Partial discharge fault identification of transformer based on CNN-SVM and multi-model fusion
Research Square (Research Square)(2023)
摘要
Abstract Partial discharge pattern recognition has been established as a standard diagnostic tool for monitoring the operation of electrical equipment. Intelligent state recognition is the development trend of transformer state recognition, but the existing intelligent state recognition has the disadvantages of single model and low recognition accuracy. In order to overcome this shortcoming, a multi-dimensional information source transformer partial discharge fault identification method based on D-S evidence theory is proposed. Firstly, wavelet packet decomposition is used to extract the energy features of high frequency partial discharge signal and ultrasonic signal. Then, according to the selected feature set, the convolutional neural network (CNN) model and CNN-SVM model are established respectively. Finally, the Dempster-Shafer(D-S) evidence theory is used to combine the output results of the two signal recognition models. The results show that using the proposed wavelet packet decomposition energy feature set as input vector, the recognition rates of the two signals CNN-SVM models reach 95% and 81.67%, which are 3.33% and 8.34% higher than CNN respectively. The overall performance of D-S evidence theory fusion method is better than that of CNN and CNN-SVM, and the accuracy and consistency are improved by 3.33% and 16.66% respectively. The effectiveness and feasibility of this method are verified.
更多查看译文
关键词
partial discharge fault identification,transformer,cnn-svm,multi-model
AI 理解论文
溯源树
样例
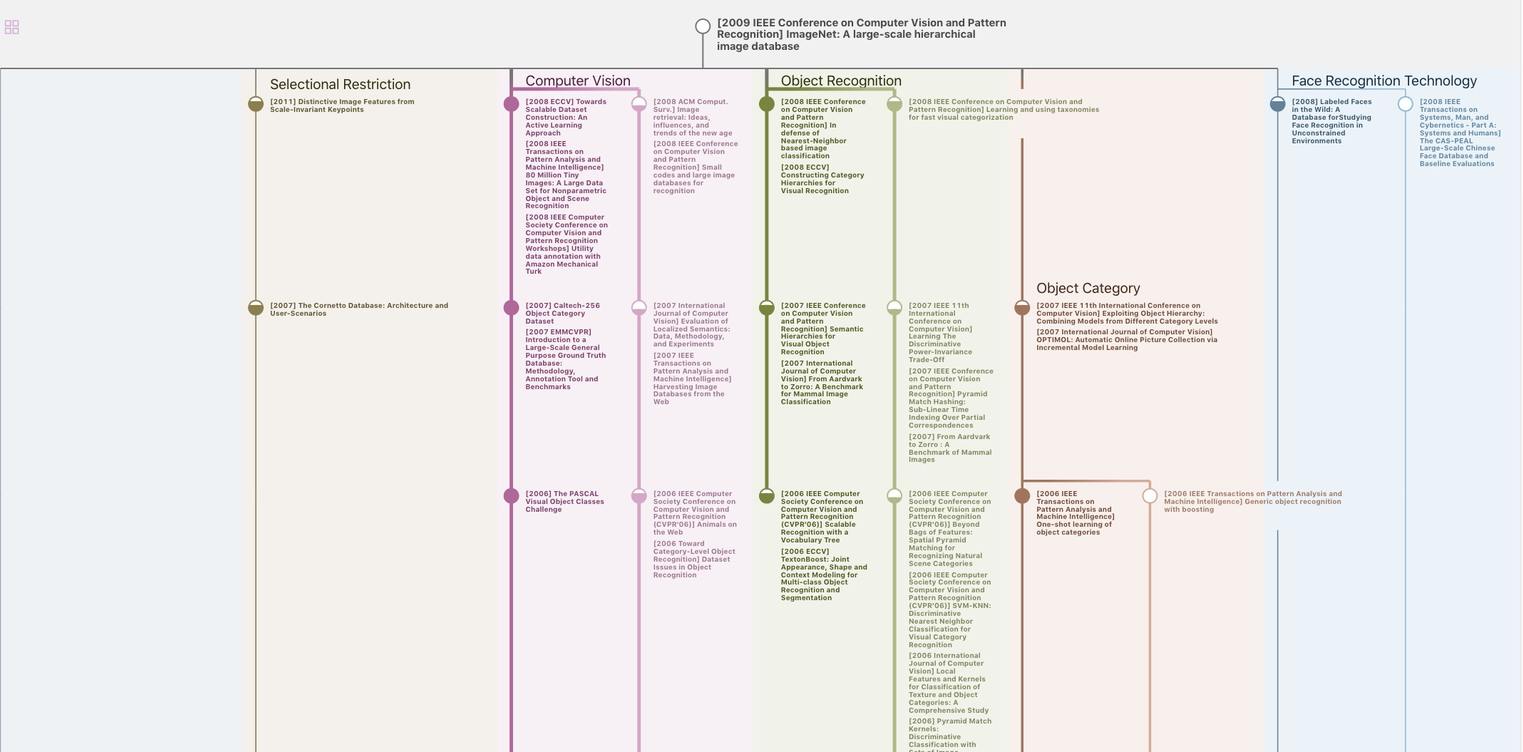
生成溯源树,研究论文发展脉络
Chat Paper
正在生成论文摘要