Multi-CC: A New Baseline for Faster and Better Deep Clustering
Electronics(2023)
摘要
The aim of our paper is to introduce a new deep clustering model called Multi-head Cross-Attention Contrastive Clustering (Multi-CC), which seeks to enhance the performance of the existing deep clustering model CC. Our approach involves first augmenting the data to form image pairs and then using the same backbone to extract the feature representation of these image pairs. We then undertake contrastive learning, separately in the row space and column space of the feature matrix, to jointly learn the instance and cluster representations. Our approach offers several key improvements over the existing model. Firstly, we use a mixed strategy of strong and weak augmentation to construct image pairs. Secondly, we get rid of the pooling layer of the backbone to prevent loss of information. Finally, we introduce a multi-head cross-attention module to improve the model's performance. These improvements have allowed us to reduce the model training time by 80%. As a baseline, Multi-CC achieves the best results on CIFAR-10, ImageNet-10, and ImageNet-dogs. It is easily replaceable with CC, making models based on CC achieve better performance.
更多查看译文
关键词
clustering,deep clustering,contrastive learning
AI 理解论文
溯源树
样例
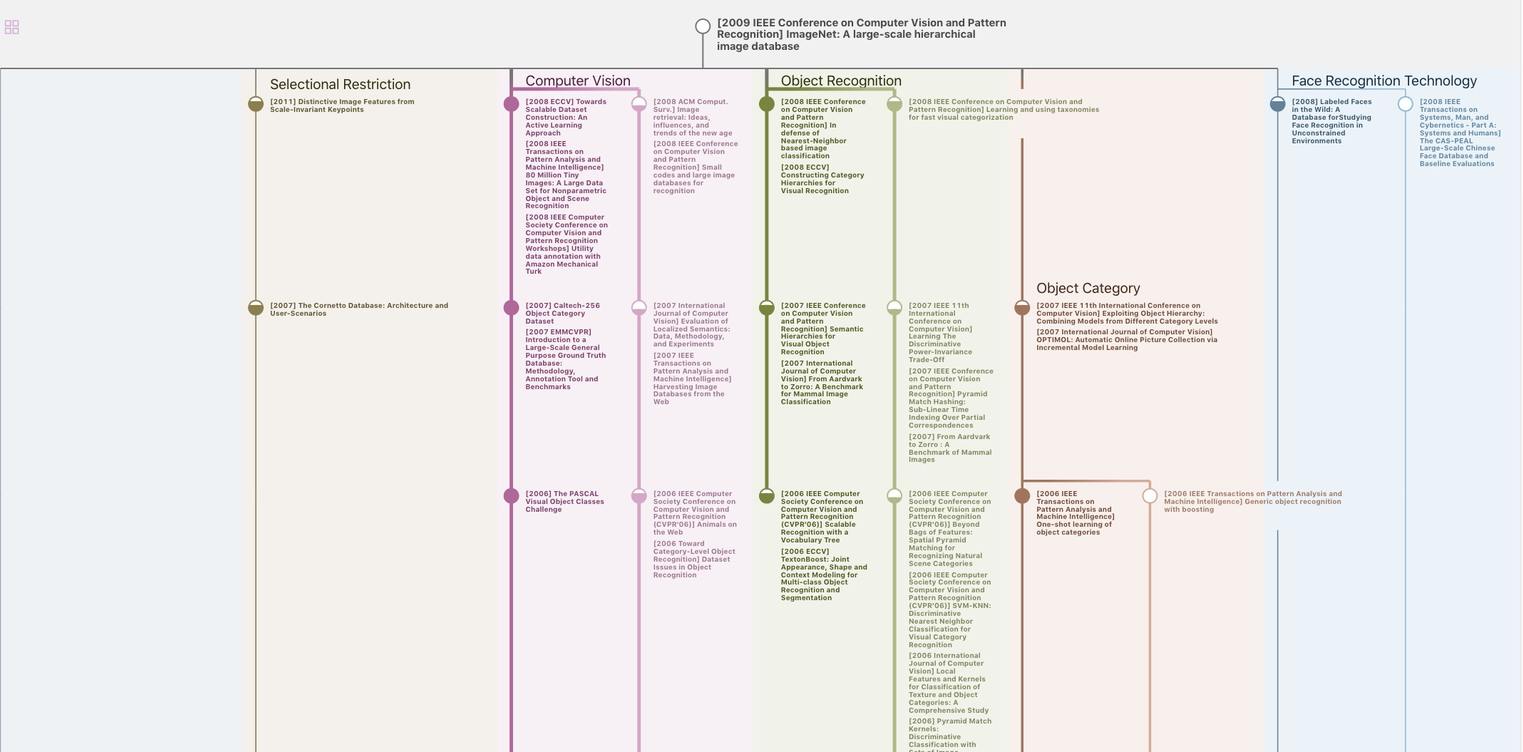
生成溯源树,研究论文发展脉络
Chat Paper
正在生成论文摘要