BioT5: Enriching Cross-modal Integration in Biology with Chemical Knowledge and Natural Language Associations
arXiv (Cornell University)(2023)
摘要
Recent advancements in biological research leverage the integration of molecules, proteins, and natural language to enhance drug discovery. However, current models exhibit several limitations, such as the generation of invalid molecular SMILES, underutilization of contextual information, and equal treatment of structured and unstructured knowledge. To address these issues, we propose $\mathbf{BioT5}$, a comprehensive pre-training framework that enriches cross-modal integration in biology with chemical knowledge and natural language associations. $\mathbf{BioT5}$ utilizes SELFIES for $100%$ robust molecular representations and extracts knowledge from the surrounding context of bio-entities in unstructured biological literature. Furthermore, $\mathbf{BioT5}$ distinguishes between structured and unstructured knowledge, leading to more effective utilization of information. After fine-tuning, BioT5 shows superior performance across a wide range of tasks, demonstrating its strong capability of capturing underlying relations and properties of bio-entities. Our code is available at $\href{https://github.com/QizhiPei/BioT5}{Github}$.
更多查看译文
关键词
chemical,biology,knowledge,cross-modal
AI 理解论文
溯源树
样例
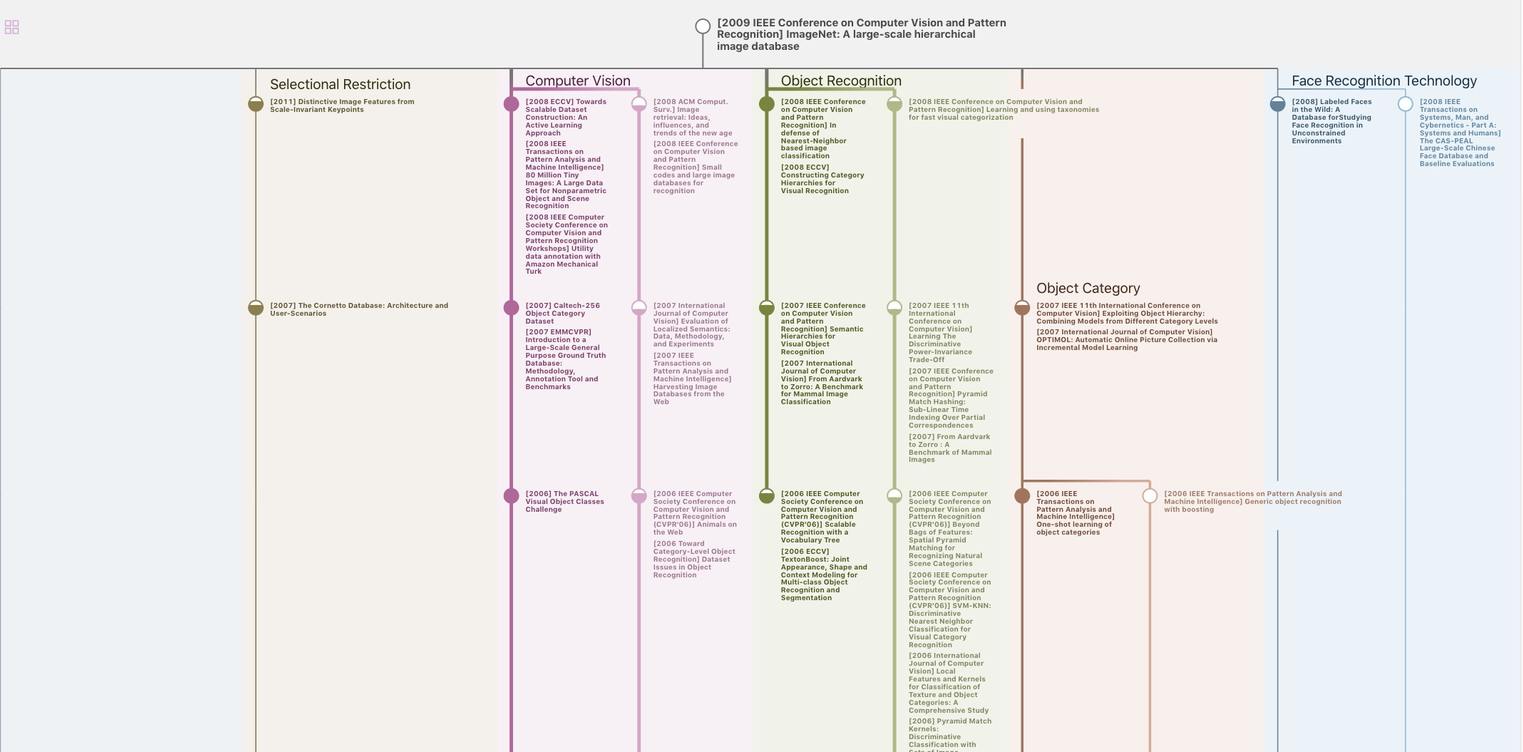
生成溯源树,研究论文发展脉络
Chat Paper
正在生成论文摘要