Transferability and interpretability of the sepsis prediction models in the intensive care unit
medRxiv (Cold Spring Harbor Laboratory)(2021)
摘要
Objective We aimed to develop an early warning system for real-time sepsis prediction in the ICU by equipping with interpretation analysis and transfer learning tools to improve the feasibility to deploy the sepsis prediction system, particularly to target cohorts. Design Retrospective and observational study. Setting Medical Information Mart for Intensive Care (MIMIC) dataset, the private Historical Database of local Ruijin Hospital (HDRJH), and data collected from Ruijin real-world study. Patients 6891 patients from MIMIC dataset and 453 patients from HDRJH for model development and 67 cases from Ruijin real-world data for model evaluation. Interventions None. Measurements and Main Results Light Gradient Boosting Machine (LightGBM) and multilayer perceptron (MLP) were trained on MIMIC dataset and then finetuned on HDRJH using transfer learning technique. Ultimately, the performance of the sepsis prediction system was further evaluated in the real-world study in the ICU of the target Ruijin Hospital. The area under the receiver operating characteristic curves (AUCs) for LightGBM and MLP models derived from MIMIC were 0.98–0.98 and 0.95–0.96 respectively on MIMIC dataset, and, in comparison, 0.82–0.86 and 0.84–0.87 respectively on HDRJH, from 1–5h preceding. After transfer learning and ensemble learning, the AUCs of the final ensemble model were enhanced to 0.94–0.94 on HDRJH and to 0.86–0.9 in the real-world study in the ICU of the target Ruijin Hospital. In addition, the Shapley additive explanation (SHAP) analysis illustrated the importance of age, antibiotics, net balance, and ventilation for sepsis prediction, making the model interpretable. Conclusions Our machine learning model allows accurate real-time prediction of sepsis within 5-h preceding. Transfer learning can effectively improve the feasibility to deploy the prediction model in the target cohort, effectively ameliorating the model performance for external validation. SHAP analysis may illuminate the importance of optimizing antibiotic use and restricting fluid management. Trial registration NCT05088850 (retrospectively registered). Key Points Question We aimed to develop an early warning system for real-time sepsis prediction in the ICU and to improve the feasibility to deploy the system to target cohorts. Findings Transfer learning technique effectively enhanced the AUCs for LightGBM and MLP models on the target cohort, HDRJH, from 0.82–0.86 and 0.84–0.87 to 0.93-0.94 and 0.92-0.93 for 1-5 hour preceding. Additionally, SHAP analysis illuminated the importance of optimizing antibiotic use and restricting fluid management. Meaning Transfer learning can improve the feasibility to deploy the prediction model to the target cohort, and SHAP analysis made the prediction model interpretable.
更多查看译文
关键词
sepsis prediction models,interpretability
AI 理解论文
溯源树
样例
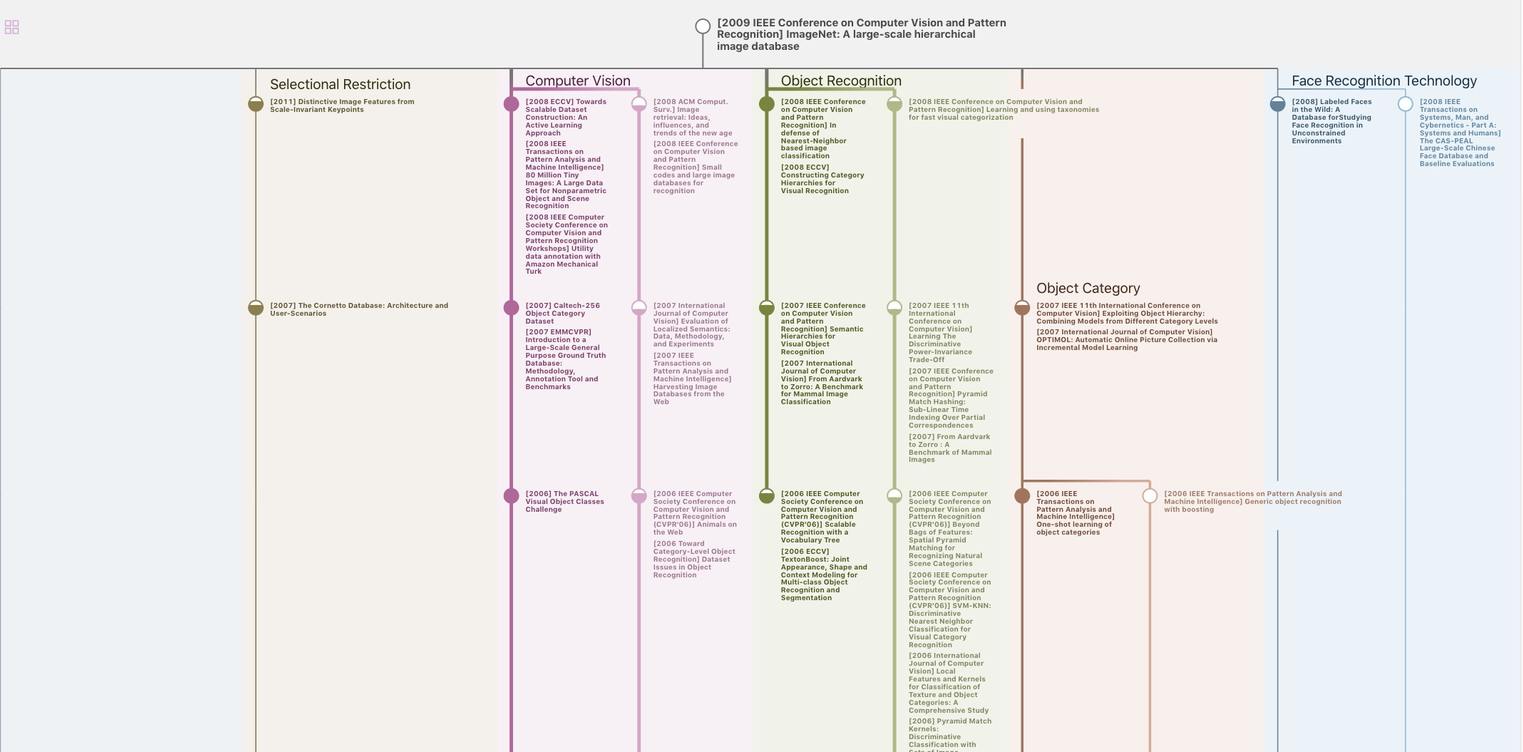
生成溯源树,研究论文发展脉络
Chat Paper
正在生成论文摘要