A hierarchy of eigencomputations for polynomial optimization on the sphere
CoRR(2023)
摘要
We introduce a convergent hierarchy of lower bounds on the minimum value of a real homogeneous polynomial over the sphere. The main practical advantage of our hierarchy over the sum-of-squares (SOS) hierarchy is that the lower bound at each level of our hierarchy is obtained by a minimum eigenvalue computation, as opposed to the full semidefinite program (SDP) required at each level of SOS. In practice, this allows us to go to much higher levels than are computationally feasible for the SOS hierarchy. For both hierarchies, the underlying space at the $k$-th level is the set of homogeneous polynomials of degree $2k$. We prove that our hierarchy converges as $O(1/k)$ in the level $k$, matching the best-known convergence of the SOS hierarchy when the number of variables $n$ is less than the half-degree $d$ (the best-known convergence of SOS when $n \geq d$ is $O(1/k^2)$). More generally, we introduce a convergent hierarchy of minimum eigenvalue computations for minimizing the inner product between a real tensor and an element of the spherical Segre-Veronese variety, with similar convergence guarantees. As examples, we obtain hierarchies for computing the (real) tensor spectral norm, and for minimizing biquadratic forms over the sphere. Hierarchies of eigencomputations for more general constrained polynomial optimization problems are discussed.
更多查看译文
关键词
eigencomputations,polynomial optimization,sphere,hierarchy
AI 理解论文
溯源树
样例
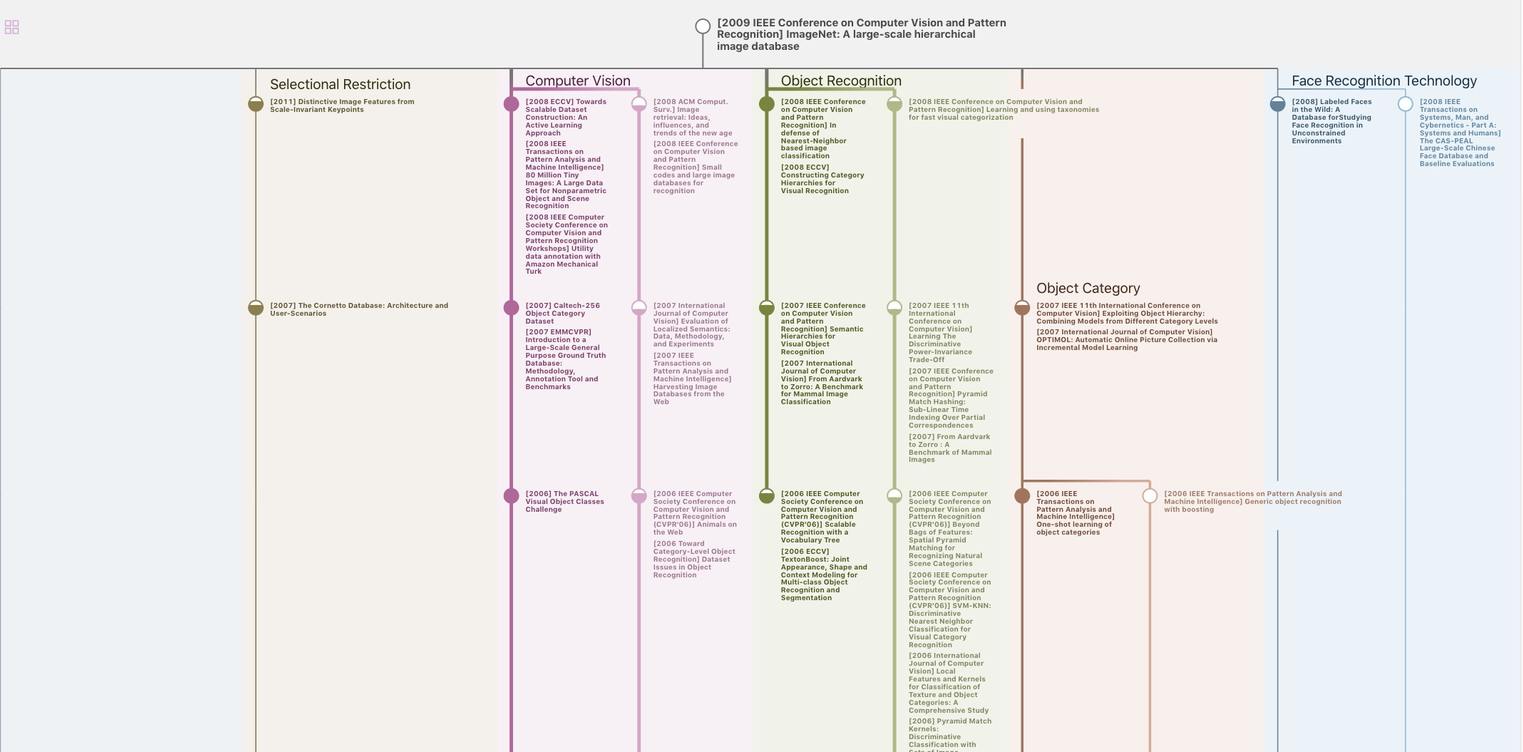
生成溯源树,研究论文发展脉络
Chat Paper
正在生成论文摘要