Variance-based sensitivity of Bayesian inverse problems to the prior distribution
arXiv (Cornell University)(2023)
摘要
The formulation of Bayesian inverse problems involves choosing prior distributions; choices that seem equally reasonable may lead to significantly different conclusions. We develop a computational approach to better understand the impact of the hyperparameters defining the prior on the posterior statistics of the quantities of interest. Our approach relies on global sensitivity analysis (GSA) of Bayesian inverse problems with respect to the hyperparameters defining the prior. This, however, is a challenging problem--a naive double loop sampling approach would require running a prohibitive number of Markov chain Monte Carlo (MCMC) sampling procedures. The present work takes a foundational step in making such a sensitivity analysis practical through (i) a judicious combination of efficient surrogate models and (ii) a tailored importance sampling method. In particular, we can perform accurate GSA of posterior prediction statistics with respect to prior hyperparameters without having to repeat MCMC runs. We demonstrate the effectiveness of the approach on a simple Bayesian linear inverse problem and a nonlinear inverse problem governed by an epidemiological model
更多查看译文
关键词
bayesian inverse problems,prior
AI 理解论文
溯源树
样例
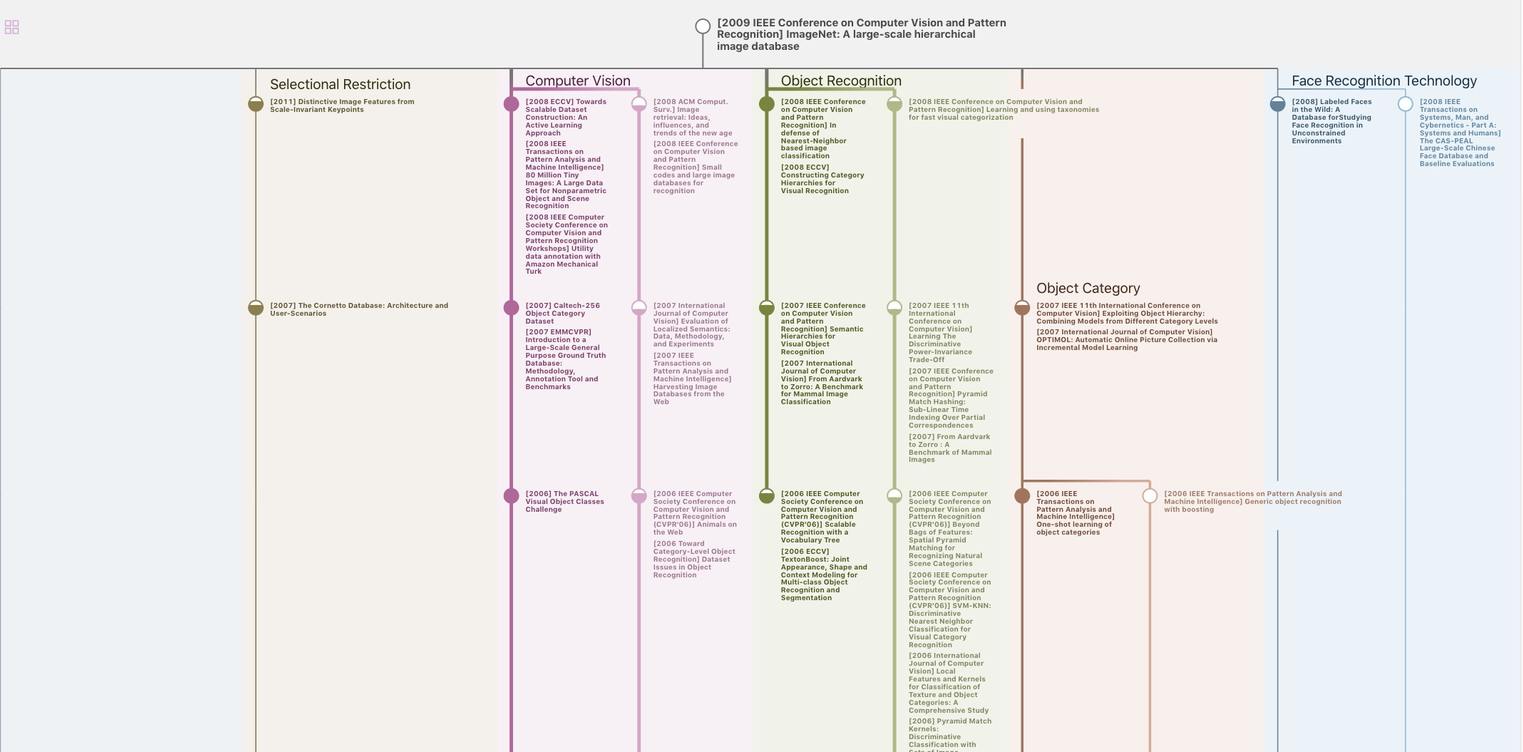
生成溯源树,研究论文发展脉络
Chat Paper
正在生成论文摘要