Label Shift Estimators for Non-Ignorable Missing Data
arXiv (Cornell University)(2023)
摘要
We consider the problem of estimating the mean of a random variable Y subject to non-ignorable missingness, i.e., where the missingness mechanism depends on Y . We connect the auxiliary proxy variable framework for non-ignorable missingness (West and Little, 2013) to the label shift setting (Saerens et al., 2002). Exploiting this connection, we construct an estimator for non-ignorable missing data that uses high-dimensional covariates (or proxies) without the need for a generative model. In synthetic and semi-synthetic experiments, we study the behavior of the proposed estimator, comparing it to commonly used ignorable estimators in both well-specified and misspecified settings. Additionally, we develop a score to assess how consistent the data are with the label shift assumption. We use our approach to estimate disease prevalence using a large health survey, comparing ignorable and non-ignorable approaches. We show that failing to account for non-ignorable missingness can have profound consequences on conclusions drawn from non-representative samples.
更多查看译文
关键词
label,data,non-ignorable
AI 理解论文
溯源树
样例
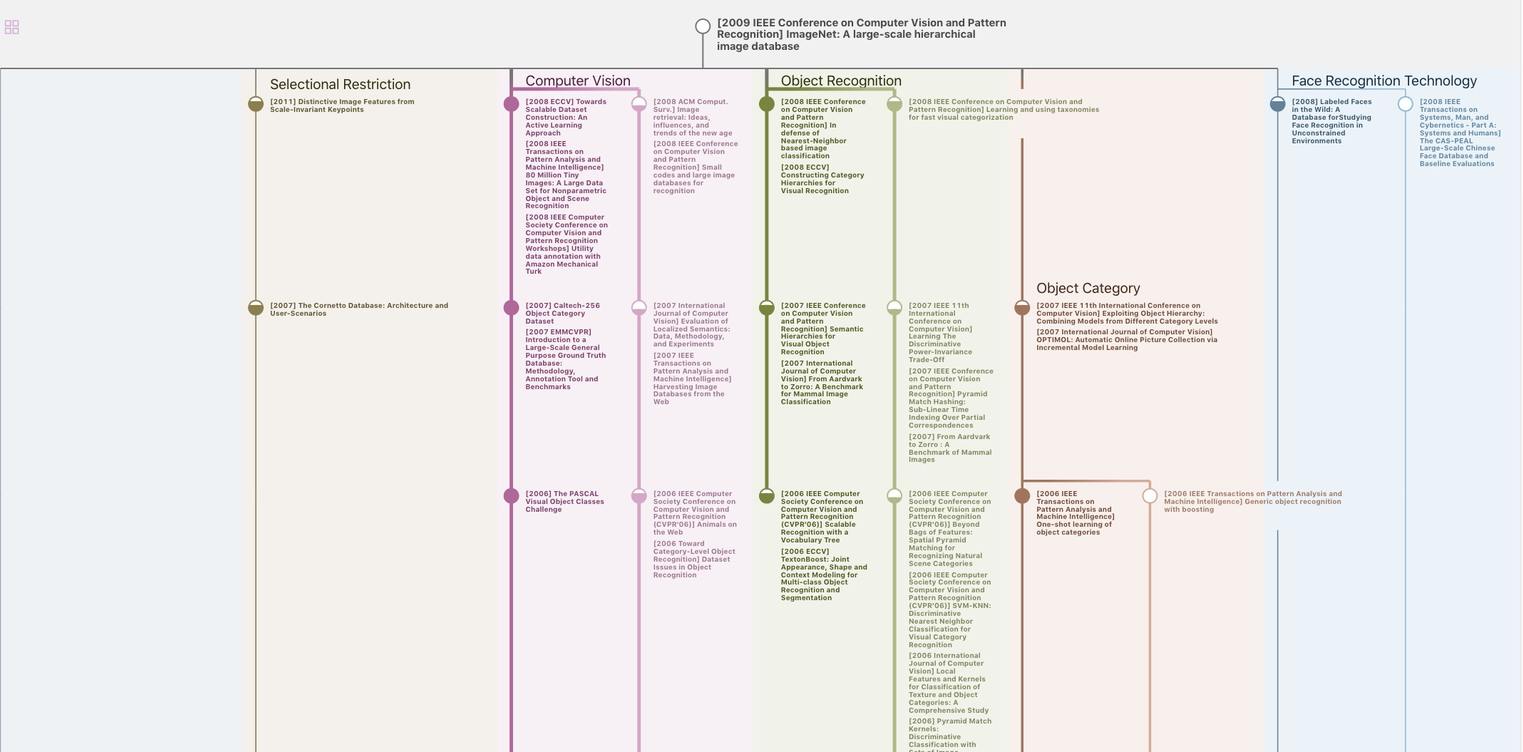
生成溯源树,研究论文发展脉络
Chat Paper
正在生成论文摘要