Machine learning: Predicting hospital length of stay in patients admitted for lupus flares
Lupus(2023)
摘要
Background Although rare, severe systemic lupus erythematosus (SLE) flares requiring hospitalization account for most of the direct costs of SLE care. New machine learning (ML) methods may optimize lupus care by predicting which patients will have a prolonged hospital length of stay (LOS). Our study uses a machine learning approach to predict the LOS in patients admitted for lupus flares and assesses which features prolong LOS. Methods Our study sampled 5831 patients admitted for lupus flares from the National Inpatient Sample Database 2016–2018 and collected 90 demographics and comorbidity features. Four machine learning (ML) models were built (XGBoost, Linear Support Vector Machines, K Nearest Neighbors, and Logistic Regression) to predict LOS, and their performance was evaluated using multiple metrics, including accuracy, receiver operator area under the curve (ROC-AUC), precision-recall area under the curve (PR- AUC), and F1-score. Using the highest-performing model (XGBoost), we assessed the feature importance of our input features using Shapley value explanations (SHAP) to rank their impact on LOS. Results Our XGB model performed the best with a ROC-AUC of 0.87, PR-AUC of 0.61, an F1 score of 0.56, and an accuracy of 95%. The features with the most significant impact on the model were “the need for a central line,” “acute dialysis,” and “acute renal failure.” Other top features include those related to renal and infectious comorbidities. Conclusion Our results were consistent with the established literature and showed promise in ML over traditional methods of predictive analyses, even with rare rheumatic events such as lupus flare hospitalizations.
更多查看译文
关键词
hospital length,patients,learning
AI 理解论文
溯源树
样例
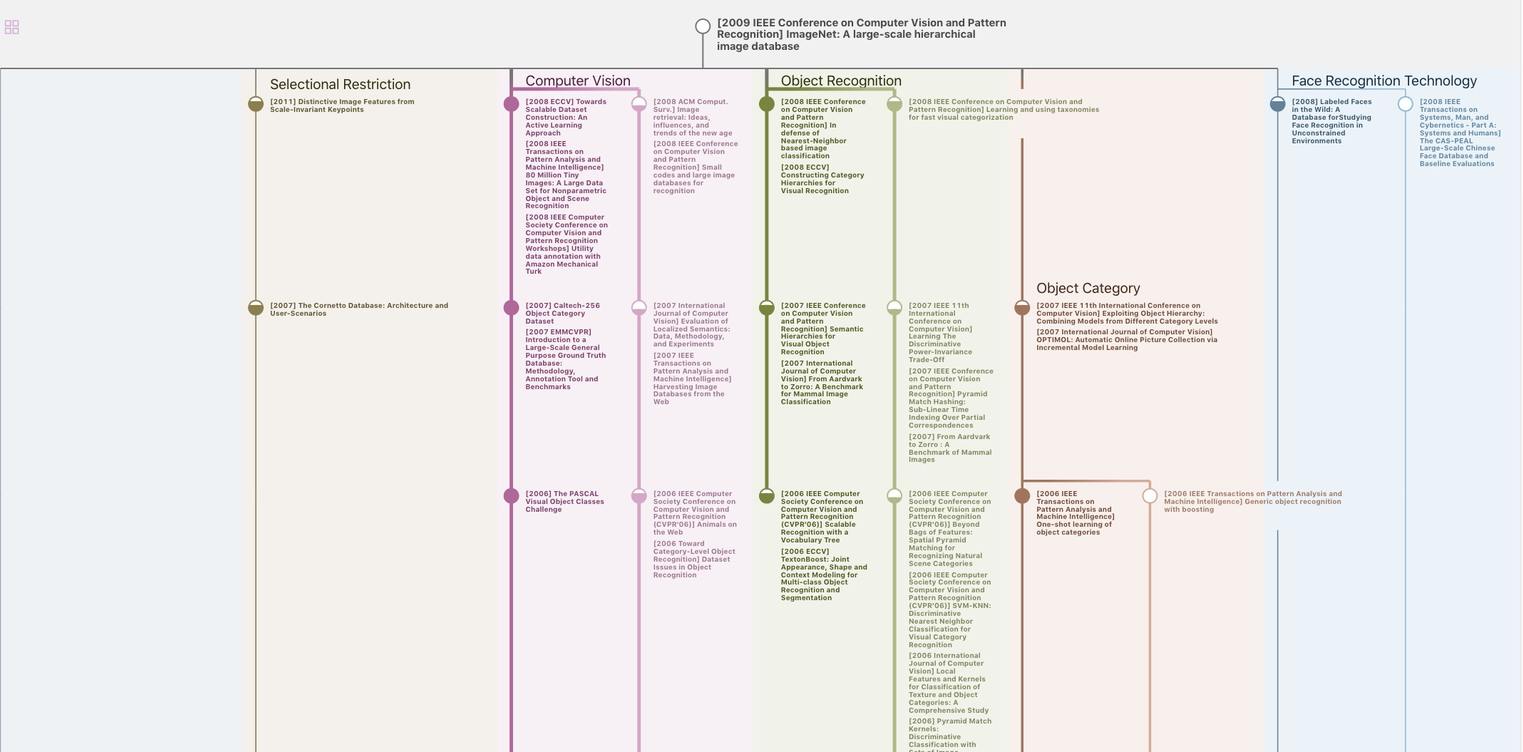
生成溯源树,研究论文发展脉络
Chat Paper
正在生成论文摘要