Learning Image-Adaptive Lookup Tables With Spatial Awareness for Image Harmonization
IEEE Transactions on Consumer Electronics(2023)
摘要
Image harmonization aims to address inharmony between the foreground and background of composite images. Current methods mainly adopt the encoder-decoder reconstruction architecture, which restricts their application to high-resolution images due to heavy resource cost. Inspired by Photoshop, whether we can leverage some adjustment tools to apply on composite images to generate harmonized ones. This paper gives an answer "yes", and explores a new viewpoint to address image harmonization problem by lookup tables (LUTs) rather than reconstruct full images, which saves a lot of memory. Specifically, a deep model is utilized to encode the down-sampled version of the input image as style-relevant weights to fuse multiple basic LUTs into image-adaptive ones, which are employed to transform the global color of the foreground in source high-resolution composite image to be harmonious with the background. Besides, a spatial attention module is applied to learn the spatial map for revising preliminary LUTs transformation as well as raising the local spatial awareness of the image-adaptive LUTs. Extensive experiments show that our method achieves competitive performance both qualitatively and quantitatively with a smaller model size.
更多查看译文
关键词
Image Harmonization,Lookup Tables,Image Composition
AI 理解论文
溯源树
样例
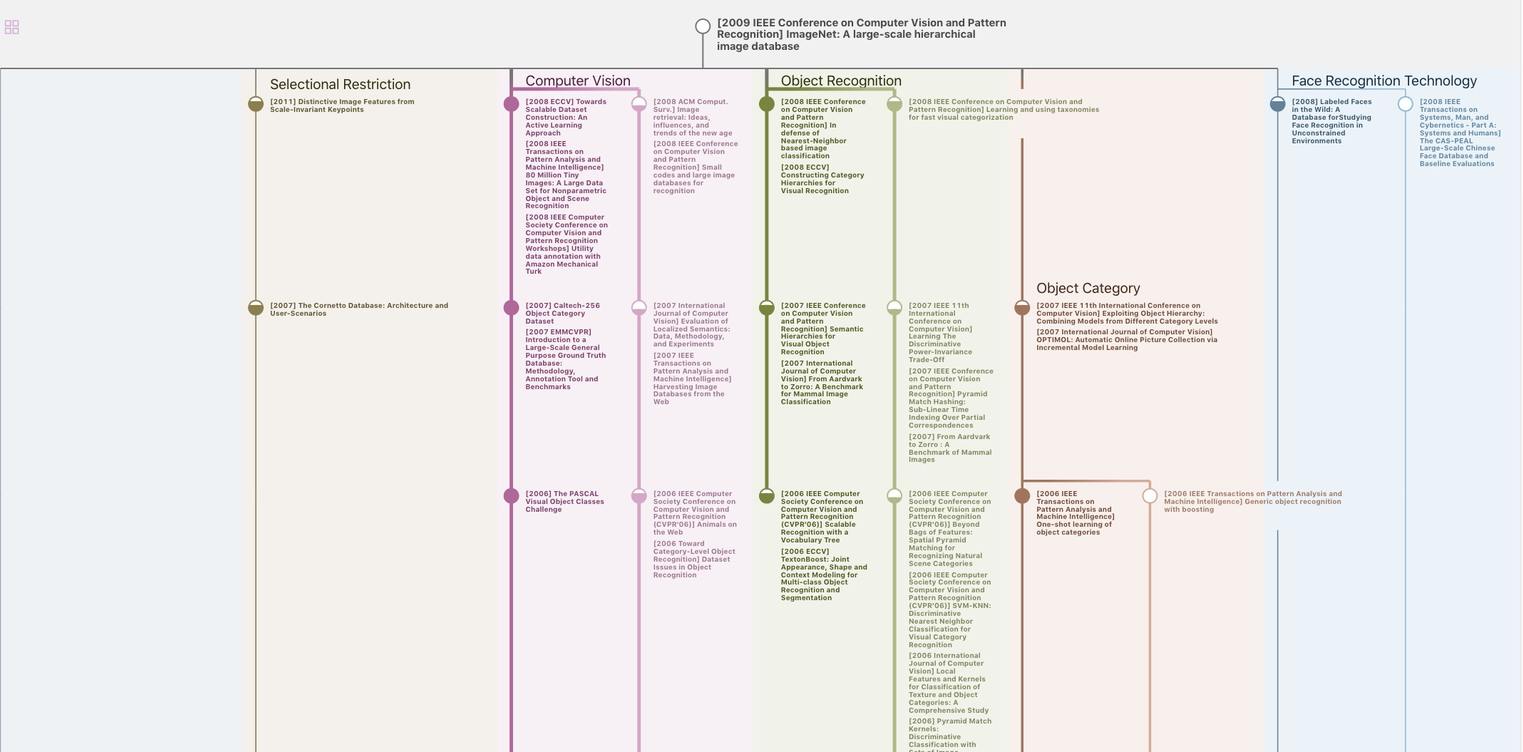
生成溯源树,研究论文发展脉络
Chat Paper
正在生成论文摘要