FaultSeg Swin-UNETR: Transformer-Based Self-Supervised Pretraining Model for Fault Recognition
CoRR(2023)
摘要
This paper introduces an approach to enhance seismic fault recognition
through self-supervised pretraining. Seismic fault interpretation holds great
significance in the fields of geophysics and geology. However, conventional
methods for seismic fault recognition encounter various issues, including
dependence on data quality and quantity, as well as susceptibility to
interpreter subjectivity. Currently, automated fault recognition methods
proposed based on small synthetic datasets experience performance degradation
when applied to actual seismic data. To address these challenges, we have
introduced the concept of self-supervised learning, utilizing a substantial
amount of relatively easily obtainable unlabeled seismic data for pretraining.
Specifically, we have employed the Swin Transformer model as the core network
and employed the SimMIM pretraining task to capture unique features related to
discontinuities in seismic data. During the fine-tuning phase, inspired by edge
detection techniques, we have also refined the structure of the Swin-UNETR
model, enabling multiscale decoding and fusion for more effective fault
detection. Experimental results demonstrate that our proposed method attains
state-of-the-art performance on the Thebe dataset, as measured by the OIS and
ODS metrics.
更多查看译文
关键词
faultseg recognition,pretraining model
AI 理解论文
溯源树
样例
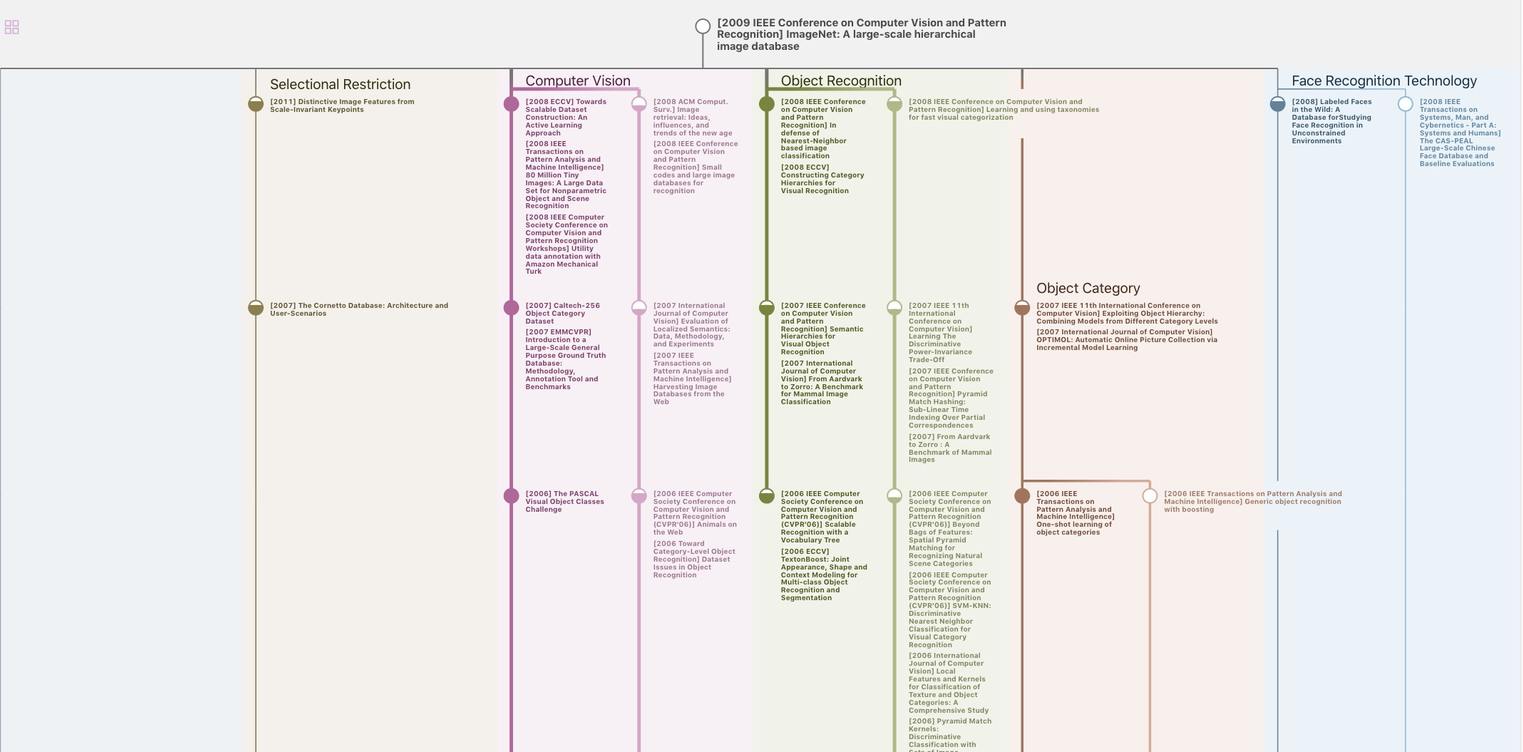
生成溯源树,研究论文发展脉络
Chat Paper
正在生成论文摘要