Compressed models for co-reference resolution: enhancing efficiency with debiased word embeddings
Scientific reports(2023)
摘要
This work presents a comprehensive approach to reduce bias in word embedding vectors and evaluate the impact on various Natural Language Processing (NLP) tasks. Two GloVe variations (840B and 50) are debiased by identifying the gender direction in the word embedding space and then removing or reducing the gender component from the embeddings of target words, while preserving useful semantic information. Their gender bias is assessed through the Word Embedding Association Test. The performance of co-reference resolution and text classification models trained on both original and debiased embeddings is evaluated in terms of accuracy. A compressed co-reference resolution model is examined to gauge the effectiveness of debiasing techniques on resource-efficient models. To the best of the authors’ knowledge, this is the first attempt to apply compression techniques to debiased models. By analyzing the context preservation of debiased embeddings using a Twitter misinformation dataset, this study contributes valuable insights into the practical implications of debiasing methods for real-world applications such as person profiling.
更多查看译文
关键词
compressed models,resolution,co-reference
AI 理解论文
溯源树
样例
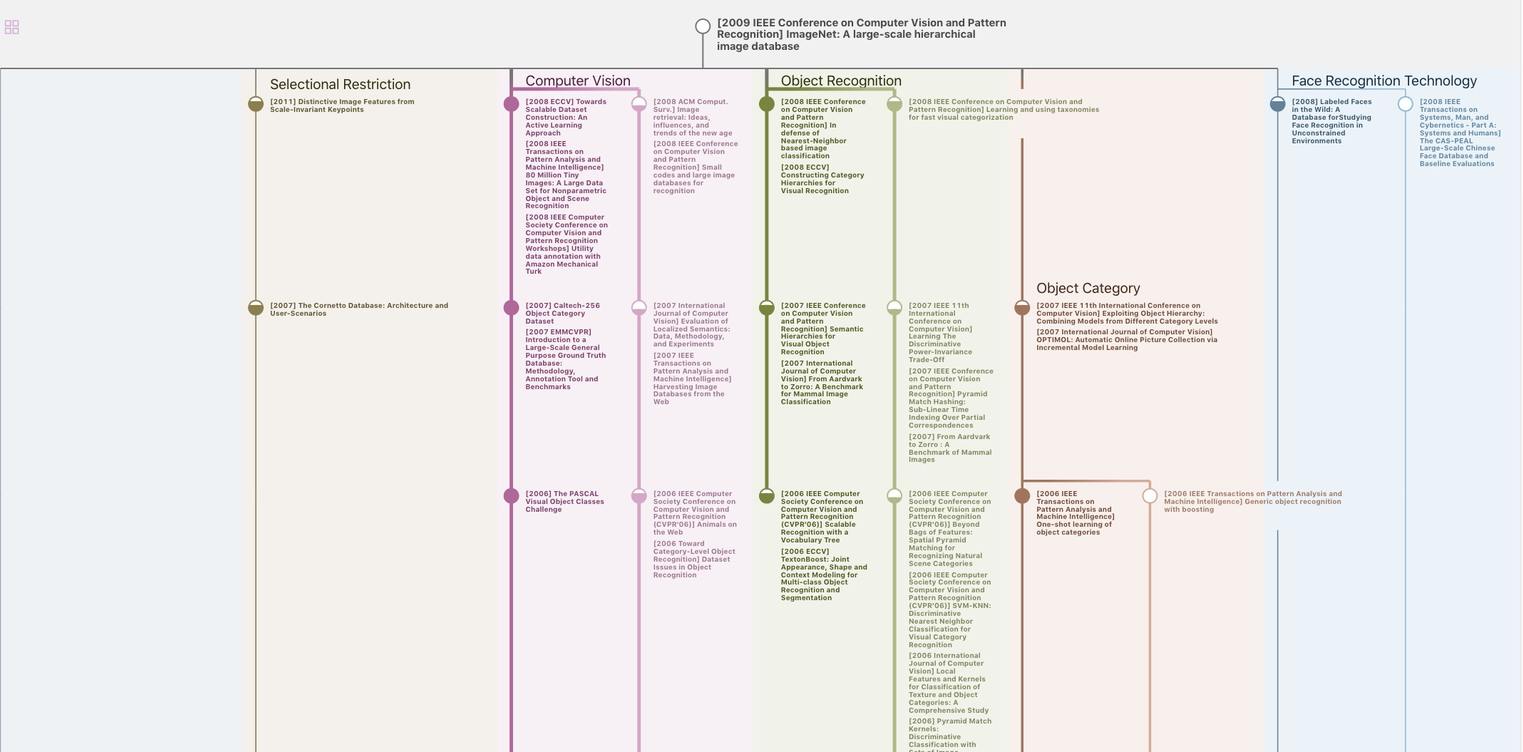
生成溯源树,研究论文发展脉络
Chat Paper
正在生成论文摘要