Weighted Sampled Split Learning (WSSL): Balancing Privacy, Robustness, and Fairness in Distributed Learning Environments
CoRR(2023)
摘要
This study presents Weighted Sampled Split Learning (WSSL), an innovative framework tailored to bolster privacy, robustness, and fairness in distributed machine learning systems. Unlike traditional approaches, WSSL disperses the learning process among multiple clients, thereby safeguarding data confidentiality. Central to WSSL's efficacy is its utilization of weighted sampling. This approach ensures equitable learning by tactically selecting influential clients based on their contributions. Our evaluation of WSSL spanned various client configurations and employed two distinct datasets: Human Gait Sensor and CIFAR-10. We observed three primary benefits: heightened model accuracy, enhanced robustness, and maintained fairness across diverse client compositions. Notably, our distributed frameworks consistently surpassed centralized counterparts, registering accuracy peaks of 82.63% and 75.51% for the Human Gait Sensor and CIFAR-10 datasets, respectively. These figures contrast with the top accuracies of 81.12% and 58.60% achieved by centralized systems. Collectively, our findings champion WSSL as a potent and scalable successor to conventional centralized learning, marking it as a pivotal stride forward in privacy-focused, resilient, and impartial distributed machine learning.
更多查看译文
关键词
balancing privacy,split learning,fairness
AI 理解论文
溯源树
样例
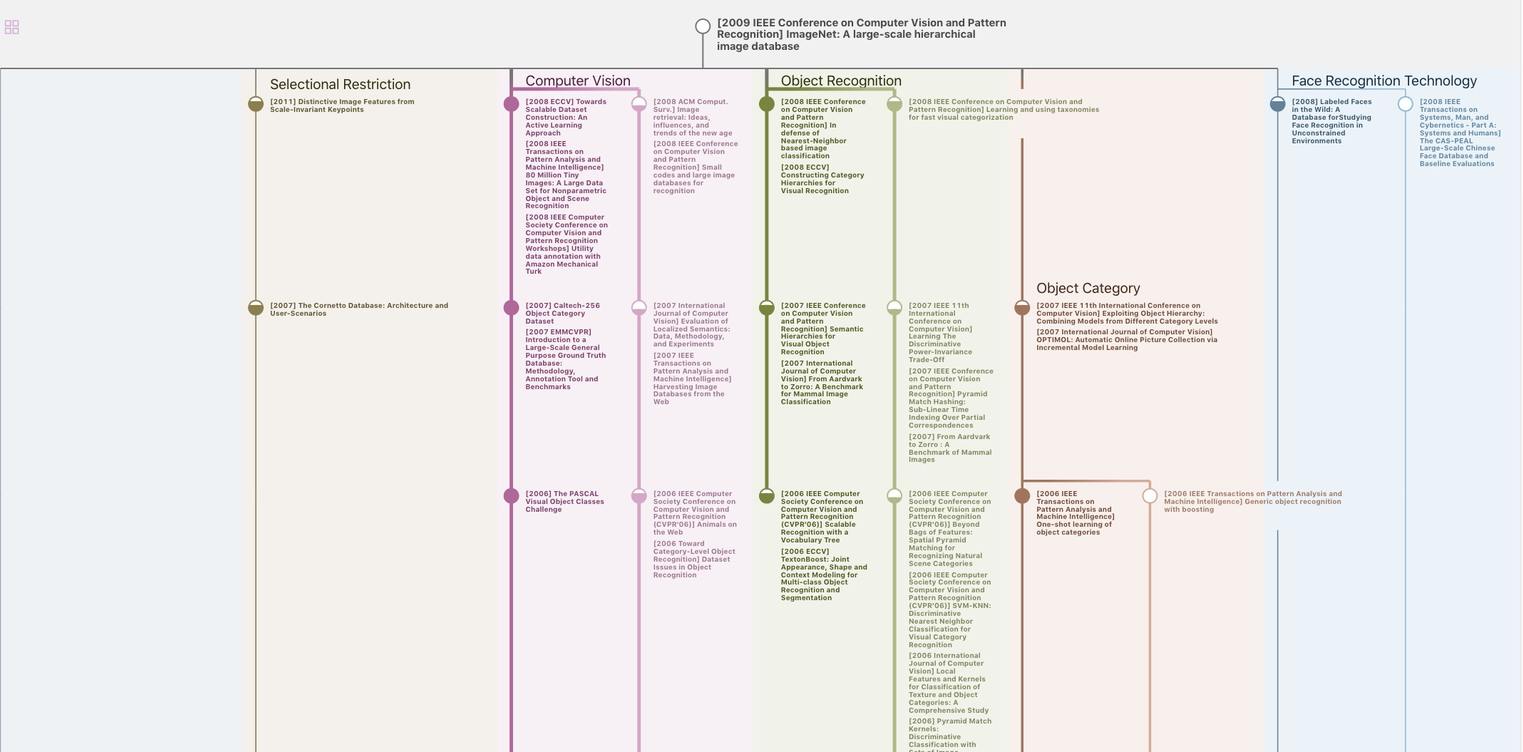
生成溯源树,研究论文发展脉络
Chat Paper
正在生成论文摘要