Data Anomaly Detection for Structural Health Monitoring Based on a Convolutional Neural Network
SENSORS(2023)
摘要
Structural health monitoring (SHM) has been extensively utilized in civil infrastructures for several decades. The status of civil constructions is monitored in real time using a wide variety of sensors; however, determining the true state of a structure can be difficult due to the presence of abnormalities in the acquired data. Extreme weather, faulty sensors, and structural damage are common causes of these abnormalities. For civil structure monitoring to be successful, abnormalities must be detected quickly. In addition, one form of abnormality generally predominates the SHM data, which might be a problem for civil infrastructure data. The current state of anomaly detection is severely hampered by this imbalance. Even cutting-edge damage diagnostic methods are useless without proper data-cleansing processes. In order to solve this problem, this study suggests a hyper-parameter-tuned convolutional neural network (CNN) for multiclass unbalanced anomaly detection. A multiclass time series of anomaly data from a real-world cable-stayed bridge is used to test the 1D CNN model, and the dataset is balanced by supplementing the data as necessary. An overall accuracy of 97.6% was achieved by balancing the database using data augmentation to enlarge the dataset, as shown in the research.
更多查看译文
关键词
CNN,data anomaly detection,time-series classification,structural health monitoring,sensor technology,deep learning
AI 理解论文
溯源树
样例
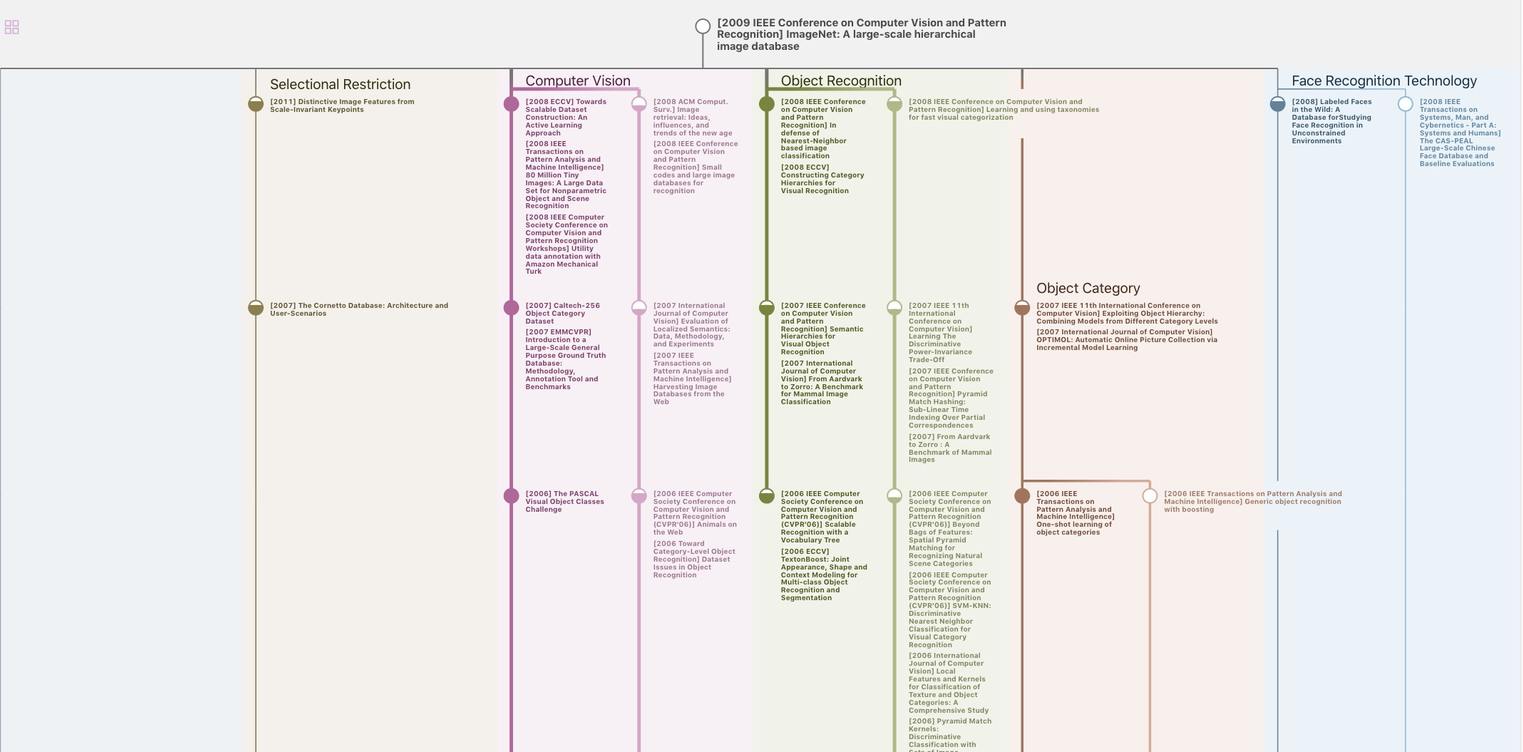
生成溯源树,研究论文发展脉络
Chat Paper
正在生成论文摘要