TabAttention: Learning Attention Conditionally on Tabular Data
MEDICAL IMAGE COMPUTING AND COMPUTER ASSISTED INTERVENTION, MICCAI 2023, PT VII(2023)
摘要
Medical data analysis often combines both imaging and tabular data processing using machine learning algorithms. While previous studies have investigated the impact of attention mechanisms on deep learning models, few have explored integrating attention modules and tabular data. In this paper, we introduce TabAttention, a novel module that enhances the performance of Convolutional Neural Networks (CNNs) with an attention mechanism that is trained conditionally on tabular data. Specifically, we extend the Convolutional Block Attention Module to 3D by adding a Temporal Attention Module that uses multihead self-attention to learn attention maps. Furthermore, we enhance all attention modules by integrating tabular data embeddings. Our approach is demonstrated on the fetal birth weight (FBW) estimation task, using 92 fetal abdominal ultrasound video scans and fetal biometry measurements. Our results indicate that TabAttention outperforms clinicians and existing methods that rely on tabular and/or imaging data for FBW prediction. This novel approach has the potential to improve computer-aided diagnosis in various clinical workflows where imaging and tabular data are combined. We provide a source code for integrating TabAttention in CNNs at https://github.com/SanoScience/Tab-Attention.
更多查看译文
关键词
Attention,Fetal Ultrasound,Tabular Data
AI 理解论文
溯源树
样例
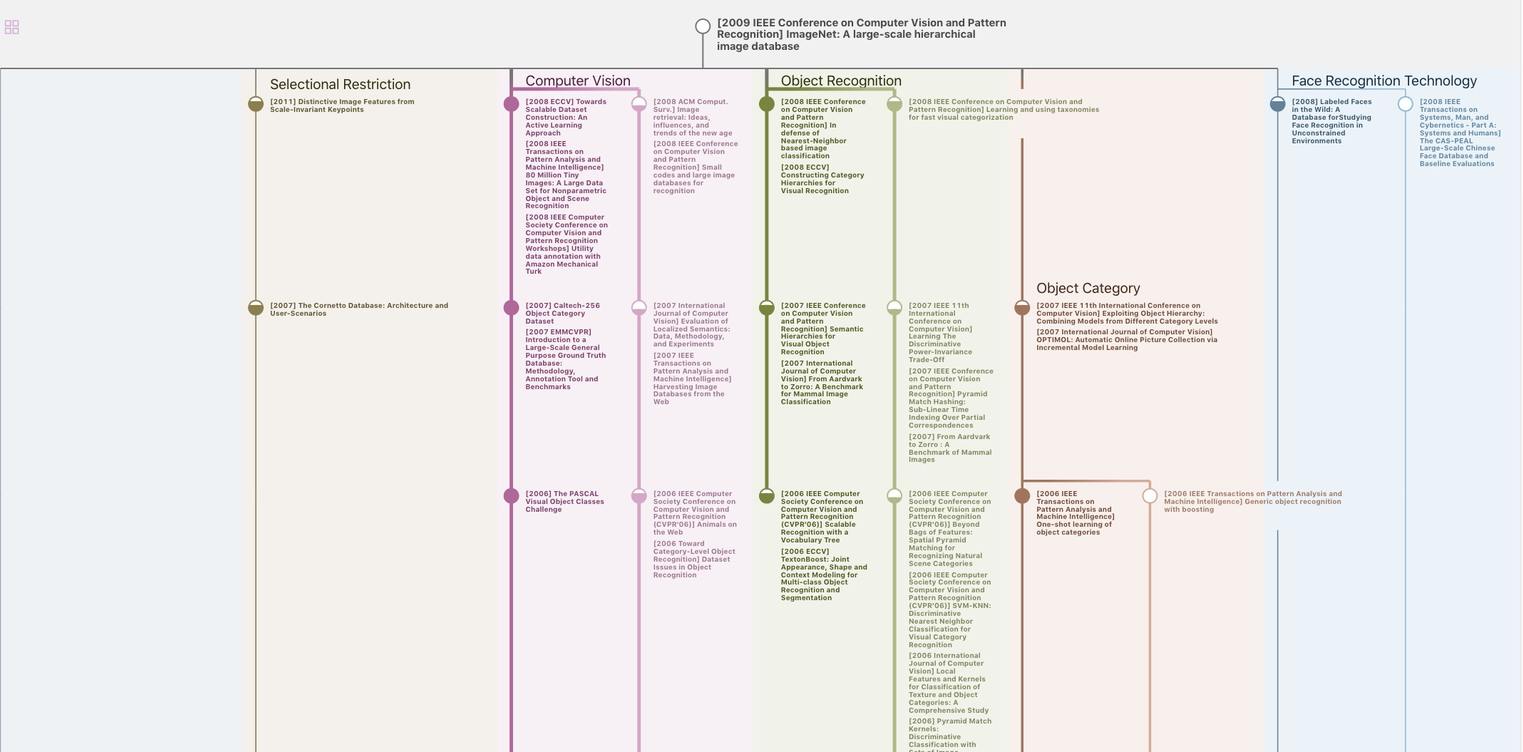
生成溯源树,研究论文发展脉络
Chat Paper
正在生成论文摘要