ZeroQuant-HERO: Hardware-Enhanced Robust Optimized Post-Training Quantization Framework for W8A8 Transformers
CoRR(2023)
摘要
Quantization techniques are pivotal in reducing the memory and computational demands of deep neural network inference. Existing solutions, such as ZeroQuant, offer dynamic quantization for models like BERT and GPT but overlook crucial memory-bounded operators and the complexities of per-token quantization. Addressing these gaps, we present a novel, fully hardware-enhanced robust optimized post-training W8A8 quantization framework, ZeroQuant-HERO. This framework uniquely integrates both memory bandwidth and compute-intensive operators, aiming for optimal hardware performance. Additionally, it offers flexibility by allowing specific INT8 modules to switch to FP16/BF16 mode, enhancing accuracy.
更多查看译文
关键词
quantization framework,transformers,zeroquant-hero,hardware-enhanced,post-training
AI 理解论文
溯源树
样例
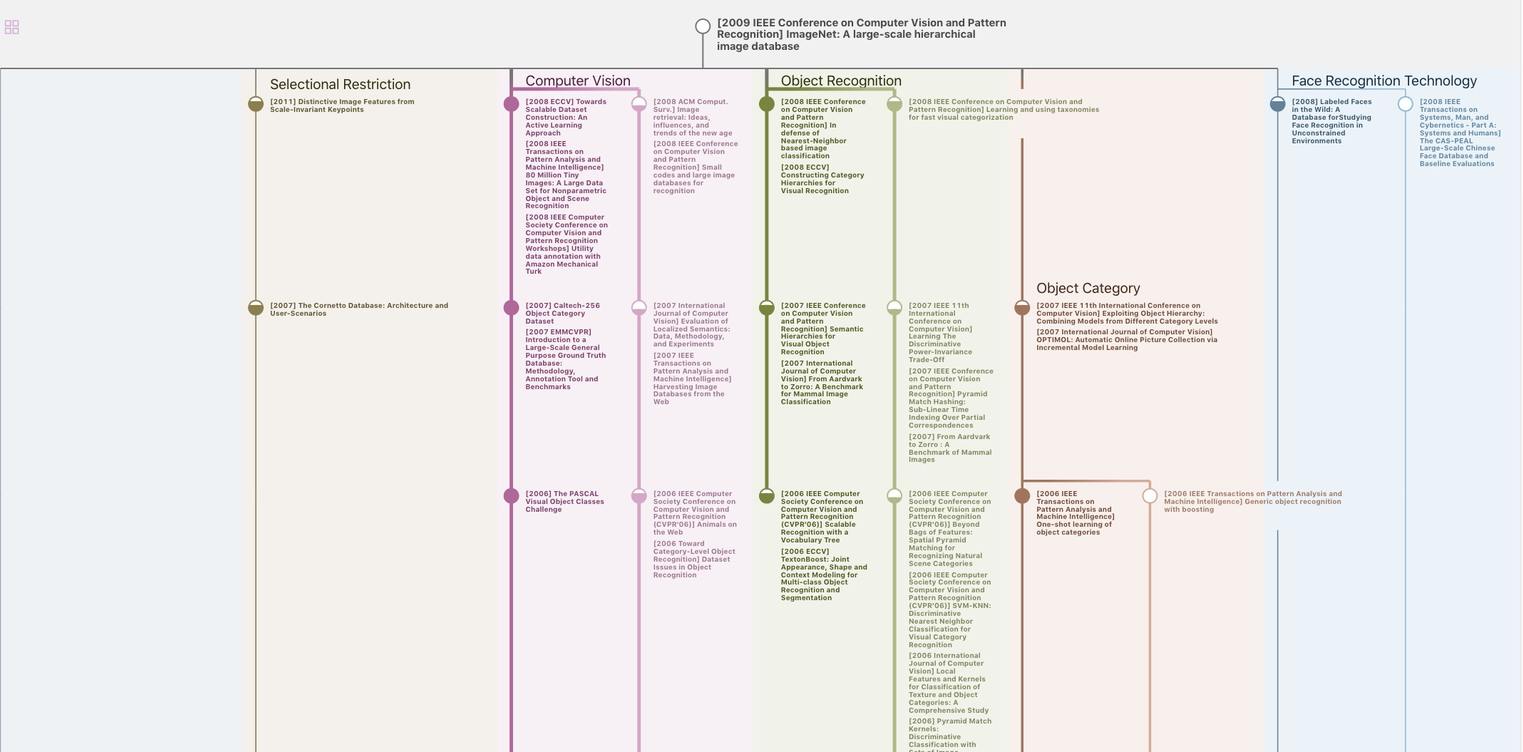
生成溯源树,研究论文发展脉络
Chat Paper
正在生成论文摘要