A Multiscale Neighbor-Aware Attention Network for Collaborative Filtering
Electronics(2023)
摘要
Most recommender systems rely on user and item attributes or their interaction records to find similar neighbors for collaborative filtering. Existing methods focus on developing collaborative signals from only one type of neighbors and ignore the unique contributions of different types of neighbor views. This paper proposes a multiscale neighbor-aware attention network for collaborative filtering (MSNAN). First, attribute-view neighbor embedding is modeled to extract the features of different types of neighbors with co-occurrence attributes, and interaction-view neighbor embedding is leveraged to describe the fine-grained neighborhood behaviors of ratings. Then, a matched attention network is used to identify different contributions of multiscale neighbors and capture multiple types of collaborative signals for overcoming sparse recommendations. Finally, we make the rating prediction by a joint learning of multi-task loss and verify the positive effect of the proposed MSNAN on three datasets. Compared with traditional methods, the experimental results of the proposed MSNAN not only improve the accuracy in MAE and RMSE indexes, but also solve the problem of poor performance for recommendation in sparse data scenarios.
更多查看译文
关键词
multiscale neighbors,attentional mechanism,collaborative embedding,recommendation
AI 理解论文
溯源树
样例
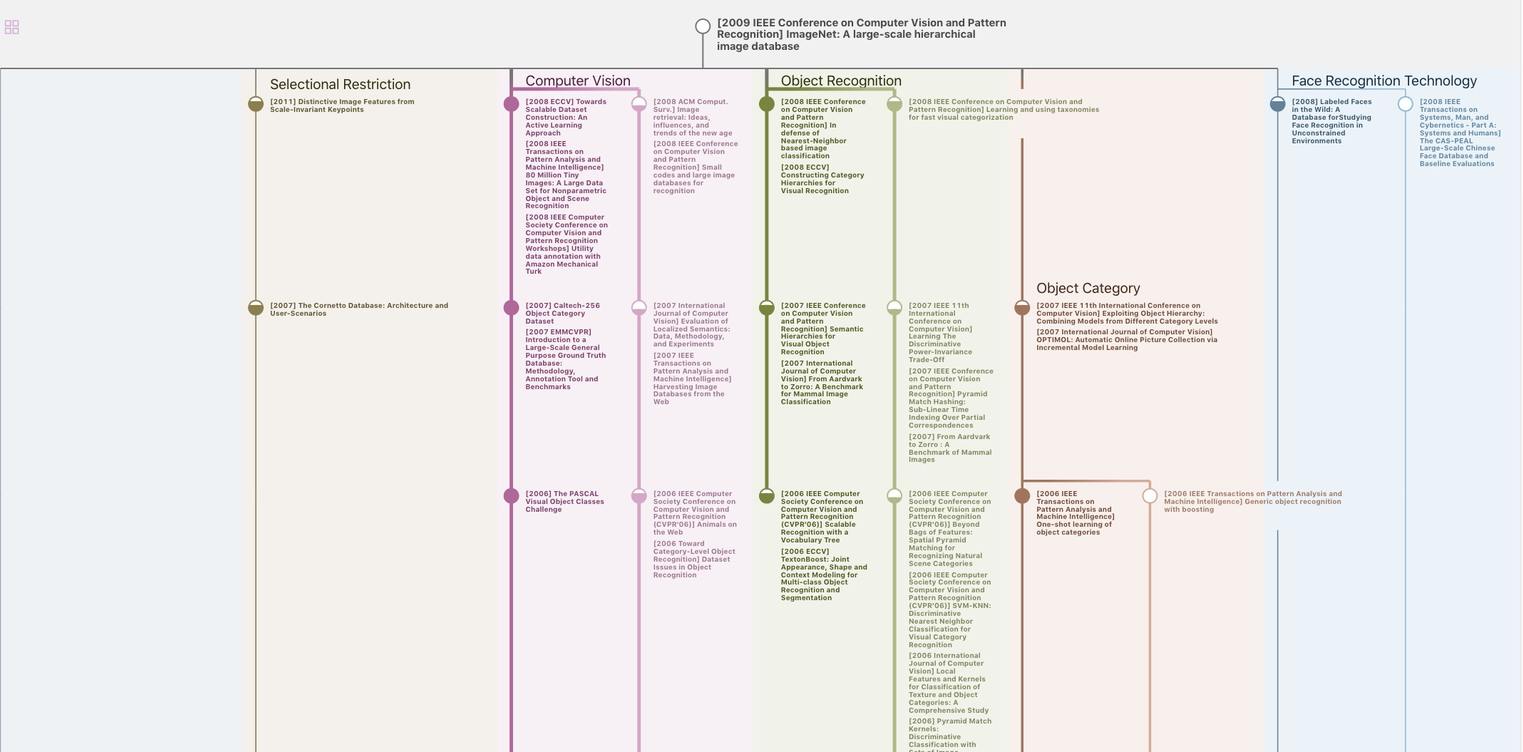
生成溯源树,研究论文发展脉络
Chat Paper
正在生成论文摘要