Using Diversities to Model the Reliability of Two-version Machine Learning Systems
IEEE Transactions on Emerging Topics in Computing(2023)
摘要
The N-version machine learning system (MLS) is an architectural approach to reduce error outputs from a system by redundant configuration using multiple machine learning (ML) modules. Improved system reliability achieved by N-version MLSs inherently depends on how diverse ML models are employed and how diverse input data sets are given. However, neither error input spaces of individual ML models nor input data distributions are obtainable in practice, which is a fundamental barrier to understanding the reliability improvement by N-version architectures. In this paper, we introduce two diversity measures quantifying the similarities of ML models' capabilities and the interdependence of input data sets causing errors, respectively. The defined measures are used to formulate the reliability of an elemental N-version MLS called dependent double-modules double-inputs MLS. The system is assumed to fail when two ML modules output errors simultaneously for the same classification task. The reliabilities of different architecture options for this MLS are comprehensively analyzed through a compact matrix representation form of the proposed reliability model. The theoretical analysis and numerical results show that the architecture exploiting two diversities achieves preferable reliability under reasonable assumptions. Intuitive relations between diversity parameters and architecture reliabilities are also demonstrated through numerical examples.
更多查看译文
关键词
Diversity,Machine learning system,Redundant architecture,Reliability,Software fault-tolerance
AI 理解论文
溯源树
样例
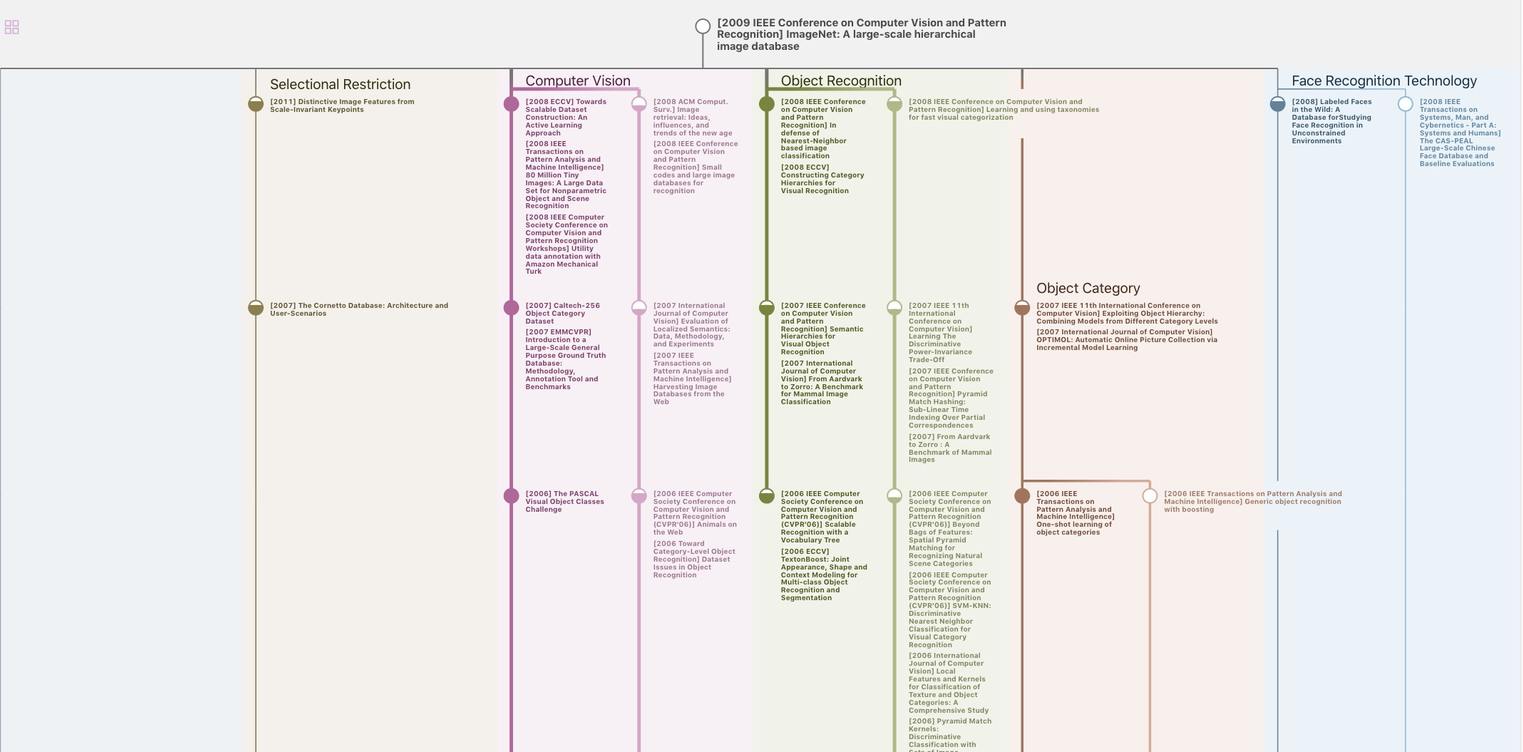
生成溯源树,研究论文发展脉络
Chat Paper
正在生成论文摘要