FEUSNet: Fourier Embedded U-Shaped Network for Image Denoising
Entropy (Basel, Switzerland)(2023)
摘要
Deep convolution neural networks have proven their powerful ability in comparing many tasks of computer vision due to their strong data learning capacity. In this paper, we propose a novel end-to-end denoising network, termed Fourier embedded U-shaped network (FEUSNet). By analyzing the amplitude spectrum and phase spectrum of Fourier coefficients, we find that low-frequency features of an image are in the former while noise features are in the latter. To make full use of this characteristic, Fourier features are learned and are concatenated as a prior module that is embedded into a U-shaped network to reduce noise while preserving multi-scale fine details. In the experiments, we first present ablation studies on the Fourier coefficients' learning networks and loss function. Then, we compare the proposed FEUSNet with the state-of-the-art denoising methods in quantization and qualification. The experimental results show that our FEUSNet performs well in noise suppression and preserves multi-scale enjoyable structures, even outperforming advanced denoising approaches.
更多查看译文
关键词
deep convolution neural network, end-to-end denoising network mechanism, Fourier coefficients
AI 理解论文
溯源树
样例
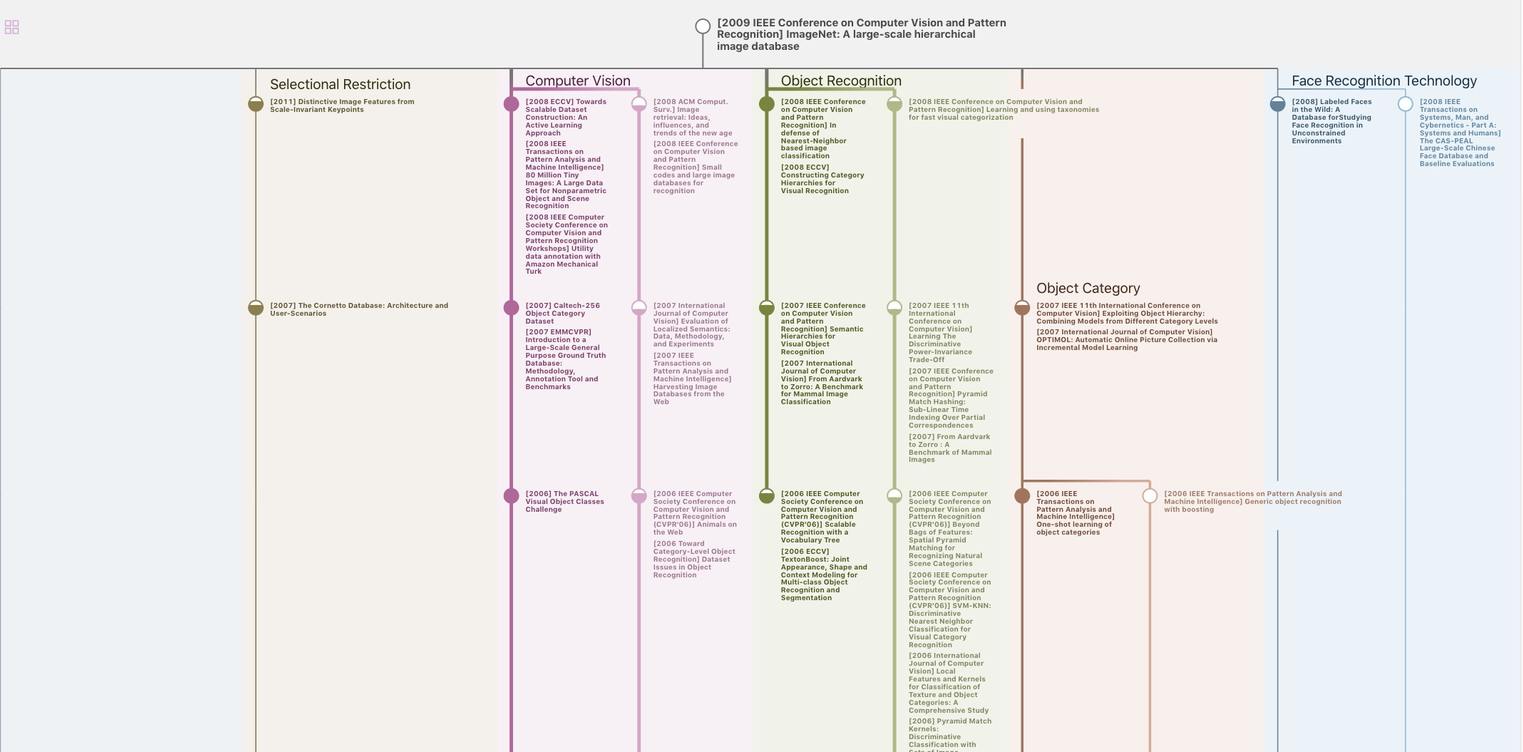
生成溯源树,研究论文发展脉络
Chat Paper
正在生成论文摘要