Controlling Cumulative Adverse Risk in Learning Optimal Dynamic Treatment Regimens
JOURNAL OF THE AMERICAN STATISTICAL ASSOCIATION(2023)
摘要
Dynamic treatment regimen (DTR) is one of the most important tools to tailor treatment in personalized medicine. For many diseases such as cancer and type 2 diabetes mellitus (T2D), more aggressive treatments can lead to a higher efficacy but may also increase risk. However, few methods for estimating DTRs can take into account both cumulative benefit and risk. In this work, we propose a general statistical learning framework to learn optimal DTRs that maximize the reward outcome while controlling the cumulative adverse risk to be below a pre-specified threshold. We convert this constrained optimization problem into an unconstrained optimization using a Lagrange function. We then solve the latter using either backward learning algorithms or simultaneously over all stages based on constructing a novel multistage ramp loss. Theoretically, we establish Fisher consistency of the proposed method and further obtain non-asymptotic convergence rates for both reward and risk outcomes under the estimated DTRs. The finite sample performance of the proposed method is demonstrated via simulation studies and through an application to a two-stage clinical trial for T2D patients. Supplementary materials for this article are available online.
更多查看译文
关键词
Benefit-risk tradeoff,Lagrange function,Multistage decision-making,Outcome weighted learning,Q-learning
AI 理解论文
溯源树
样例
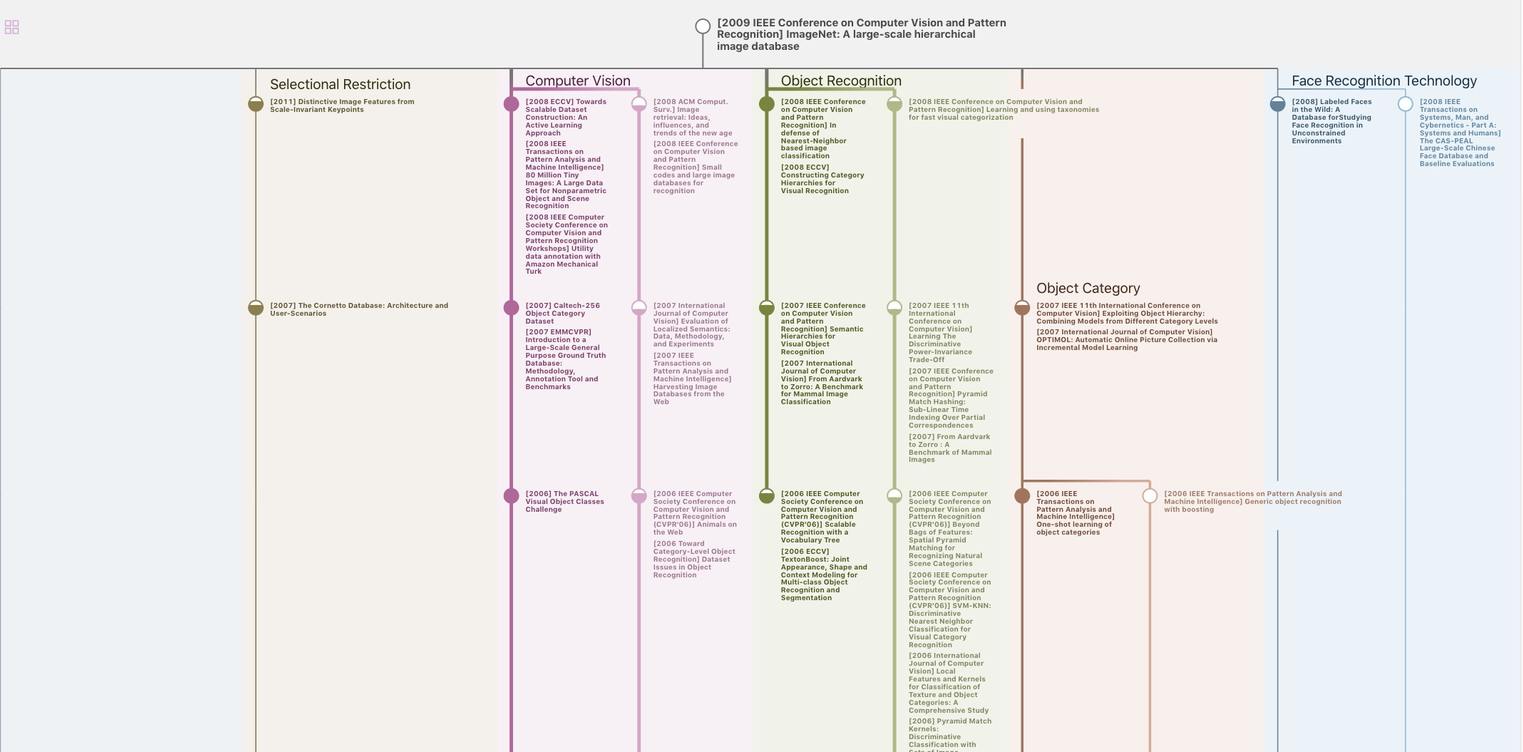
生成溯源树,研究论文发展脉络
Chat Paper
正在生成论文摘要