CEFL: Carbon-Efficient Federated Learning
CoRR(2023)
摘要
Federated Learning (FL) distributes machine learning (ML) training across many edge devices to reduce data transfer overhead and protect data privacy. Since FL model training may span millions of devices and is thus resource-intensive, prior work has focused on improving its resource efficiency to optimize time-to-accuracy. However, prior work generally treats all resources the same, while, in practice, they may incur widely different costs, which instead motivates optimizing cost-to-accuracy. To address the problem, we design CEFL, which uses adaptive cost-aware client selection policies to optimize an arbitrary cost metric when training FL models. Our policies extend and combine prior work on utility-based client selection and critical learning periods by making them cost-aware. We demonstrate CEFL by designing carbon-efficient FL, where energy's carbon-intensity is the cost, and show that it i) reduces carbon emissions by 93\% and reduces training time by 50% compared to random client selection and ii) reduces carbon emissions by 80%, while only increasing training time by 38%, compared to a state-of-the-art approach that optimizes training time.
更多查看译文
关键词
federated learning,carbon-efficient
AI 理解论文
溯源树
样例
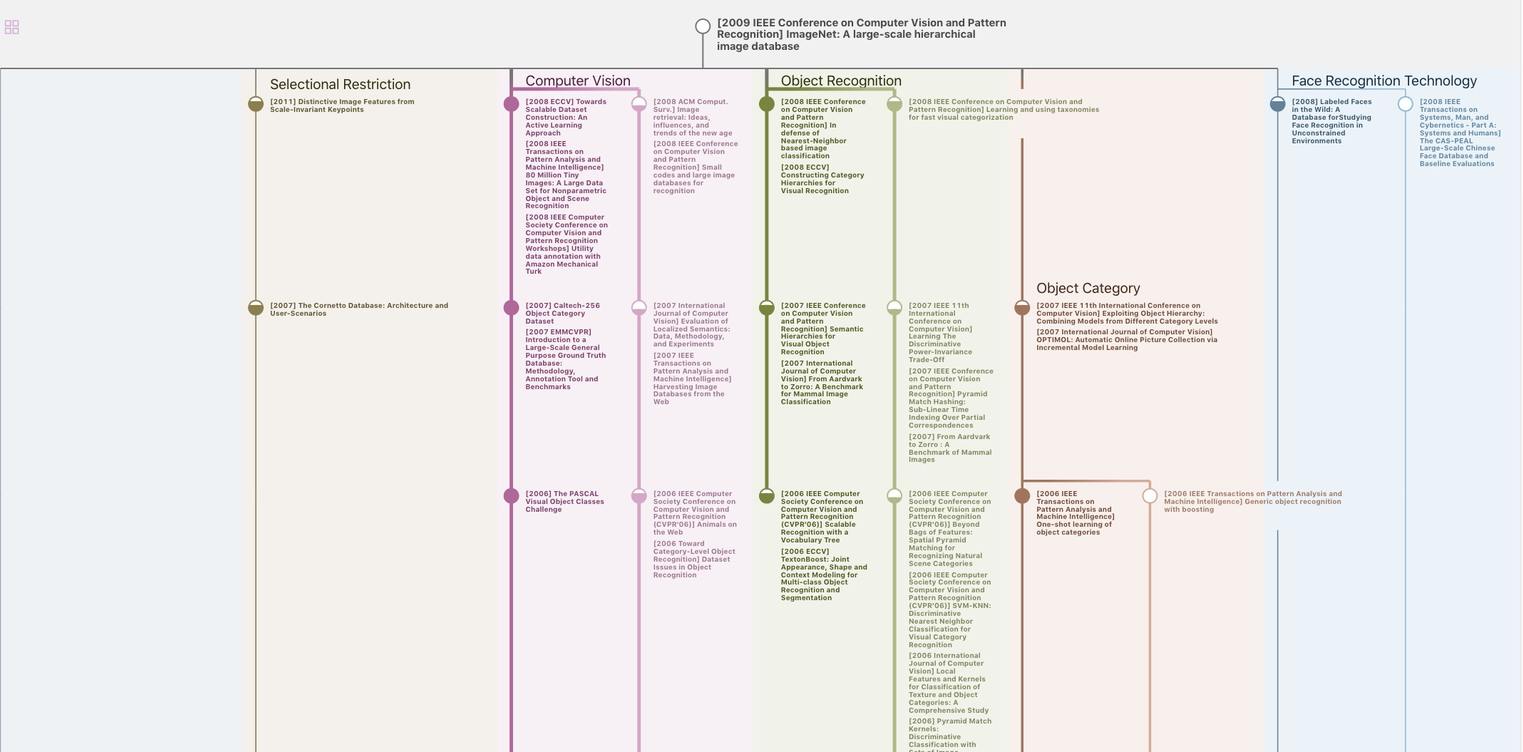
生成溯源树,研究论文发展脉络
Chat Paper
正在生成论文摘要