Conditional Consistency Regularization for Semi-Supervised Multi-Label Image Classification
IEEE TRANSACTIONS ON MULTIMEDIA(2024)
摘要
Consistency regularization has achieved great successes in Semi-Supervised Single-Label Image Classification (SS-SLC) with deep learning models, while few effort has been devoted to Semi-Supervised Multi-Label Image Classification (SS-MLC) with deep learning models. One intuitive solution for introducing consistency regularization to SS-MLC is to regularize model predictions to be invariant to different augmented data of the same input image. However, the solution lacks the consideration of label relations, which are key elements in multi-label image classification. In this article, we go beyond the consistency regularization for multi-view input images, and propose Conditional Consistency Regularization (CCR) that is tailored for SS-MLC. Specifically, for two augmented input images, we make the two model predictions conditioned on different label states (i.e., positive, negative, or unknown for each class). By encouraging the two predictions to be consistent, the model is able to build relations between the given two different label states, which helps to make use of label relations for boosting image classification. The experiments on large-scale real-world SS-MLC benchmarks demonstrate that the proposed method can surpass state-of-the-art methods by a large margin.
更多查看译文
关键词
Predictive models,Image classification,Data models,Motorcycles,Manifolds,Training,Deep learning,semi-supervised multi-label learning,consistency regularization
AI 理解论文
溯源树
样例
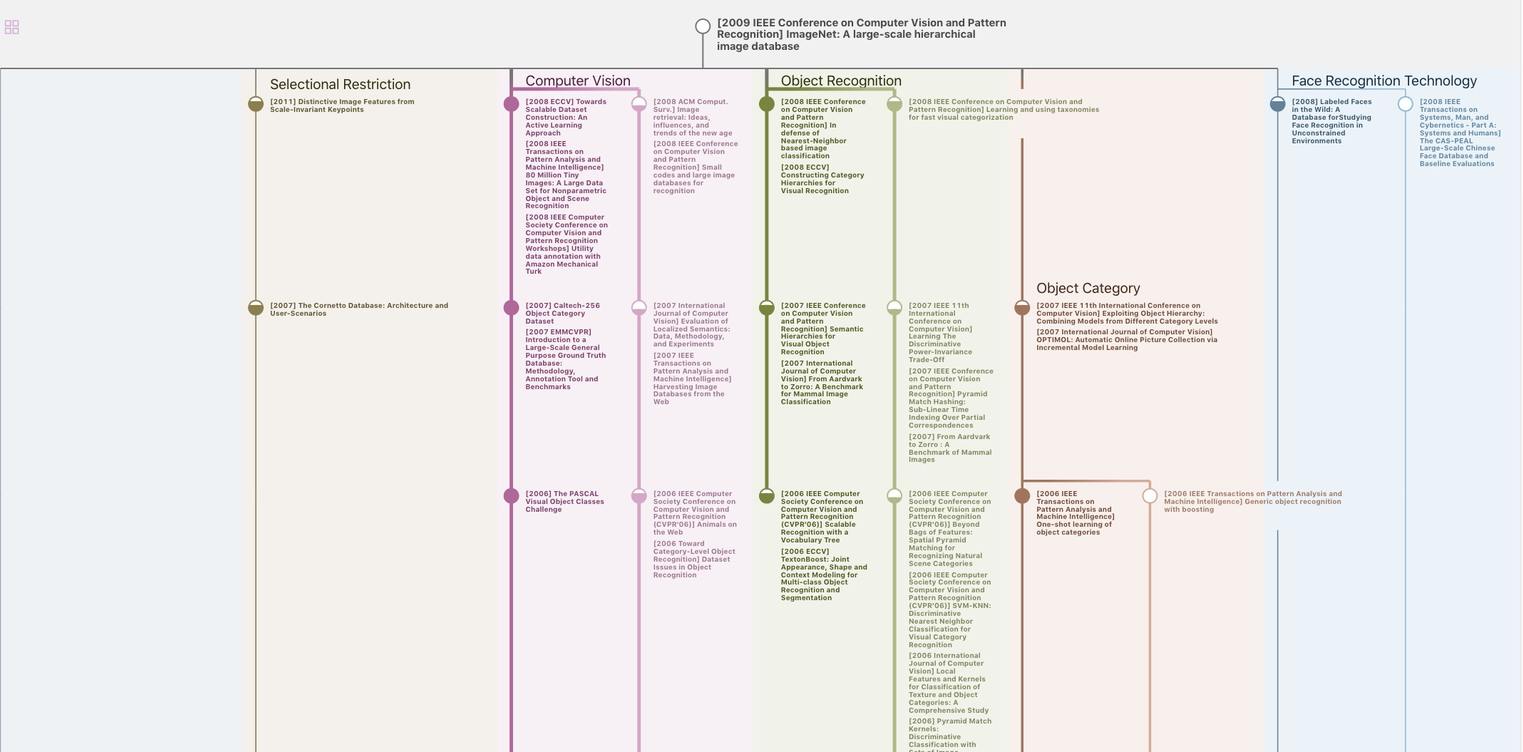
生成溯源树,研究论文发展脉络
Chat Paper
正在生成论文摘要