The Solution for the CVPR2023 NICE Image Captioning Challenge
arXiv (Cornell University)(2023)
摘要
In this paper, we present our solution to the New frontiers for Zero-shot Image Captioning Challenge. Different from the traditional image captioning datasets, this challenge includes a larger new variety of visual concepts from many domains (such as COVID-19) as well as various image types (photographs, illustrations, graphics). For the data level, we collect external training data from Laion-5B, a large-scale CLIP-filtered image-text dataset. For the model level, we use OFA, a large-scale visual-language pre-training model based on handcrafted templates, to perform the image captioning task. In addition, we introduce contrastive learning to align image-text pairs to learn new visual concepts in the pre-training stage. Then, we propose a similarity-bucket strategy and incorporate this strategy into the template to force the model to generate higher quality and more matching captions. Finally, by retrieval-augmented strategy, we construct a content-rich template, containing the most relevant top-k captions from other image-text pairs, to guide the model in generating semantic-rich captions. Our method ranks first on the leaderboard, achieving 105.17 and 325.72 Cider-Score in the validation and test phase, respectively.
更多查看译文
关键词
cvpr2023,challenge,image
AI 理解论文
溯源树
样例
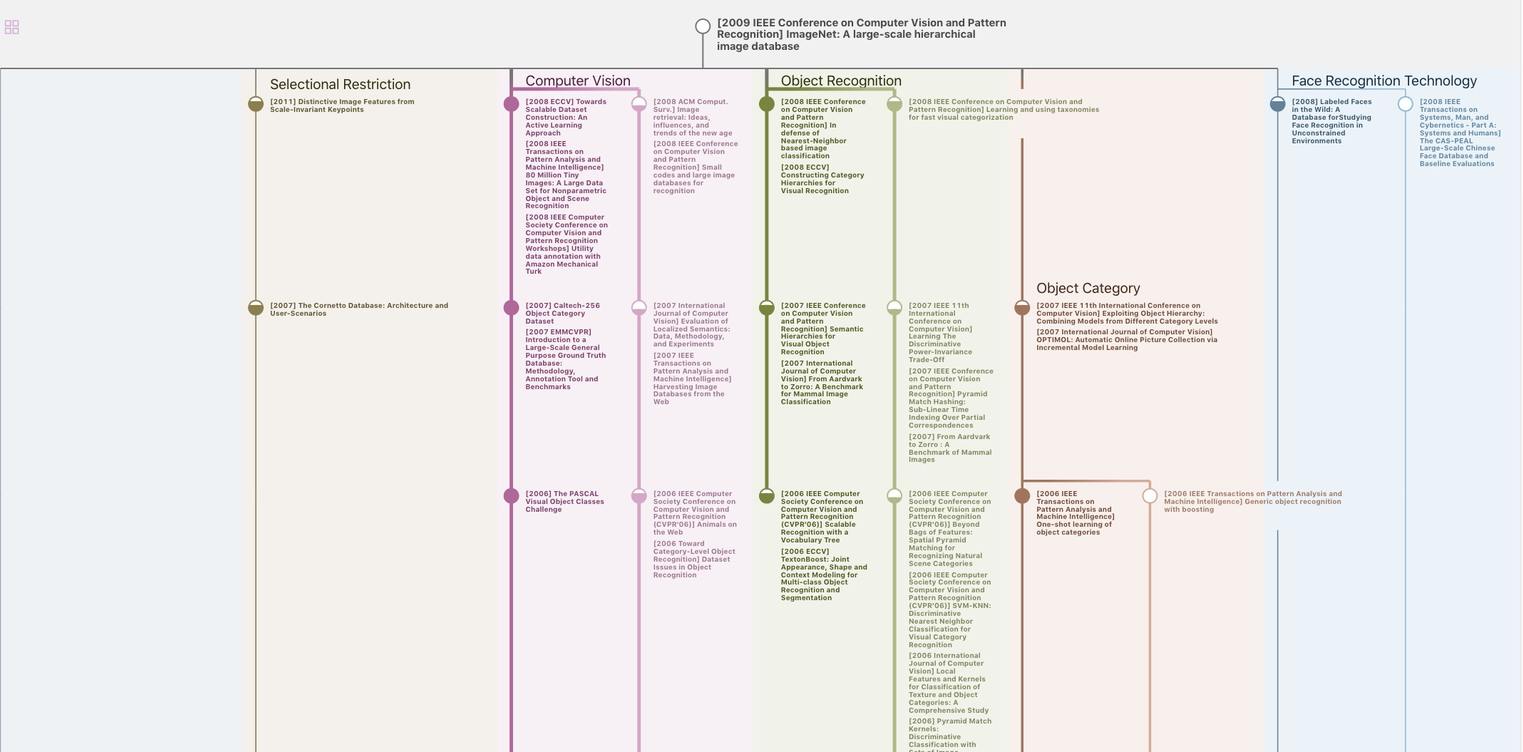
生成溯源树,研究论文发展脉络
Chat Paper
正在生成论文摘要