A customizable framework for multimodal emotion recognition using ensemble of deep neural network models
Multimedia Systems(2023)
摘要
Multimodal emotion recognition of videos of human oration, commonly called opinion videos, has a wide scope of applications across all domains. Here, the speakers express their views or opinions about various topics. This field is being researched by many with the aim of introducing accurate and efficient architectures for the same. This study also carries the same objective while exploring novel concepts in the field of emotion recognition. The proposed framework uses cross-dataset training and testing, so that the resultant architecture and models are unrestricted by the domain of input. It uses benchmark datasets and ensemble learning to make sure that even if the individual models are slightly biased, they can be countered by the learnings of the other models. Therefore, to achieve this objective with the mentioned novelties, three benchmark datasets, ISEAR, RAVDESS, and FER-2013, are used to train independent models for each of the three modalities of text, audio, and images. Another dataset is used in addition to the ISEAR dataset to train the text model. They are then combined and tested on the benchmark multimodal dataset of CMU-MOSEI. For the text analysis model, ELMo embedding and RNN are used, for audio, a simple DNN is used and for image emotion recognition, a 2D CNN is used after pre-processing. They are aggregated using the stacking technique for the final result. The complete architecture can be used as a partially pre-trained algorithm for the prediction of individual modalities, and partially trainable for stacking the results to get efficient emotion prediction based on input quality. The accuracy obtained on the CMU-MOSEI data set is 86.60% and the F1-score for the same is 0.84.
更多查看译文
关键词
CNN, Cross-dataset, Deep neural network, ELMo, Multimodal emotion recognition, RNN, Stacking
AI 理解论文
溯源树
样例
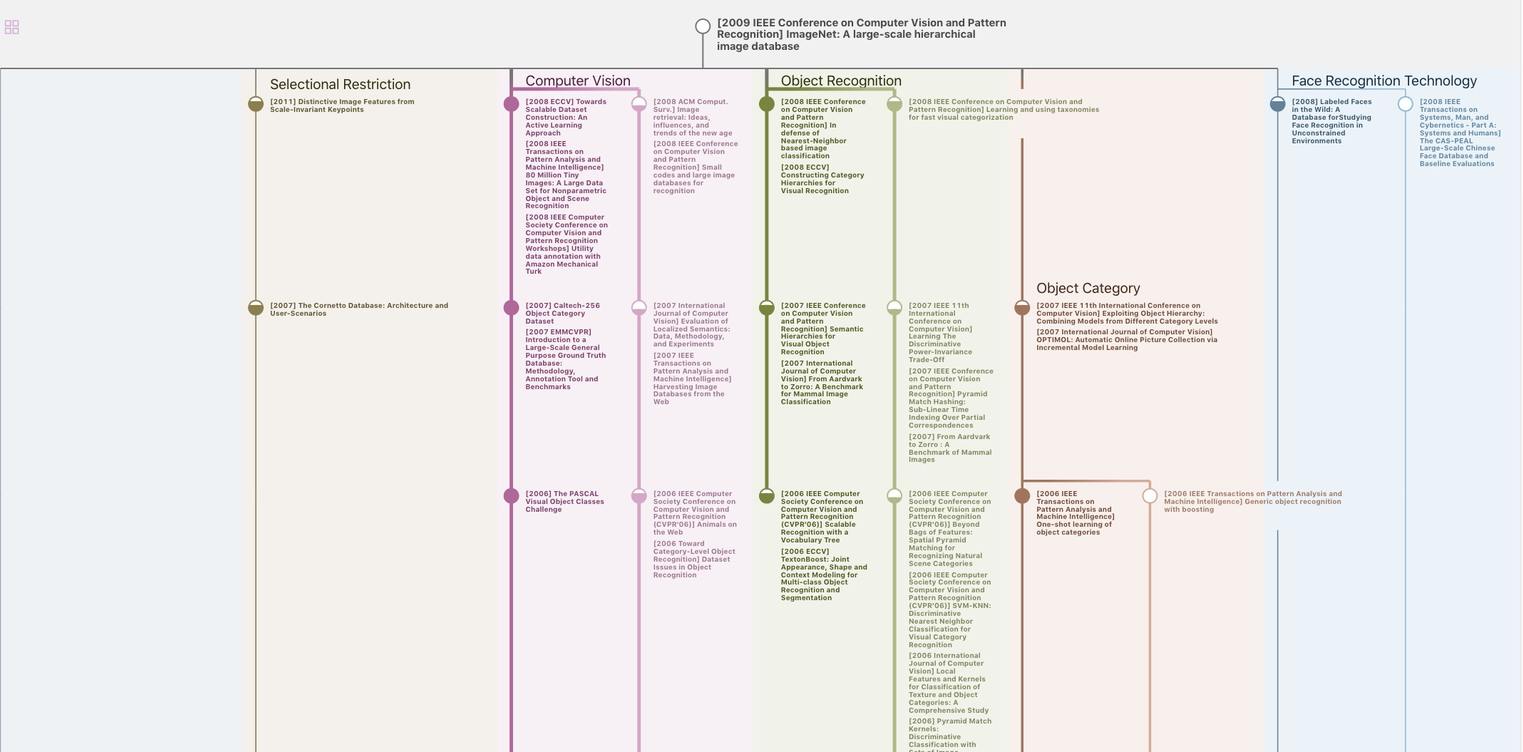
生成溯源树,研究论文发展脉络
Chat Paper
正在生成论文摘要