Stochastic interpretable machine learning based multiscale modeling in thermal conductivity of Polymeric graphene-enhanced composites
COMPOSITE STRUCTURES(2024)
摘要
We introduce an interpretable stochastic integrated machine learning based multiscale approach for the prediction of the macroscopic thermal conductivity in Polymeric graphene-enhanced composites (PGECs). This method encompasses the propagation of uncertain input parameters from the meso to macro scale, implemented through a foundational bottom-up multi-scale framework. In this context, Representative Vol-ume Elements in Finite Element Modeling (RVE-FEM) are employed to derive the homogenized thermal conductivity. Besides, we employ two sets of techniques: Regression-tree-based methods (Random Forest and Gradient Boosting Machine) and Neural networks-based approaches (Artificial Neural Networks and Deep Neural Networks). To ascertain the relative influence of factors on output estimations, the SHapley Additive exPlanations (SHAP) algorithm is integrated. This interpretable machine learning methodology demonstrates strong alignment with published experimental data. It holds promise as an efficient and versatile tool for designing new composite materials tailored to applications involving thermal management.
更多查看译文
关键词
Polymeric graphene-enhanced composites,(PGECs),Interpretable Integrated Learning,Stochastic multi-scale modeling,Thermal properties,Data-driven technique
AI 理解论文
溯源树
样例
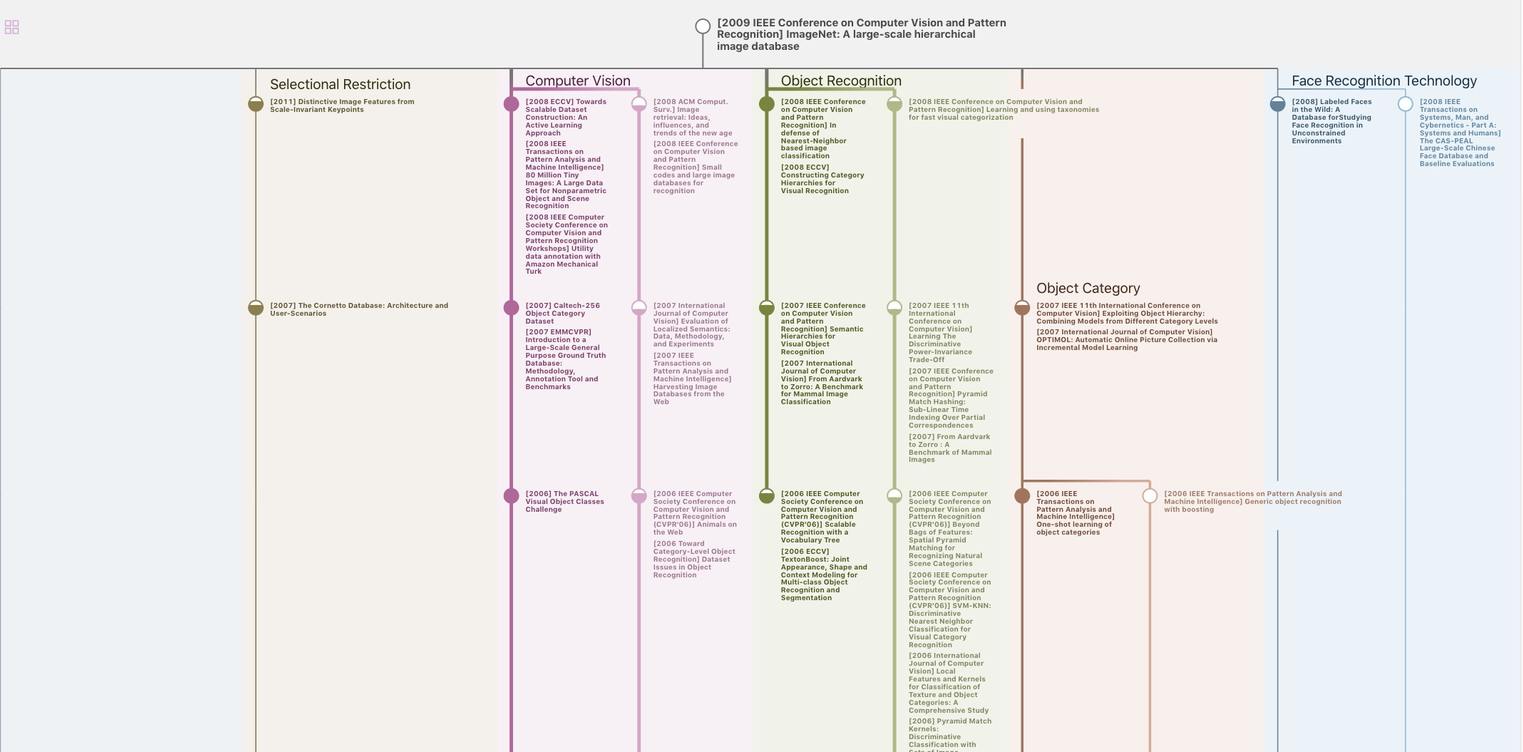
生成溯源树,研究论文发展脉络
Chat Paper
正在生成论文摘要