and solvated K + $$ {}^{+} $$ and Cl - $$ {}^{-} $$ ions require explicit many-body corrections. Finally, a many-body-corrected dimer potential energy surface exceeds the accuracy attained using a conventional empirical force field, potentially reaching that of an FMO calculation. The present work systematically quantifies which terms improve the performance of an existing force field and what reference data to use for parametrizing these terms in a tractable fashion for ML fitting of pure and heterogeneous systems.
更多查看译文
AI 理解论文
溯源树
样例
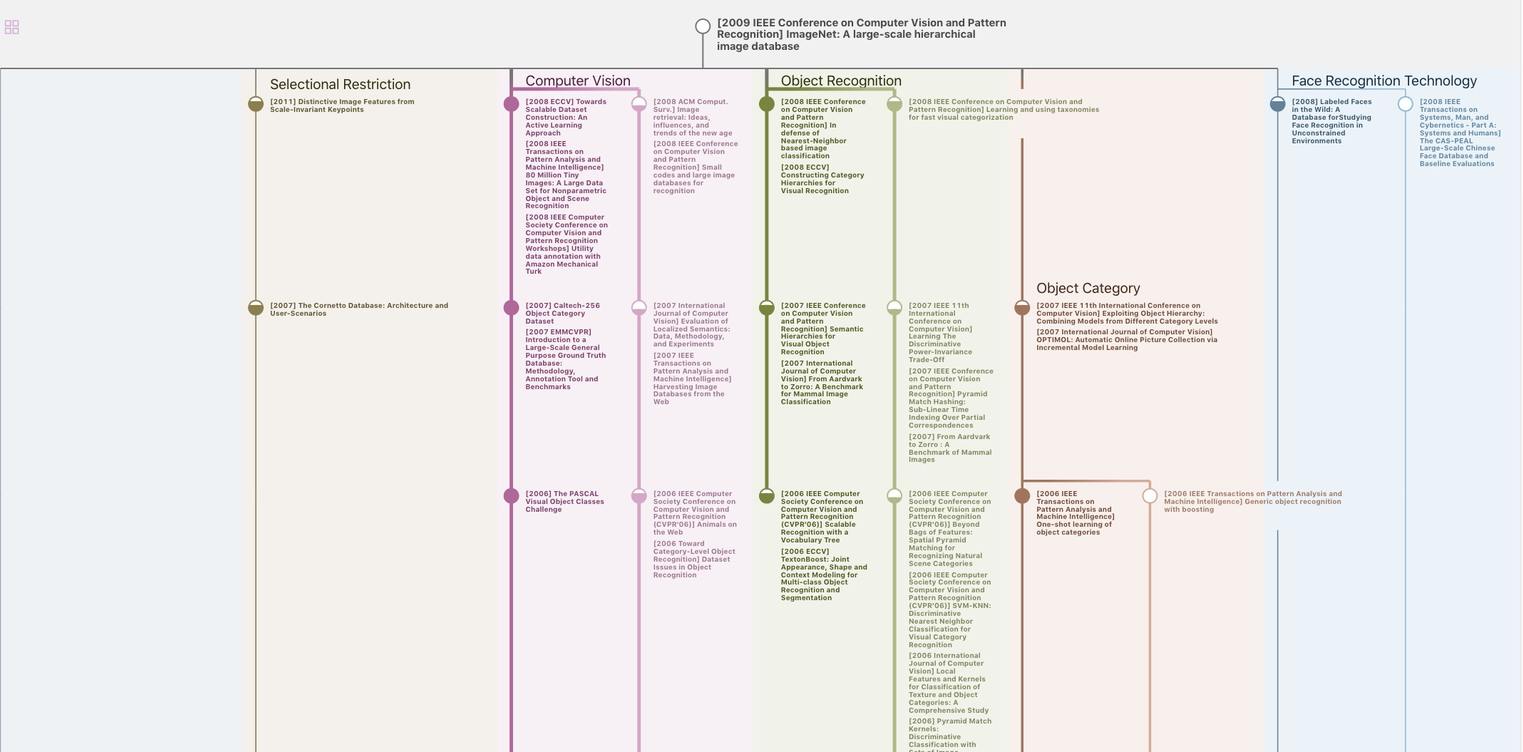
生成溯源树,研究论文发展脉络
Chat Paper
正在生成论文摘要