MLP-COMET-based decision model re-identification for continuous decision-making in the complex network environment.
FedCSIS(2023)
摘要
In recent years, complex networks have gained significant attention for their practical potential in data analysis and decision-making. However, assessing node relevance in complex networks poses challenges, including subjectivity and difficulty reproducing criteria relationships. To address these issues, we propose MLP-COMET. This novel approach combines the Multi-Layer Perceptron (MLP) with the Characteristic Objects Method (COMET) in Multi-Criteria Decision Analysis (MCDA). MLP-COMET aims to re-identify decision models using MLP to evaluate characteristic objects. We evaluate the approach to assessing the complex network and demonstrate its effectiveness in evaluating without heavy reliance on domain experts. The MLP-COMET performance is evaluated through ranking comparisons, showing a strong correlation with reference expert rankings. We also analyze the impact of training sample size and number of characteristic objects on ranking similarity, observing high stability and similarity using the r
w
metric. MLP-COMET offers an effective and reliable tool for evaluating complex networks and facilitating decision-making processes.
更多查看译文
关键词
complex network environment,decision decision-making,mlp-comet-based,re-identification
AI 理解论文
溯源树
样例
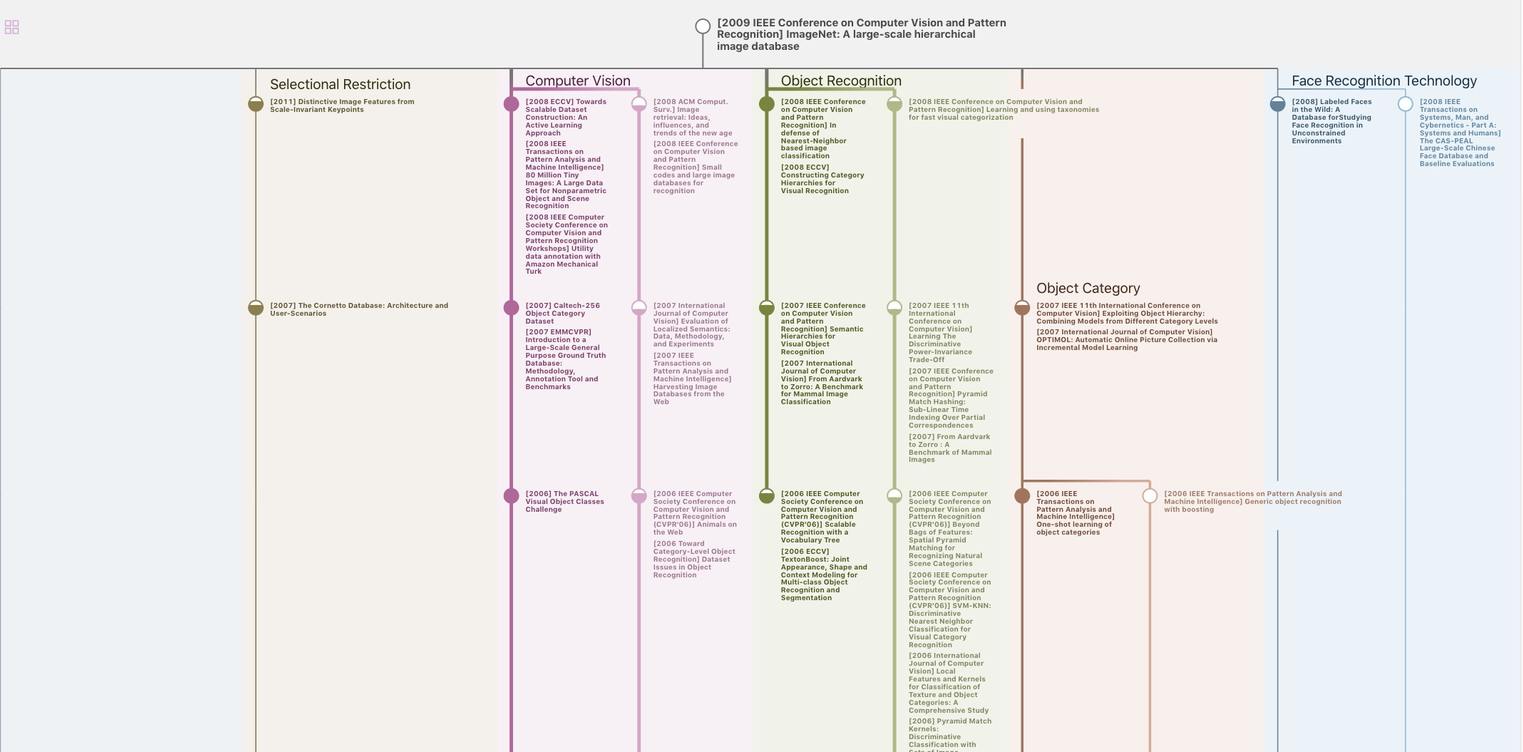
生成溯源树,研究论文发展脉络
Chat Paper
正在生成论文摘要