Sparsity-Constrained Kernel Generalized Maximum Versoria Criterion With Variable Center
IEEE Transactions on Circuits and Systems II: Express Briefs(2023)
摘要
Sparsity-aware kernel adaptive filtering algorithms using maximum correntropy criterion (MCC) and maximum Versoria criterion (MVC) have emerged as a promising candidate for nonlinear sparse parameter estimation under non-Gaussian distortions. However, the existing zero-attracting (ZA) kernel-MCC (ZA-KMCC) and ZA-kernel-MVC (ZA-KMVC) algorithms deliver suboptimal performance for non-zero-mean noise distributions due to the fixed center located at origin. In this brief, first we propose a novel generalized MVC with variable center (GMVC-VC) based learning paradigm by introducing a center at any location, which consists of higher order central moments of error. Next, we propose a novel generalized Versoria zero-attracting kernel GMVC-VC (GVZA-KGMVC-VC) algorithm, which is robust for nonlinear sparse systems under non-Gaussian noise having non-zero-mean. Furthermore, the detailed convergence analysis in both mean and mean-square sense is performed for the proposed algorithm. Simulations demonstrate that the proposed GVZA-KGMVC-VC approach delivers lower mean square deviation over the existing state-of-art benchmarks.
更多查看译文
关键词
Sparsity-aware,maximum Versoria criterion,zero-attracting,reproducing kernel Hilbert space,nonlinear,sparse parameter estimation,non-Gaussian,non-zero-mean
AI 理解论文
溯源树
样例
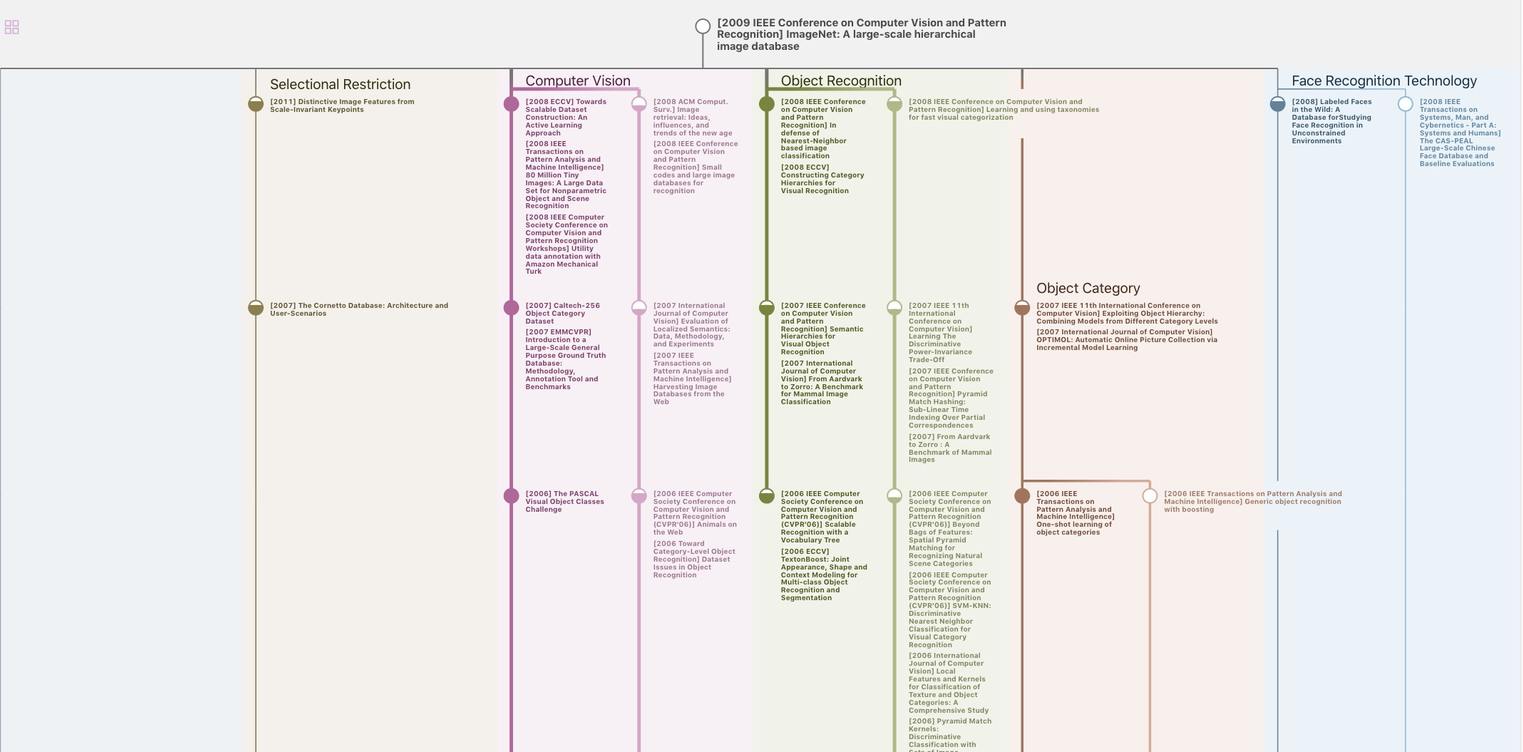
生成溯源树,研究论文发展脉络
Chat Paper
正在生成论文摘要