Achalasia phenotypes and prediction of peroral endoscopic myotomy outcomes using machine learning
DIGESTIVE ENDOSCOPY(2023)
摘要
ObjectivesHigh-resolution manometry (HRM) and esophagography are used for achalasia diagnosis; however, achalasia phenotypes combining esophageal motility and morphology are unknown. Moreover, predicting treatment outcomes of peroral endoscopic myotomy (POEM) in treatment-naive patients remains an unmet need.MethodsIn this multicenter cohort study, we included 1824 treatment-naive patients diagnosed with achalasia. In total, 1778 patients underwent POEM. Clustering by machine learning was conducted to identify achalasia phenotypes using patients' demographic data, including age, sex, disease duration, body mass index, and HRM/esophagography findings. Machine learning models were developed to predict persistent symptoms (Eckardt score >= 3) and reflux esophagitis (RE) (Los Angeles grades A-D) after POEM.ResultsMachine learning identified three achalasia phenotypes: phenotype 1, type I achalasia with a dilated esophagus (n = 676; 37.0%); phenotype 2, type II achalasia with a dilated esophagus (n = 203; 11.1%); and phenotype 3, late-onset type I-III achalasia with a nondilated esophagus (n = 619, 33.9%). Types I and II achalasia in phenotypes 1 and 2 exhibited different clinical characteristics from those in phenotype 3, implying different pathophysiologies within the same HRM diagnosis. A predictive model for persistent symptoms exhibited an area under the curve of 0.70. Pre-POEM Eckardt score >= 6 was the greatest contributing factor for persistent symptoms. The area under the curve for post-POEM RE was 0.61.ConclusionAchalasia phenotypes combining esophageal motility and morphology indicated multiple disease pathophysiologies. Machine learning helped develop an optimal risk stratification model for persistent symptoms with novel insights into treatment resistance factors.
更多查看译文
关键词
achalasia,machine learning,peroral endoscopic myotomy
AI 理解论文
溯源树
样例
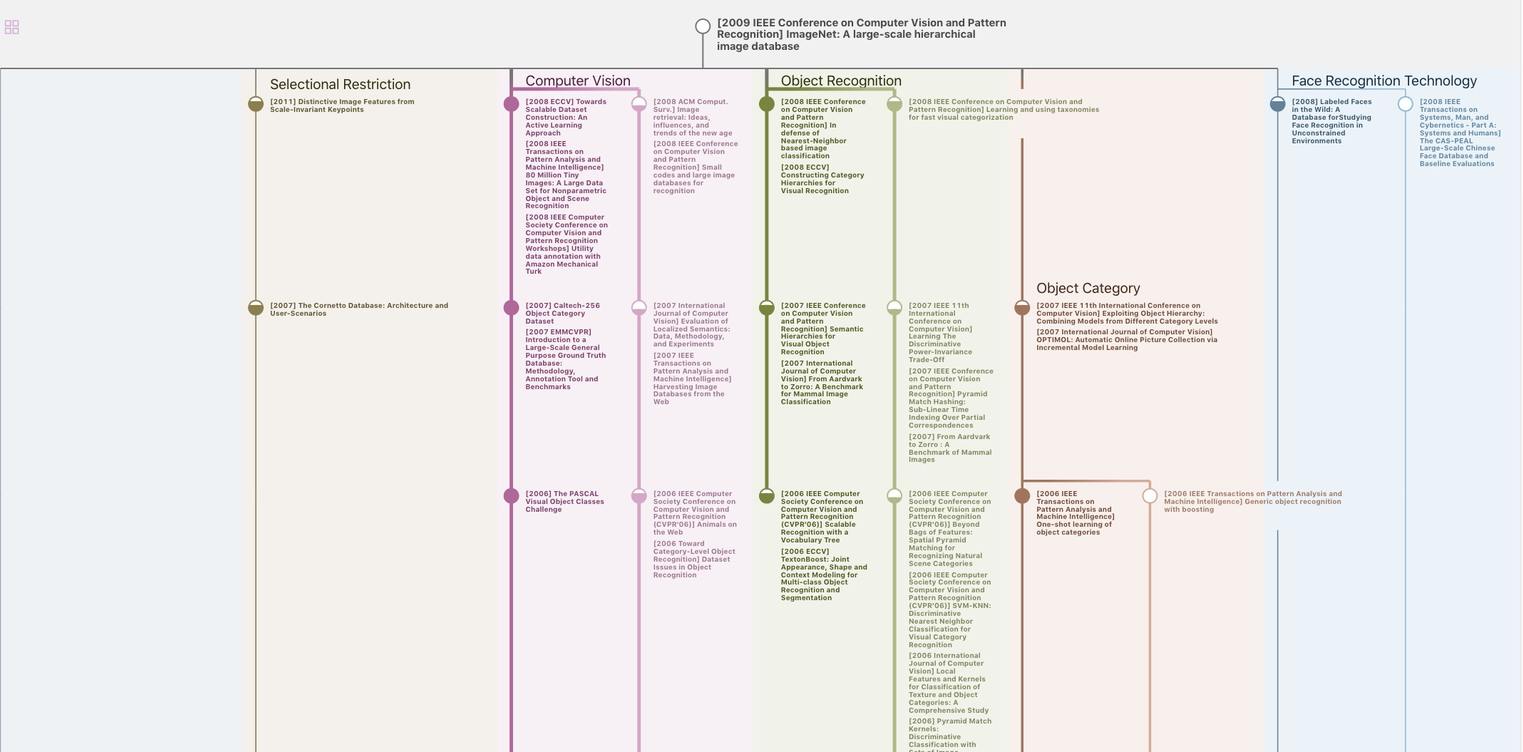
生成溯源树,研究论文发展脉络
Chat Paper
正在生成论文摘要