Exploratory Analysis of the Role of Radiomic Features in the Differentiation of Oncocytoma and Chromophobe RCC in the Nephrographic CT Phase
Life (Basel, Switzerland)(2023)
摘要
In diagnostic imaging, distinguishing chromophobe renal cell carcinomas (chRCCs) from renal oncocytomas (ROs) is challenging, since they both present similar radiological characteristics. Radiomics has the potential to help in the differentiation between chRCCs and ROs by extracting quantitative imaging. This is a preliminary study of the role of radiomic features in the differentiation of chRCCs and ROs using machine learning models. In this retrospective work, 38 subjects were involved: 19 diagnosed with chRCCs and 19 with ROs. The CT nephrographic contrast phase was selected in each case. Three-dimensional segmentations of the lesions were performed and the radiomic features were extracted. To assess the reliability of the features, the intraclass correlation coefficient was calculated from the segmentations performed by three radiologists with different degrees of expertise. The selection of features was based on the criteria of excellent intraclass correlation coefficient (ICC), high correlation, and statistical significance. Three machine learning models were elaborated: support vector machine (SVM), random forest (RF), and logistic regression (LR). From 105 extracted features, 41 presented an excellent ICC and 6 were not highly correlated with each other. Only two features showed significant differences according to histological type and machine learning models were developed with them. LR was the better model, in particular, with an 83% precision.
更多查看译文
关键词
diagnostic radiology,computed tomography,artificial intelligence,texture analysis,radiomics,renal cell carcinoma
AI 理解论文
溯源树
样例
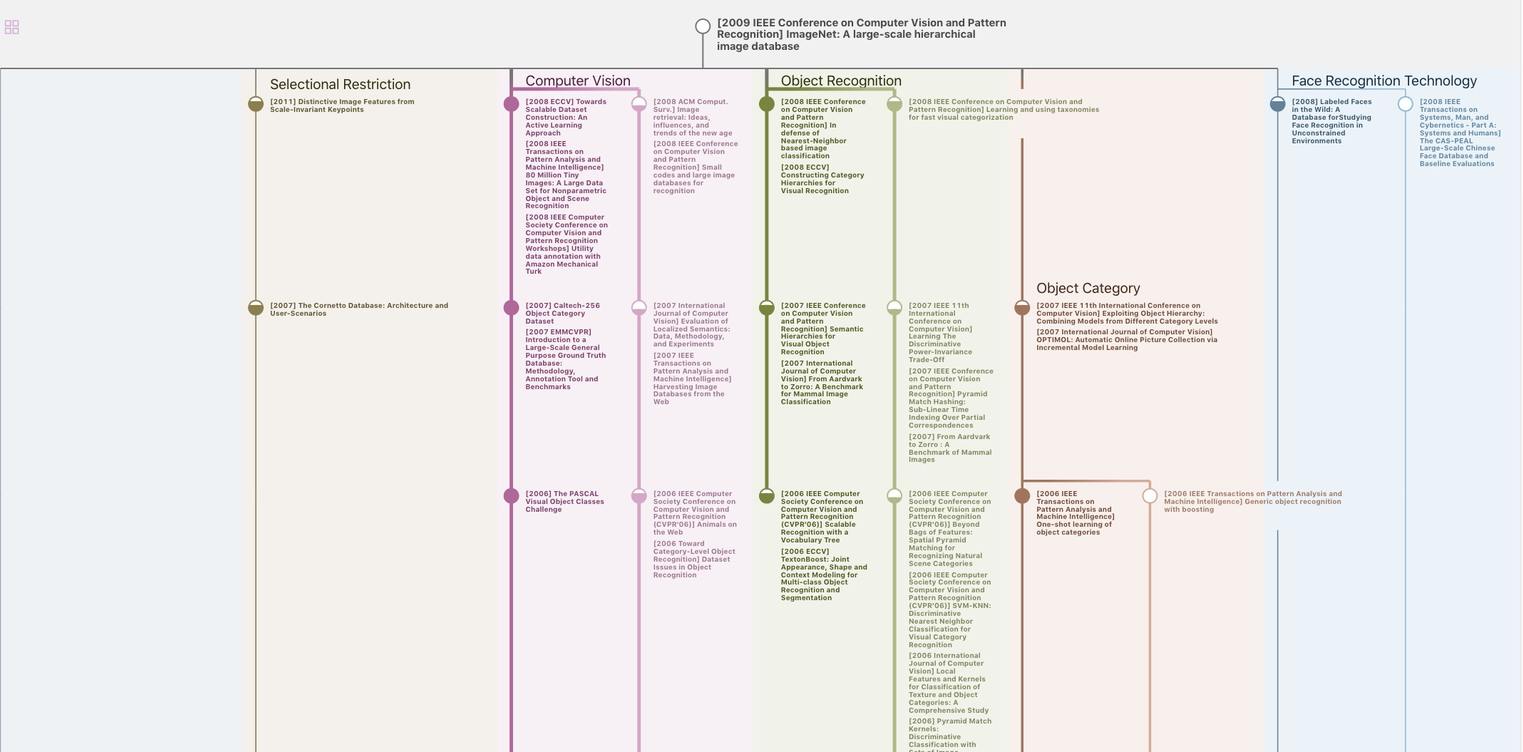
生成溯源树,研究论文发展脉络
Chat Paper
正在生成论文摘要