Identification of chrysanthemum using hyperspectral imaging based on few-shot class incremental learning
COMPUTERS AND ELECTRONICS IN AGRICULTURE(2023)
摘要
Chrysanthemum, a traditional Chinese medicine, possesses diverse pharmacological effects with a myriad of origins and varieties. Due to the difficulty of acquiring and modeling all Chrysanthemum varieties comprehensively, it becomes imperative to establish models based on the available samples in order to swiftly identify newly emerging Chrysanthemum categories from a limited dataset. In this study, hyperspectral imaging combined with deep learning was exploited for the classification of fourteen Chrysanthemum categories by origin and variety. Leveraging the convolutional neural network, the few-shot class-incremental learning (class-IL) method was applied to the detection of few-shot Chrysanthemum categories. By employing a Replay training strategy, the challenges associated with severely sample-limited and unbalanced classes can be effectively addressed. When incrementally expanding from four to fourteen categories, with each new category consisting of only 30 samples, the achieved accuracy on the test dataset reached 80.13 %. This remarkable performance exhibited a narrow margin of 15.75 % compared to conventional supervised learning, which utilized an incremental training sample size nearly 100 times larger. This approach consistently outperforms conventional supervised learning methods, thereby showcasing its remarkable scalability. It facilitates the practical implementation of few-shot learning and deep learning models, providing a substantiated framework to tackle real-world scenarios in various domains using hyperspectral imaging and related techniques.
更多查看译文
关键词
Near-infrared hyperspectral imaging,Chrysanthemum,Few-shot learning,Transfer learning,Class-incremental learning
AI 理解论文
溯源树
样例
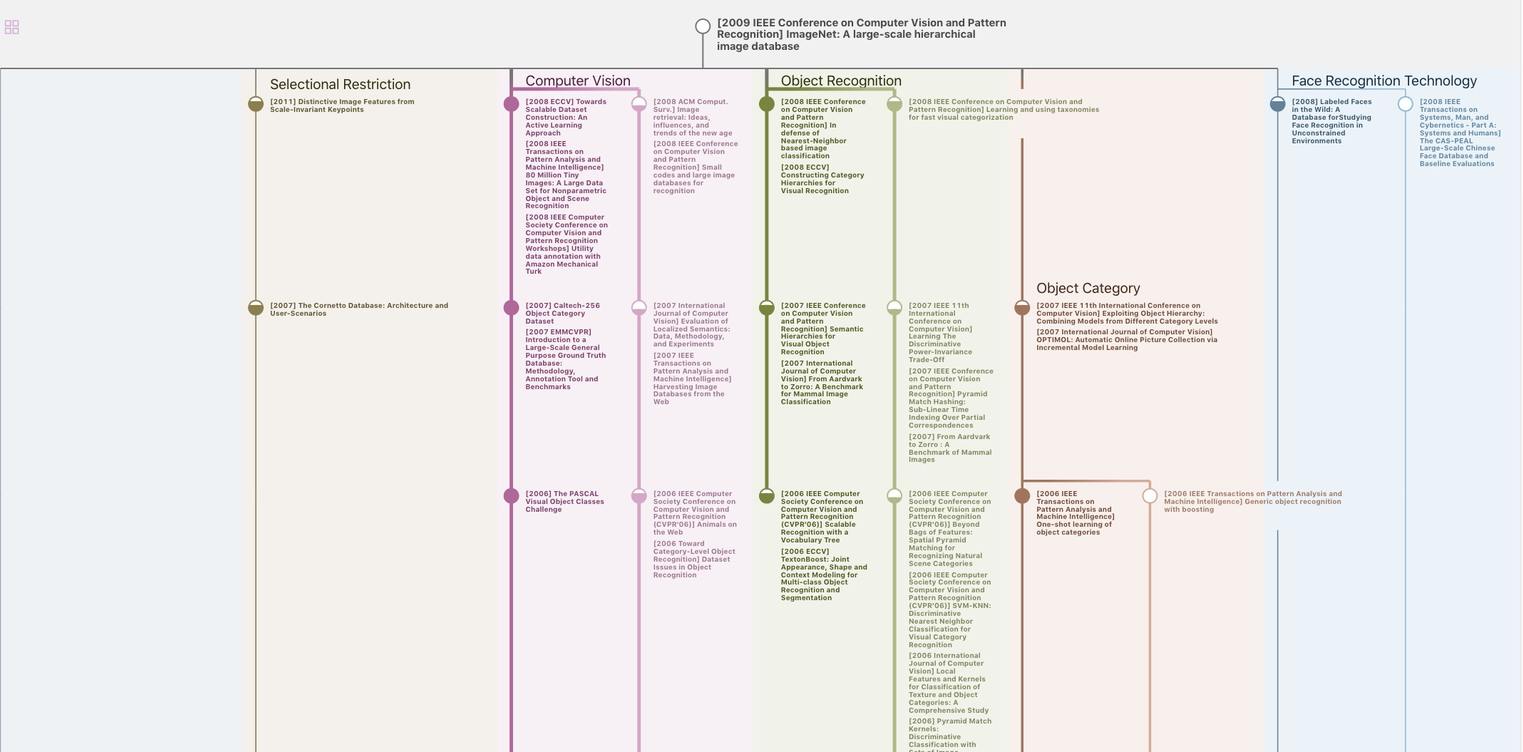
生成溯源树,研究论文发展脉络
Chat Paper
正在生成论文摘要