Interactive Joint Planning for Autonomous Vehicles
IEEE ROBOTICS AND AUTOMATION LETTERS(2024)
摘要
In highly interactive driving scenarios, the actions of one agent greatly influence those of its neighbors. Planning safe motions for autonomous vehicles (AVs) in such interactive environments, therefore, requires reasoning about the impact of the ego's intended motion plan on nearby agents' behavior. Deep-learning-based models have recently achieved considerable success in trajectory prediction and many models in the literature allow for ego-conditioned prediction. However, leveraging ego-conditioned prediction remains challenging in downstream planning due to the complex nature of neural networks, limiting the planner structure to simple types, e.g., sampling-based planners. Despite the ability of gradient-based planning algorithms, such as model predictive control (MPC), to generate fine-grained high-quality motion plans, it is difficult for them to leverage ego-conditioned prediction due to their iterative nature and need for gradients. We present Interactive Joint Planning (IJP), which bridges MPC with learned prediction models in a computationally scalable manner to provide us with the best of both the worlds. In particular, IJP optimizes over the joint behavior of the ego and the surrounding agents and leverages deep-learned prediction models as prediction priors that the join trajectory optimization tries to stay close to. Furthermore, by leveraging free-end homotopy classes-a novel concept that we introduce in this letter-IJP efficiently searches over diverse motion plans. Closed-loop simulation results show that IJP significantly outperforms baselines without joint optimization or running sampling-based planning.
更多查看译文
关键词
Autonomous vehicle navigation,machine learning for robot control,integrated planning and learning
AI 理解论文
溯源树
样例
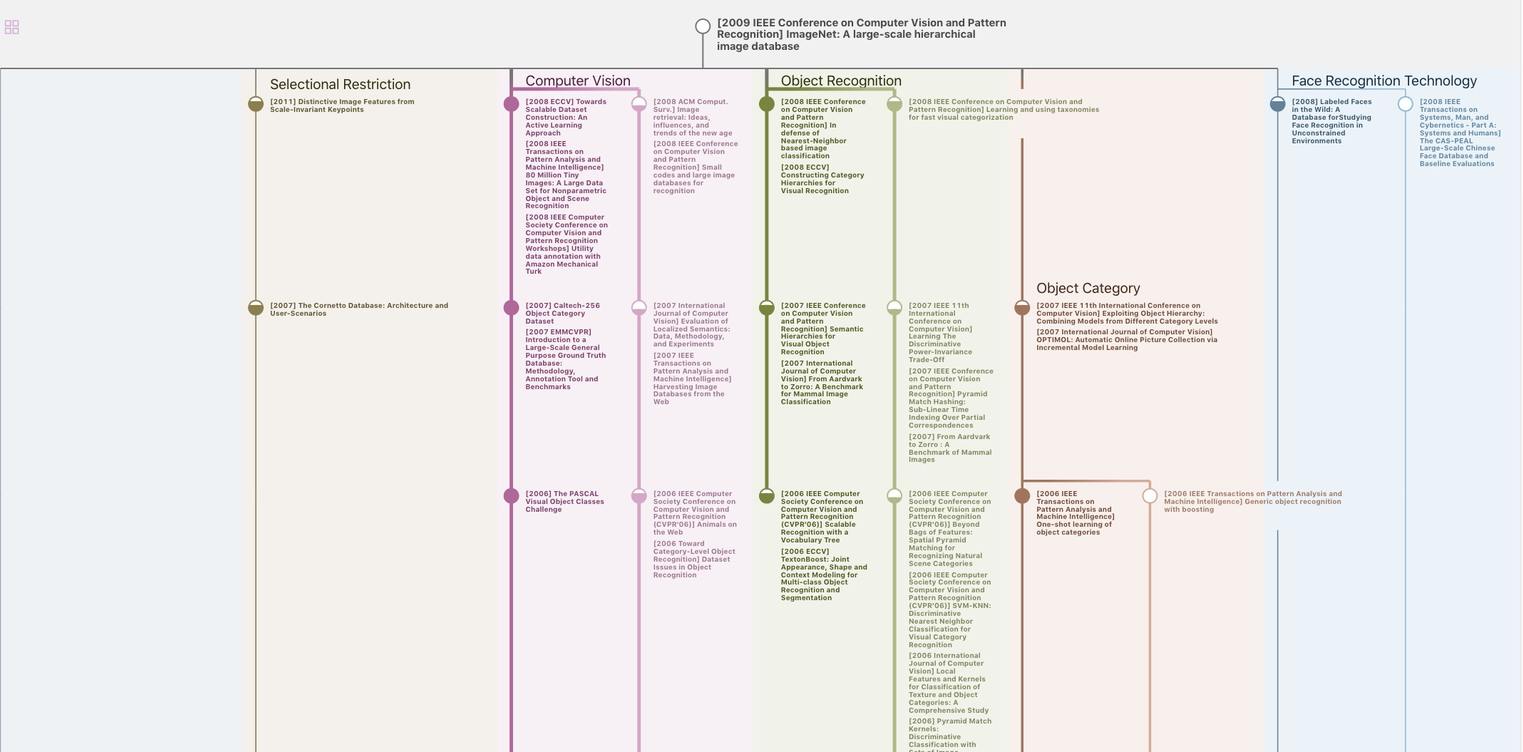
生成溯源树,研究论文发展脉络
Chat Paper
正在生成论文摘要